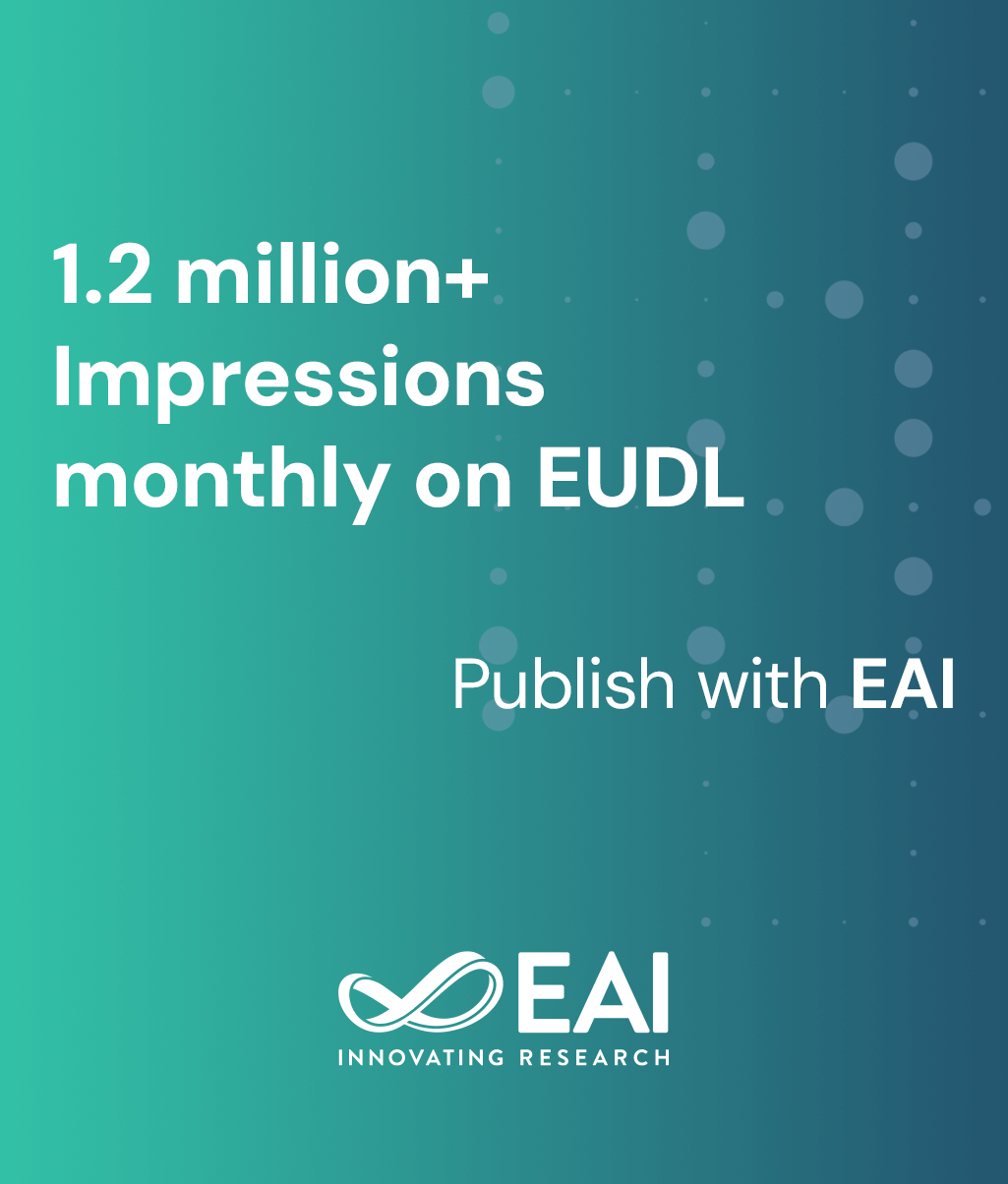
Research Article
Vehicle Feature Point Trajectory Clustering and Vehicle Behavior Analysis in Complex Traffic Scenes
@INPROCEEDINGS{10.1007/978-3-030-44751-9_17, author={Xuan Wang and Jindong Zhao and Yingjie Wang and Jun Lv and Weiqing Yan}, title={Vehicle Feature Point Trajectory Clustering and Vehicle Behavior Analysis in Complex Traffic Scenes}, proceedings={IoT as a Service. 5th EAI International Conference, IoTaaS 2019, Xi’an, China, November 16-17, 2019, Proceedings}, proceedings_a={IOTAAS}, year={2020}, month={6}, keywords={Vehicle segmentation Feature point detection Trajectory clustering Behavior analysis}, doi={10.1007/978-3-030-44751-9_17} }
- Xuan Wang
Jindong Zhao
Yingjie Wang
Jun Lv
Weiqing Yan
Year: 2020
Vehicle Feature Point Trajectory Clustering and Vehicle Behavior Analysis in Complex Traffic Scenes
IOTAAS
Springer
DOI: 10.1007/978-3-030-44751-9_17
Abstract
Video-based analysis technology has a wide range of applications in intelligent transportation system (ITS). Vehicle segmentation and behavior analysis has become an important research area in traffic video analysis. To solve the problem of 2D video detection technology in actual traffic video scenes, a bottom-up analysis method is employed to study the related technical problems. Firstly, M-BRISK descriptor algorithm is proposed for describing local feature points, which based on the method of original BRISK. Secondly, a 3D feature analysis method based on rigid motion constraints for vehicle trajectory is proposed. With the result of camera calibration and the preset back-projection plane, the 2D trajectory points can be back-projected to the 3D space, and the back projection data of the 2D image can be reconstructed in 3D space. Thirdly, similarity measure method is proposed for achieving the trajectory clustering. The experimental results show that the proposed method not only accelerates the speed of clustering method, but also improves the accuracy of trajectory clustering at some extent. Moreover, the vehicle motion information contained in the trajectory data can be analyzed to recognize vehicle behavior. All of these provide an important data foundation for vehicle abnormal behavior detection and the identification of traffic status levels in traffic scenes.