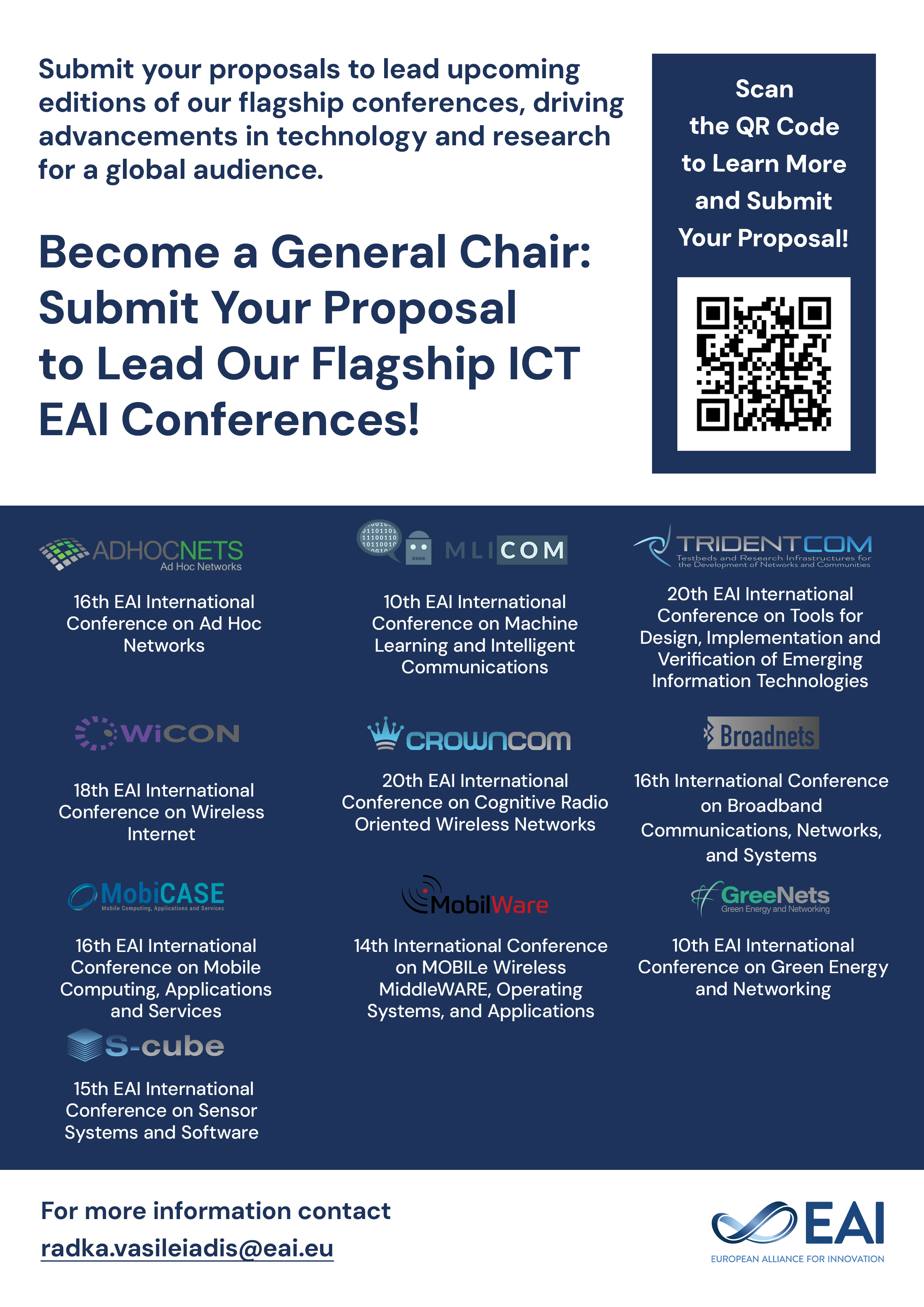
Research Article
A Link Analysis Based Approach to Predict Character Death in Game of Thrones
@INPROCEEDINGS{10.1007/978-3-030-43215-7_16, author={Swati Agarwal and Rahul Thakur and Sudeepta Mishra}, title={A Link Analysis Based Approach to Predict Character Death in Game of Thrones}, proceedings={Testbeds and Research Infrastructures for the Development of Networks and Communications. 14th EAI International Conference, TridentCom 2019, Changsha, China, December 7-8, 2019, Proceedings}, proceedings_a={TRIDENTCOM}, year={2020}, month={3}, keywords={Character similarity Death prediction Feature importance Game of Thrones Social network analysis Weighted vector space model}, doi={10.1007/978-3-030-43215-7_16} }
- Swati Agarwal
Rahul Thakur
Sudeepta Mishra
Year: 2020
A Link Analysis Based Approach to Predict Character Death in Game of Thrones
TRIDENTCOM
Springer
DOI: 10.1007/978-3-030-43215-7_16
Abstract
Mysterious and uncertain deaths in the “Game of Thrones” novel-series have been stupefying to the vast pool of readers and hence interested researchers to come up with various models to predict the deaths. In this paper, we propose a Death-Prone Score model to predict if the candidate character is going to die or stay alive in the upcoming book in the series. We address the challenge of high-dimensional data and train our model on the most significant attributes by computing feature importance in the vector space. Further, we address the challenge of multiple interactions between characters and create a social network representing the weighted similarity between each character pair in the book. The proposed model takes similarity and proximity in a social network into account and generates a death-prone score for each character. To evaluate our model, we divide the characters data into training (characters died before year 300) and testing (characters died in the year 300 and characters alive till year 300). Our results show that the proposed Death-Prone Score model achieves an f-score of 86.2%.