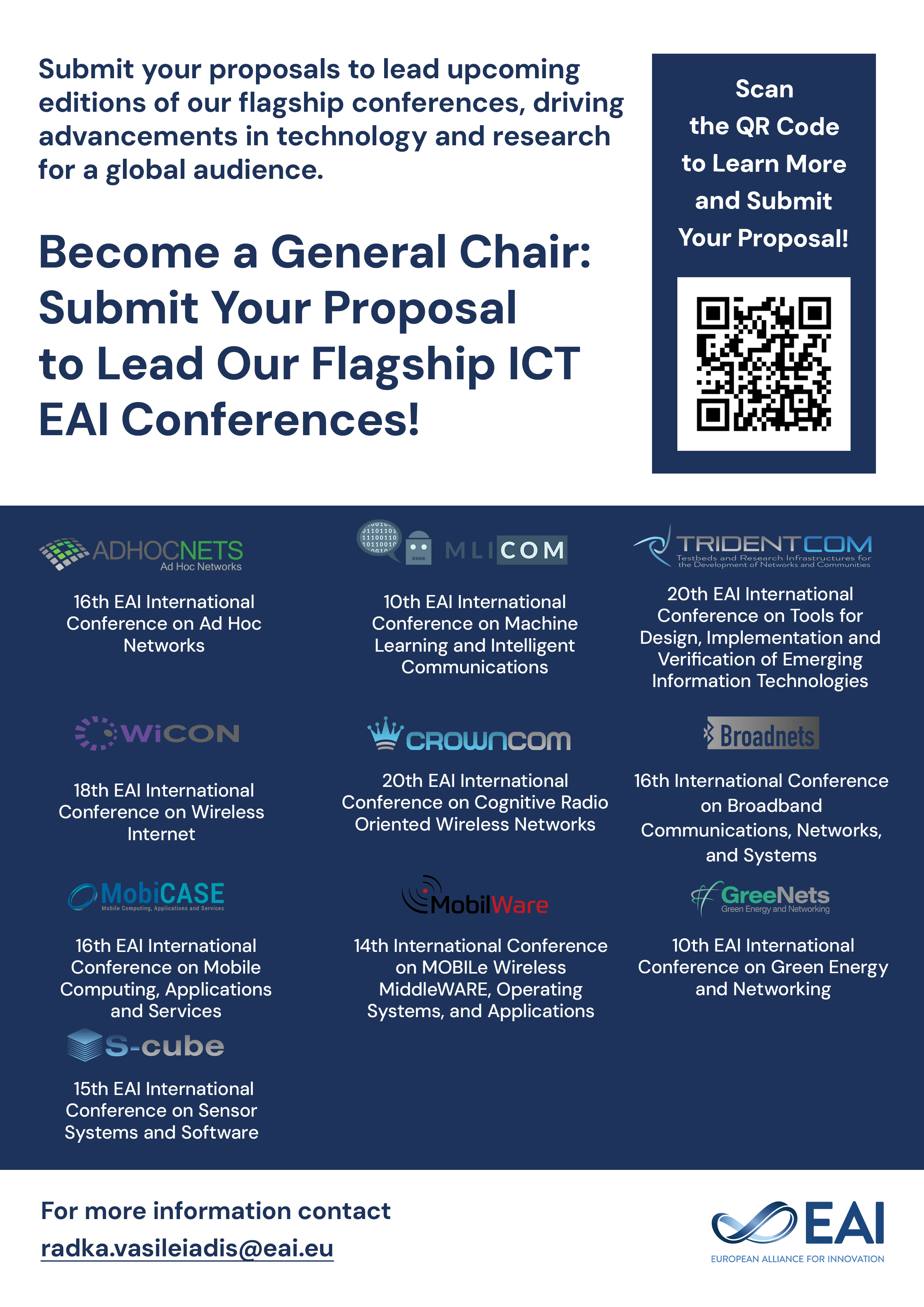
Research Article
Mobility Prediction in Wireless Networks Using Deep Learning Algorithm
@INPROCEEDINGS{10.1007/978-3-030-43690-2_31, author={Abebe Adege and Hsin-Piao Lin and Getaneh Tarekegn and Yirga Yayeh}, title={Mobility Prediction in Wireless Networks Using Deep Learning Algorithm}, proceedings={Advances of Science and Technology. 7th EAI International Conference, ICAST 2019, Bahir Dar, Ethiopia, August 2--4, 2019, Proceedings}, proceedings_a={ICAST}, year={2020}, month={6}, keywords={Long-short term memory Location based services Mobility prediction}, doi={10.1007/978-3-030-43690-2_31} }
- Abebe Adege
Hsin-Piao Lin
Getaneh Tarekegn
Yirga Yayeh
Year: 2020
Mobility Prediction in Wireless Networks Using Deep Learning Algorithm
ICAST
Springer
DOI: 10.1007/978-3-030-43690-2_31
Abstract
Recently, wireless-technologies and their users are rising due to productions of sensor-networks, mobile devices, and supporting applications. Location Based Services (LBS) such as mobility prediction is a key technology for the success of IoT. However, mobility prediction in wireless network is too challenging since the network becomes very condensed and it changes dynamically. In this paper, we propose a deep neural network based mobility prediction in wireless environment to provide an adaptive and accurate positioning system to mobile users. In the system development processes, firstly, we collect RSS values from three Unmanned Aerial Vehicle Base Stations (UAV-BSs). Secondly, we preprocess the collected data to get refine features and to avoid null records or cells. Thirdly, we exhaustively train the Long-short term memory (LSTM) network to find the optimum model for mobility prediction of the smartphone users. Finally, we test the designed model to evaluate system performances. The performance of the proposed system also compared with Multilayer Perceptron (MLP) algorithm to assess the soundness of mobility prediction model. The LSTM outperforms the MLP algorithm in different evaluating parameters.