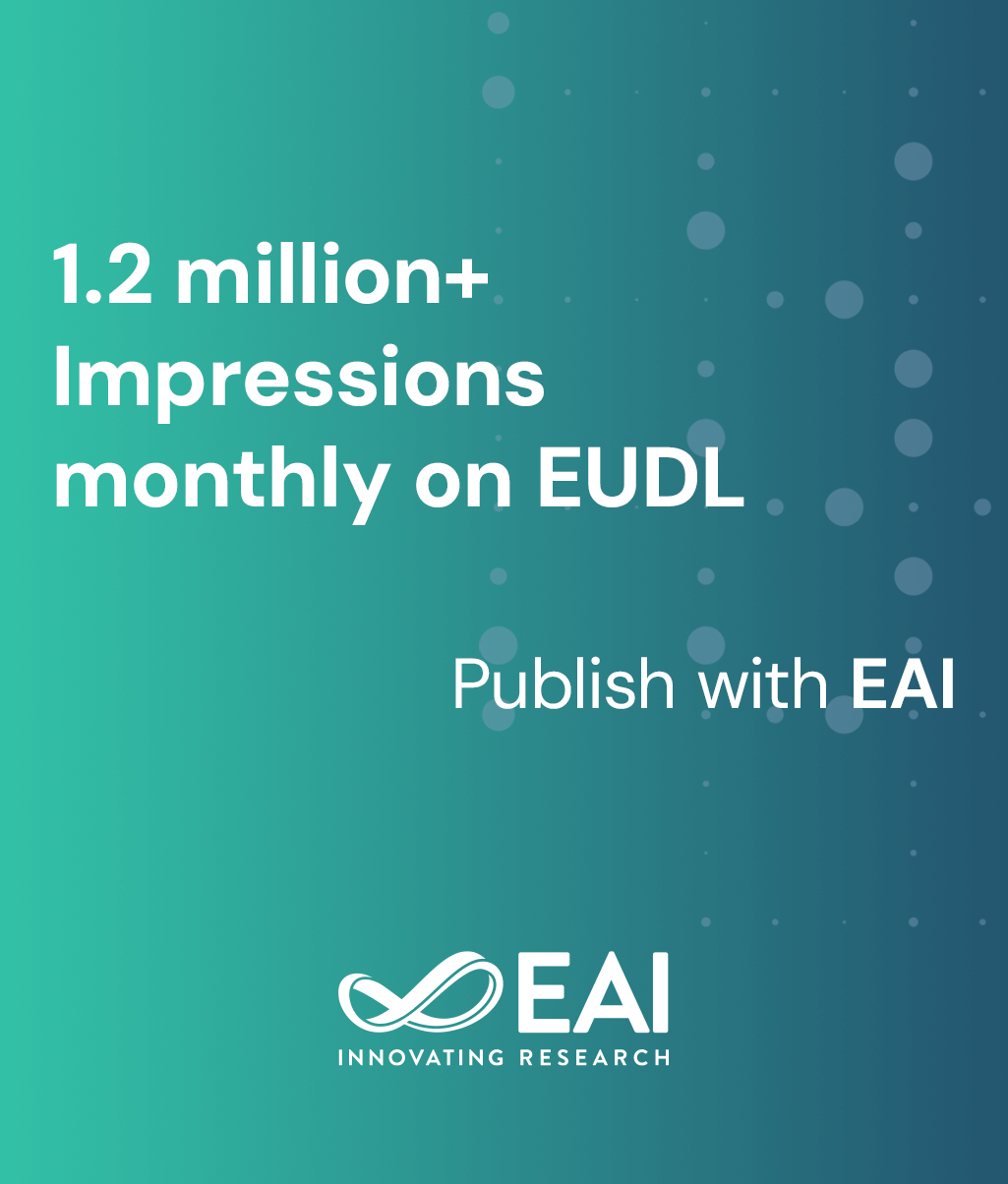
Research Article
: A Students Academic Performances Prediction System
@INPROCEEDINGS{10.1007/978-3-030-41593-8_16, author={Iffanice Houndayi and Vinasetan Houndji and Pierre Zohou and Eug\'{e}ne Ezin}, title={: A Students Academic Performances Prediction System}, proceedings={e-Infrastructure and e-Services for Developing Countries. 11th EAI International Conference, AFRICOMM 2019, Porto-Novo, Benin, December 3--4, 2019, Proceedings}, proceedings_a={AFRICOMM}, year={2020}, month={2}, keywords={Students performances prediction Machine learning Classification Regression Teaching unit LMD}, doi={10.1007/978-3-030-41593-8_16} }
- Iffanice Houndayi
Vinasetan Houndji
Pierre Zohou
Eugène Ezin
Year: 2020
: A Students Academic Performances Prediction System
AFRICOMM
Springer
DOI: 10.1007/978-3-030-41593-8_16
Abstract
This paper presents a system, called , that predicts the academic performances of students in the LMD system. The approach used allows to establish, for each of the teaching units of a given semester, some estimates of the students results. To achieve this, various machine learning techniques were used. In order to choose the best model for each teaching unit, we have tested 9 different algorithms offered by the Python Scikit-learn library to make predictions. The experiments were performed on data collected over two years at “Institut de Formation et de Recherche en Informatique (IFRI)” of University of Abomey-Calavi, Benin. The results obtained on the test data reveal that, on five of the nine teaching units for which the work was conducted, we obtain an F2-score of at least 75% for the classification and an RMSE of less than or equal to 2.93 for the regression. The solution therefore provides relatively good results with regard to the dataset used.