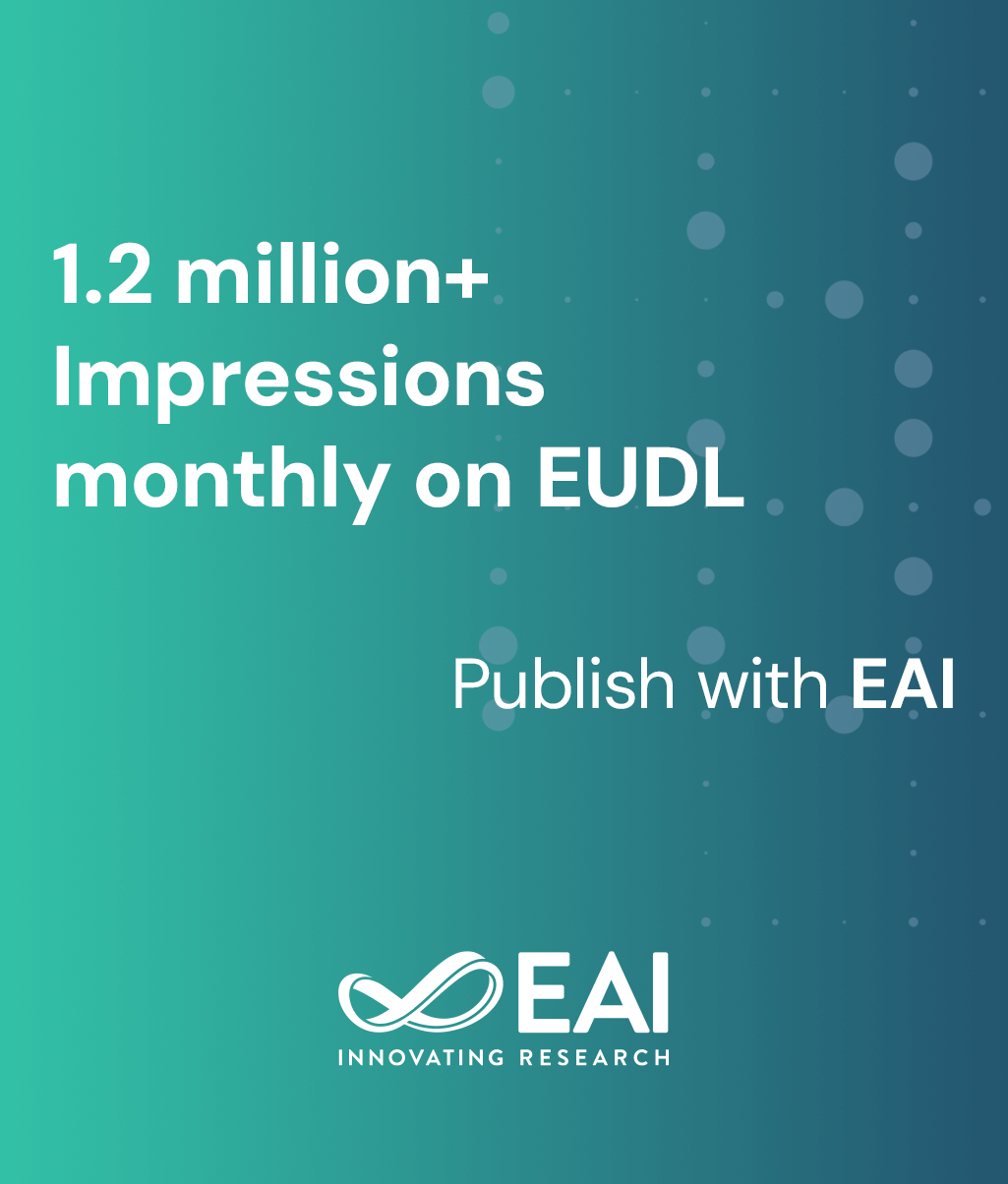
Research Article
Recent Approaches to Drift Effects in Credit Rating Models
@INPROCEEDINGS{10.1007/978-3-030-41593-8_18, author={Rachael Chikoore and Okuthe Kogeda and S. Ojo}, title={Recent Approaches to Drift Effects in Credit Rating Models}, proceedings={e-Infrastructure and e-Services for Developing Countries. 11th EAI International Conference, AFRICOMM 2019, Porto-Novo, Benin, December 3--4, 2019, Proceedings}, proceedings_a={AFRICOMM}, year={2020}, month={2}, keywords={Credit rating Drift effect Concept drift Population drift Prediction Approaches Credit rating models Probability distribution}, doi={10.1007/978-3-030-41593-8_18} }
- Rachael Chikoore
Okuthe Kogeda
S. Ojo
Year: 2020
Recent Approaches to Drift Effects in Credit Rating Models
AFRICOMM
Springer
DOI: 10.1007/978-3-030-41593-8_18
Abstract
Credit Rating is the valuation of the credit worthiness of the borrowing entity, which gives an indication of the borrower’s current credit position and the probability of default. A credit rating model must be very accurate in doing its predictions because critical decisions are made based on the classification that would have been made for the prospective borrower. Different changes occur in the environment that would have been used to come up with the initial model, which might not be applicable to the current sample population and this might have an effect on the prediction accuracy. Changes to the data stream, economic climate, social and cultural environment may cause a drift. Drift shows that there is a change in probability distribution of the concept under study. Population drift is an example of concept drift. Having a static credit rating model will bring challenges in future predictions, hence, there is the need for designing a dynamic credit rating system that caters for the changes that might occur to the initial population sample in order to maintain the prediction accuracy of the model. In this paper, a detailed literature study was conducted exploring recent solution approaches to drift effect in credit rating models. A comprehensive recent solutions is presented in this paper that could be a source of information of interested researchers.