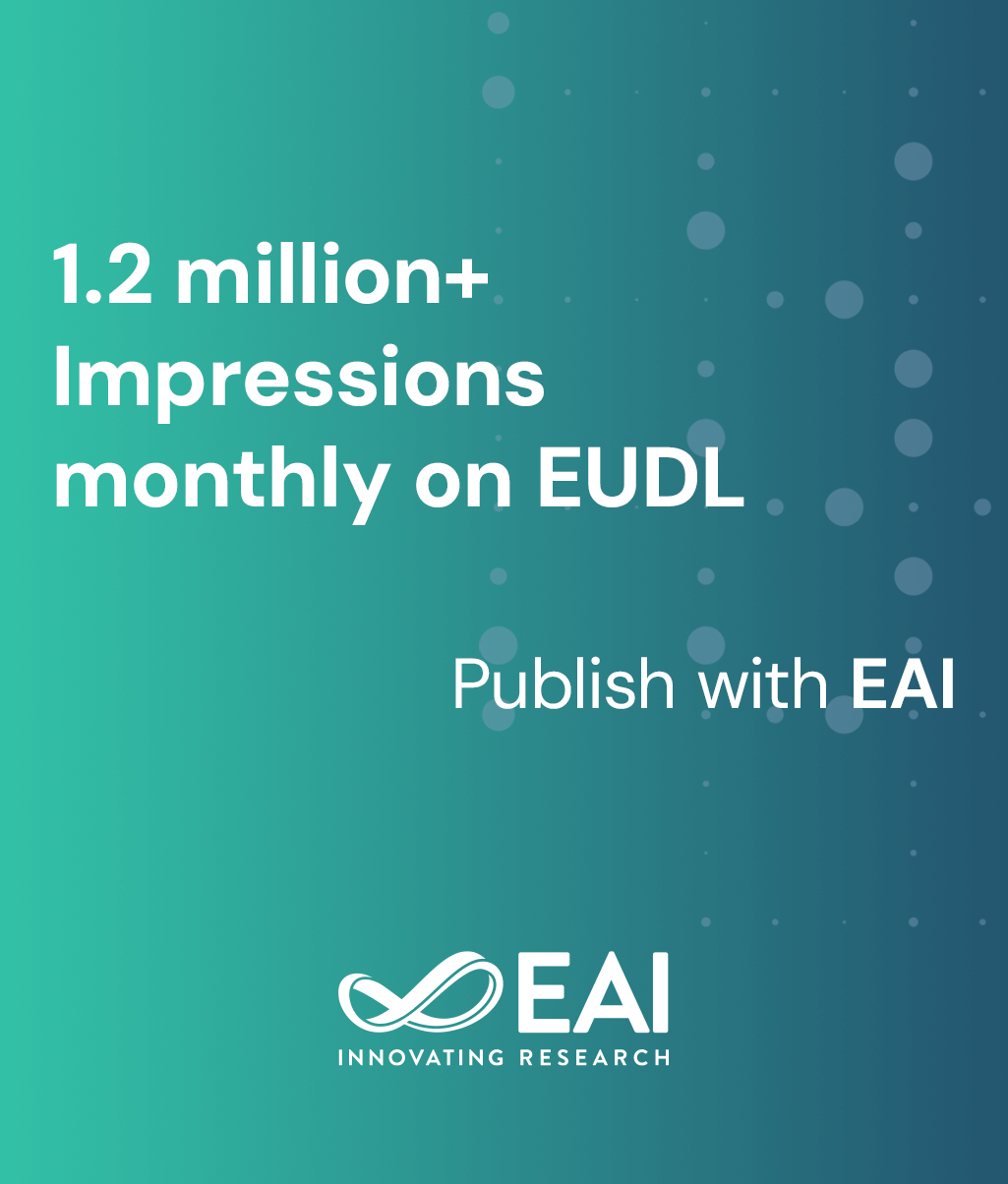
Research Article
Task Migration Using Q-Learning Network Selection for Edge Computing in Heterogeneous Wireless Networks
@INPROCEEDINGS{10.1007/978-3-030-44751-9_13, author={Yi Liu and Jie Zheng and Jie Ren and Ling Gao and Hai Wang}, title={Task Migration Using Q-Learning Network Selection for Edge Computing in Heterogeneous Wireless Networks}, proceedings={IoT as a Service. 5th EAI International Conference, IoTaaS 2019, Xi’an, China, November 16-17, 2019, Proceedings}, proceedings_a={IOTAAS}, year={2020}, month={6}, keywords={Edge computing Heterogeneous wireless networks Network selection Task migration Q-learning}, doi={10.1007/978-3-030-44751-9_13} }
- Yi Liu
Jie Zheng
Jie Ren
Ling Gao
Hai Wang
Year: 2020
Task Migration Using Q-Learning Network Selection for Edge Computing in Heterogeneous Wireless Networks
IOTAAS
Springer
DOI: 10.1007/978-3-030-44751-9_13
Abstract
For edge devices, pushing the task to other near devices has become a widely concerned service provision paradigm. However, the energy-constrained nature of edge devices makes optimizing for Quality of Service (QoS) difficult. We choose three factors as QoS: the delay limitation, the CPU usage of terminal and energy consumption. Due to the delay limitation of different tasks for edge computing and the different rates in heterogeneous wireless networks, we propose a network selection task migration algorithm based on Q-learning that captures the trade-off between QoS and energy consumption. Our approach can automatically choose a suitable network to perform task migration reasons about the task’s QoS requirements and computing rate in 4G network, Wi-Fi, Device-to-device (D2D). We demonstrate a working prototype using the YOLOv3 on the Vivo X9 devices. Based on real hardware and software measurements, we achieve 27.79% energy saving and 35% reduction in delay.