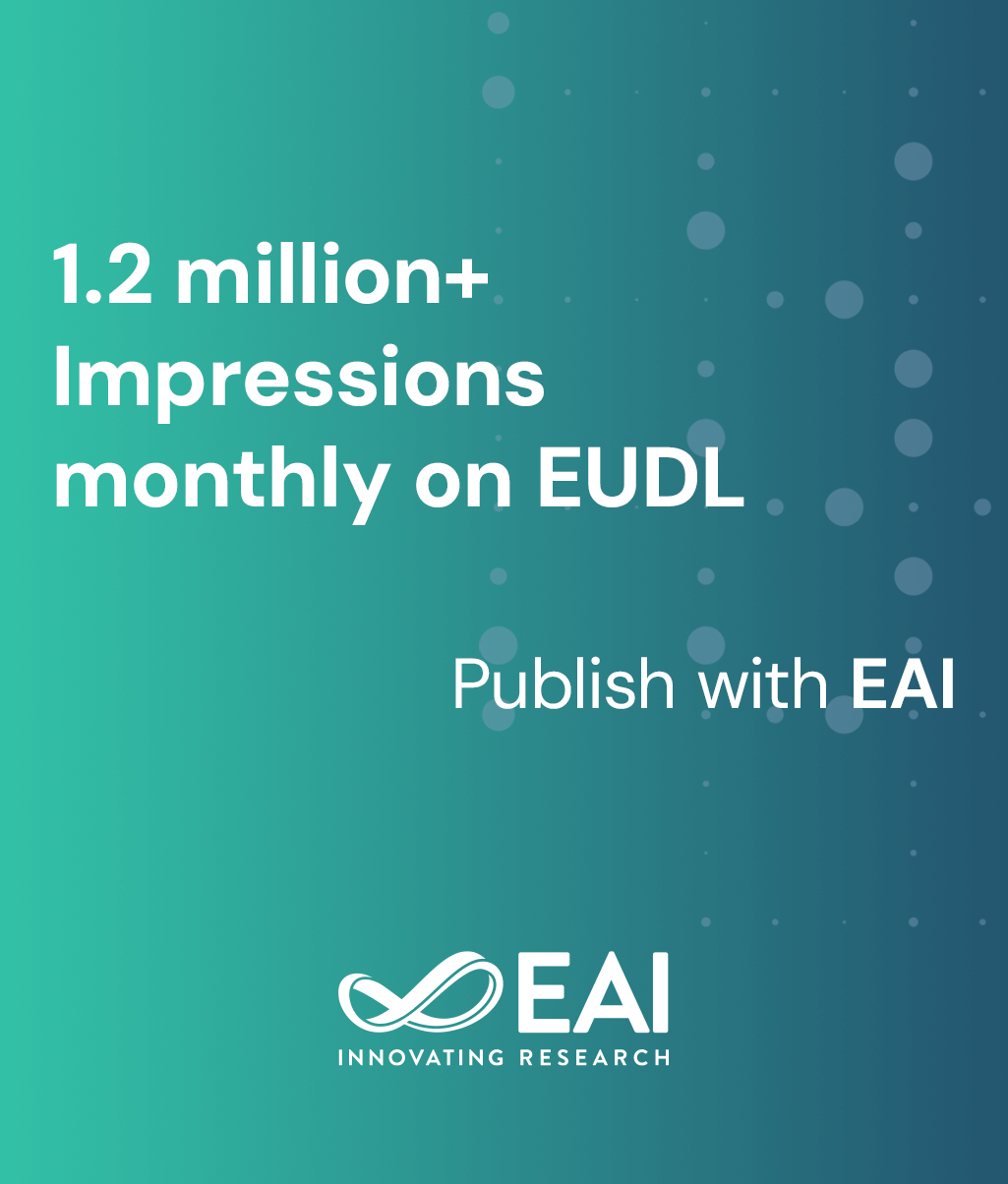
Research Article
Vehicle Re-identification Using Joint Pyramid Feature Representation Network
@INPROCEEDINGS{10.1007/978-3-030-44751-9_44, author={Xiangwei Lin and Huanqiang Zeng and Jinhui Hou and Jianqing Zhu and Jing Chen and Kai-Kuang Ma}, title={Vehicle Re-identification Using Joint Pyramid Feature Representation Network}, proceedings={IoT as a Service. 5th EAI International Conference, IoTaaS 2019, Xi’an, China, November 16-17, 2019, Proceedings}, proceedings_a={IOTAAS}, year={2020}, month={6}, keywords={Vehicle re-identification Joint pyramid feature representation Deep learning}, doi={10.1007/978-3-030-44751-9_44} }
- Xiangwei Lin
Huanqiang Zeng
Jinhui Hou
Jianqing Zhu
Jing Chen
Kai-Kuang Ma
Year: 2020
Vehicle Re-identification Using Joint Pyramid Feature Representation Network
IOTAAS
Springer
DOI: 10.1007/978-3-030-44751-9_44
Abstract
Vehicle re-identification (Re-ID) technology plays an important role in intelligent video surveillance systems. Due to various factors, e.g., resolution variation, viewpoint variation, illumination changes, occlusion, etc., vehicle Re-ID is a very challenging computer vision task. In order to solve this problem, a joint pyramid feature representation network (JPFRN) is proposed in this paper. Based on the consideration that various convolution blocks with different depths hold various resolution and semantic information of the vehicle image, which can help to effectively identify the vehicle, the proposed JPFRN method obtains four vehicle feature blocks with different depths by designing pyramidal feature fusion of each convolution block in a basic network. After that, a joint representation of these pyramidal features is feed into the loss function for learning discriminative features for vehicle Re-ID. We validated the proposed approach on a commonly used vehicle database i.e., VehicleID. Extensive experimental results show that the proposed method is superior to multiple state-of-the-art vehicle Re-ID methods.