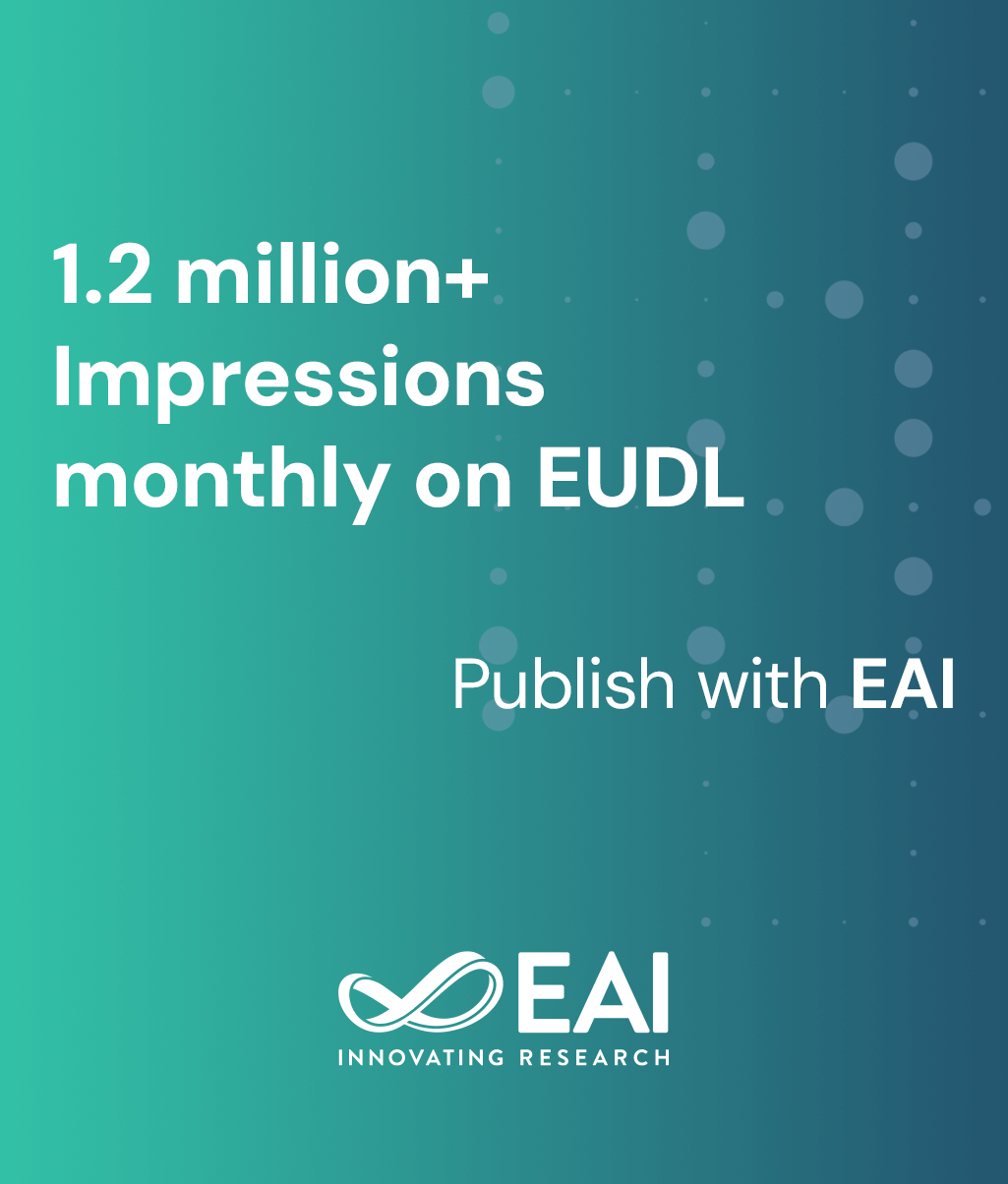
Research Article
Ethiopic Natural Scene Text Recognition Using Deep Learning Approaches
@INPROCEEDINGS{10.1007/978-3-030-43690-2_36, author={Direselign Addis and Chuan-Ming Liu and Van-Dai Ta}, title={Ethiopic Natural Scene Text Recognition Using Deep Learning Approaches}, proceedings={Advances of Science and Technology. 7th EAI International Conference, ICAST 2019, Bahir Dar, Ethiopia, August 2--4, 2019, Proceedings}, proceedings_a={ICAST}, year={2020}, month={6}, keywords={Scene text recognition Deep learning Ethiopic script}, doi={10.1007/978-3-030-43690-2_36} }
- Direselign Addis
Chuan-Ming Liu
Van-Dai Ta
Year: 2020
Ethiopic Natural Scene Text Recognition Using Deep Learning Approaches
ICAST
Springer
DOI: 10.1007/978-3-030-43690-2_36
Abstract
The success of deep learning approaches for scene text recognition in English, Chinese and Arabic language inspired us to pose a benchmark scene text recognition for Ethiopic script. To transcribe the word images to the cross bonding text, we use a segmentation free end-to-end trainable Convolutional and Recurrent Neural Network (CRNN) hybrid architecture. In the network, robust representation features from cropped word images are extracted at convolutional layer and the extracted representations features are transcribed to a sequence of labels by the recurrent layer and transcription layer. The transcription is not bounded by lexicon or word length. Due to it is effective uses to transcribe sequence-to-sequence tasks, CTC loss is applied to train the network. In order to train the proposed model, we prepare synthetic word images from Unicode fonts of Ethiopic scripts, besides the model performance is evaluated on real scene text dataset collected from different sources. The experiment result of the proposed model, shows a promising result.