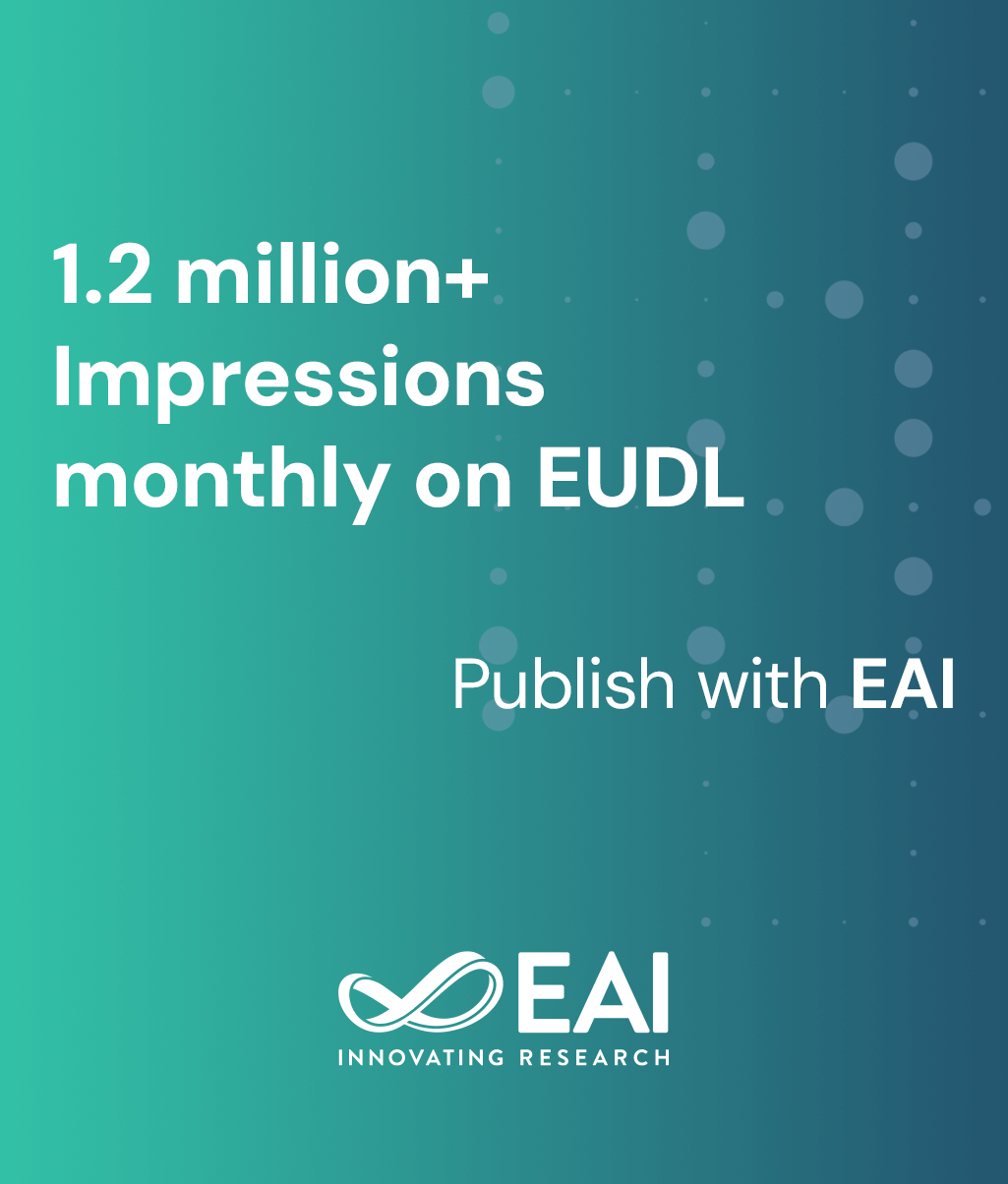
Research Article
Activity Recognition and Classification via Deep Neural Networks
@INPROCEEDINGS{10.1007/978-3-030-43215-7_15, author={Zhi Wang and Liangliang Lin and Ruimeng Wang and Boyang Wei and Yueshen Xu and Zhiping Jiang and Rui Li}, title={Activity Recognition and Classification via Deep Neural Networks}, proceedings={Testbeds and Research Infrastructures for the Development of Networks and Communications. 14th EAI International Conference, TridentCom 2019, Changsha, China, December 7-8, 2019, Proceedings}, proceedings_a={TRIDENTCOM}, year={2020}, month={3}, keywords={Channel state information Pearson correlation coefficient Deep convolutional neural networks AlexNet network}, doi={10.1007/978-3-030-43215-7_15} }
- Zhi Wang
Liangliang Lin
Ruimeng Wang
Boyang Wei
Yueshen Xu
Zhiping Jiang
Rui Li
Year: 2020
Activity Recognition and Classification via Deep Neural Networks
TRIDENTCOM
Springer
DOI: 10.1007/978-3-030-43215-7_15
Abstract
Based on the Wi-Fi widely separated in the world, Wi-Fi-based wireless activity recognition has attracted more and more research efforts. Now, device-based activity awareness is being used for commercial purpose as the most important solution. Such devices based on various acceleration sensors and direction sensor are very mature at present. With more and more profound understanding of wireless signals, commercial wireless routers are used to obtain signal information of the physical layer: channel state information (CSI) more granular than the RSSI signal information provides a theoretical basis for wireless signal perception. Through research on activity recognition techniques based on CSI of wireless signal and deep learning, the authors proposed a system for learning classification using deep learning, mainly including a data preprocessing stage, an activity detection stage, a learning stage and a classification stage. During the activity detection model stage, a correlation-based model was used to detect the time of the activity occurrence and the activity time interval, thus solving the problem that the waveform changes due to variable environment at stable time. During the activity recognition stage, the network was studied by innovative deep learning to conduct training for activity learning. By replacing the fingerprint way, which is used broadly today, with learning the CSI signal information of activities, we classified the activities through trained network.