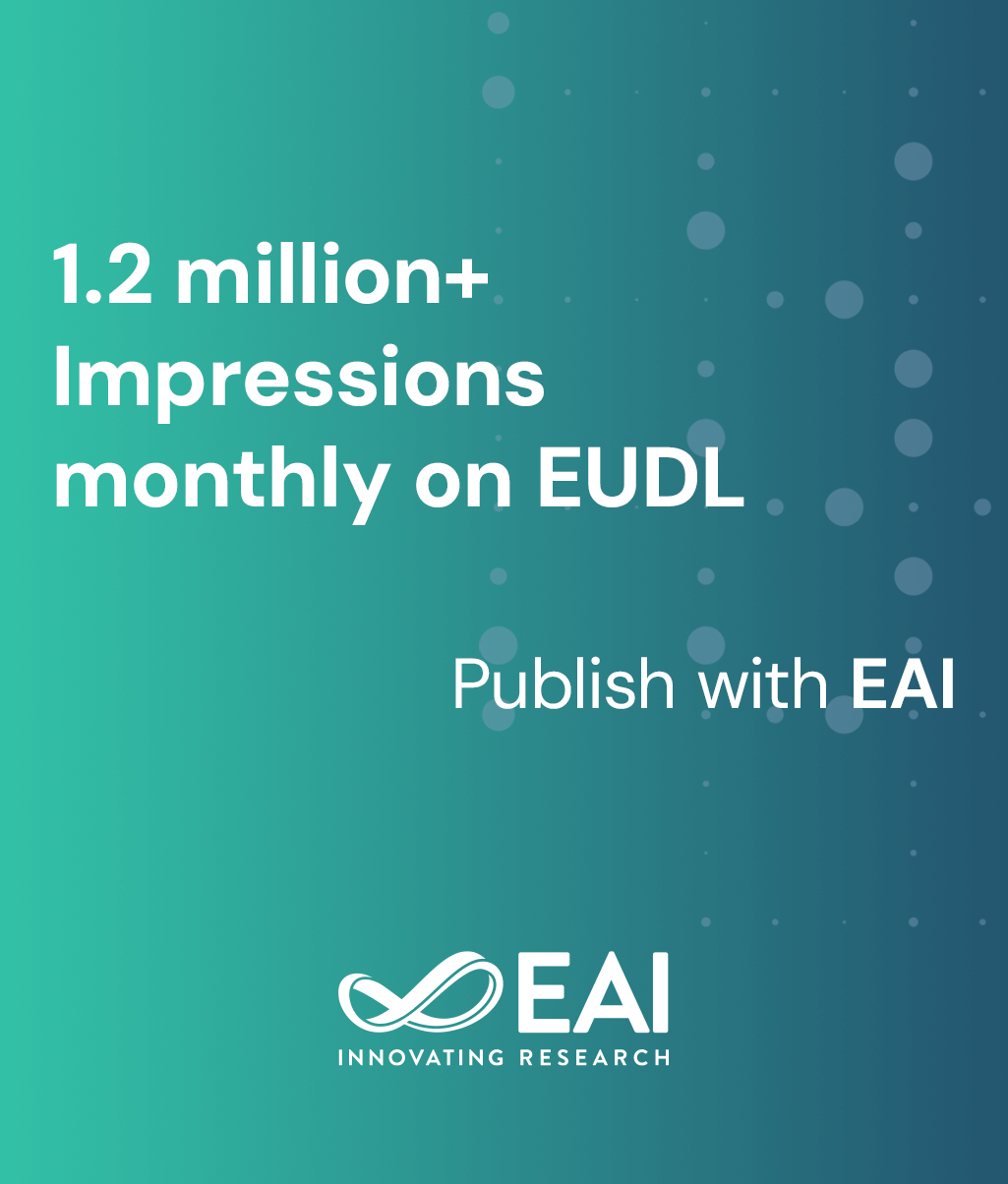
Research Article
Analysis of Software Vulnerabilities Using Machine Learning Techniques
119 downloads
@INPROCEEDINGS{10.1007/978-3-030-41593-8_3, author={Doffou Diako and Odilon Achiepo and Edoete Mensah}, title={Analysis of Software Vulnerabilities Using Machine Learning Techniques}, proceedings={e-Infrastructure and e-Services for Developing Countries. 11th EAI International Conference, AFRICOMM 2019, Porto-Novo, Benin, December 3--4, 2019, Proceedings}, proceedings_a={AFRICOMM}, year={2020}, month={2}, keywords={Machine learning Vulnerabilities Naive Bayes Support vectors machines CVSS}, doi={10.1007/978-3-030-41593-8_3} }
- Doffou Diako
Odilon Achiepo
Edoete Mensah
Year: 2020
Analysis of Software Vulnerabilities Using Machine Learning Techniques
AFRICOMM
Springer
DOI: 10.1007/978-3-030-41593-8_3
Abstract
With the increasing development of software technologies, we see that software vulnerabilities are a very critical issue of IT security. Because of their serious impacts, many different approaches have been proposed in recent decades to mitigate the damage caused by software vulnerabilities. Machine learning is also part of an approach to solve this problem. The main objective of this document is to provide three supervised machine to predict software vulnerabilities from a dataset of 6670 observations from national vulnerabilities database (NVD). The effectiveness of the proposed models has been evaluated with several performance indicators including Accuracy.
Copyright © 2019–2025 ICST