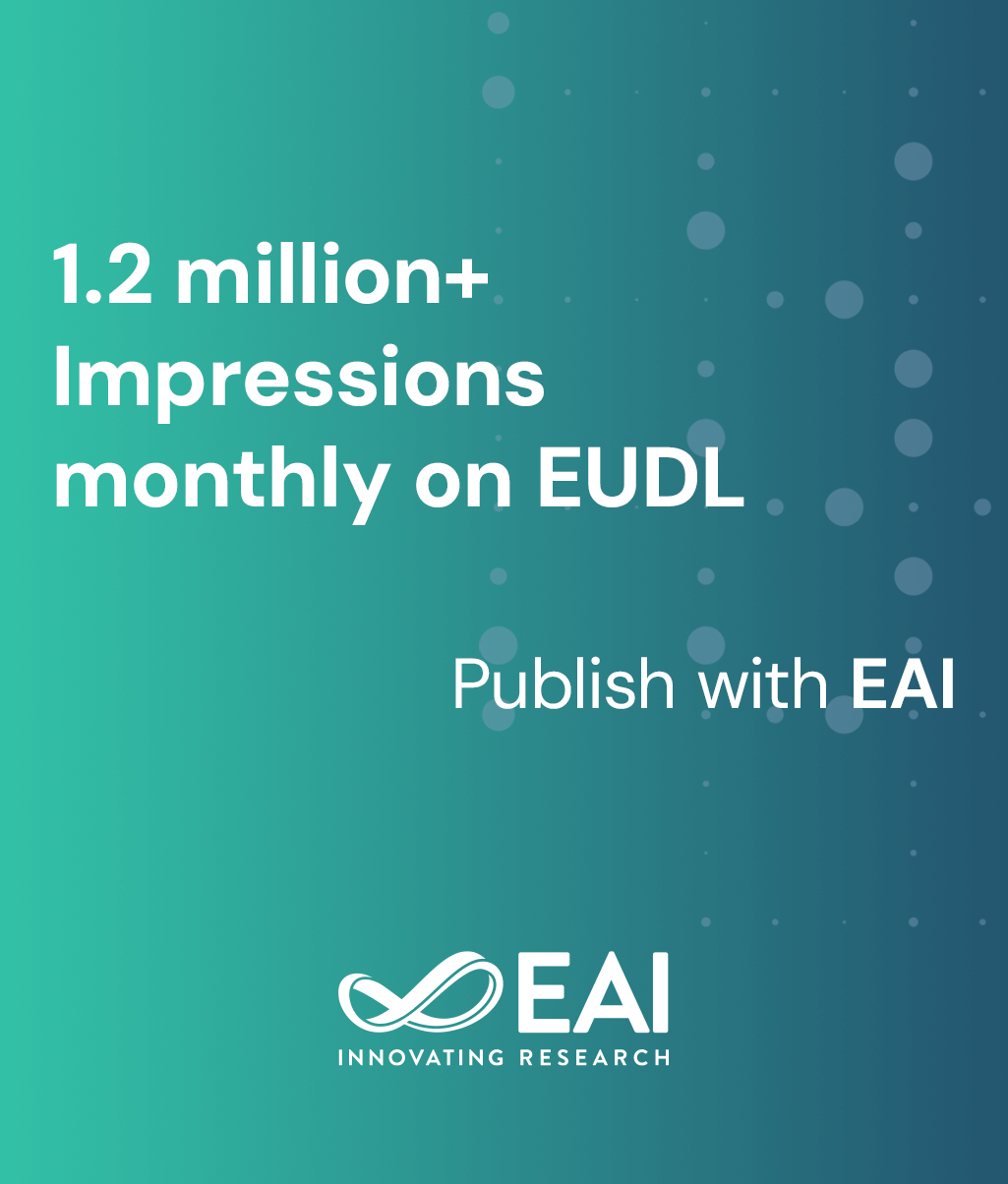
Research Article
Wi-Fi-Enabled Automatic Eating Moment Monitoring Using Smartphones
@INPROCEEDINGS{10.1007/978-3-030-42029-1_6, author={Zhenzhe Lin and Yucheng Xie and Xiaonan Guo and Chen Wang and Yanzhi Ren and Yingying Chen}, title={Wi-Fi-Enabled Automatic Eating Moment Monitoring Using Smartphones}, proceedings={IoT Technologies for HealthCare. 6th EAI International Conference, HealthyIoT 2019, Braga, Portugal, December 4--6, 2019, Proceedings}, proceedings_a={HEALTHYIOT}, year={2020}, month={6}, keywords={Eating moment monitoring WiFi sensing Healthy eating}, doi={10.1007/978-3-030-42029-1_6} }
- Zhenzhe Lin
Yucheng Xie
Xiaonan Guo
Chen Wang
Yanzhi Ren
Yingying Chen
Year: 2020
Wi-Fi-Enabled Automatic Eating Moment Monitoring Using Smartphones
HEALTHYIOT
Springer
DOI: 10.1007/978-3-030-42029-1_6
Abstract
Dietary habits are closely correlated with people’s health. Study reveals that unhealthy eating habits may cause various diseases such as obesity, diabetes and anemia. To help users create good eating habits, eating moment monitoring plays a significant role. However, traditional methods mainly rely on manual self-report or wearable devices, which either require much user efforts or intrusive dedicated hardware. In this work, we propose a user effort-free eating moment monitoring system by leveraging the WiFi signals extracted from the commercial off-the-shelf (COTS) smartphones. In particular, our system captures the eating activities of users to determine the eating moments. The proposed system can further identify the fine-grained food intake gestures (e.g., eating with fork, knife, spoon, chopsticks and bard hand) to estimate the detailed eating episode for each food intake gesture. Utilizing the dietary information, our system shows the potential to infer the food category and food amount. Extensive experiments with 10 subjects over 400-min eating show that our system can recognize a user’s food intake gestures with up to 97.8% accuracy and estimate the dietary moment within 1.1-s error.