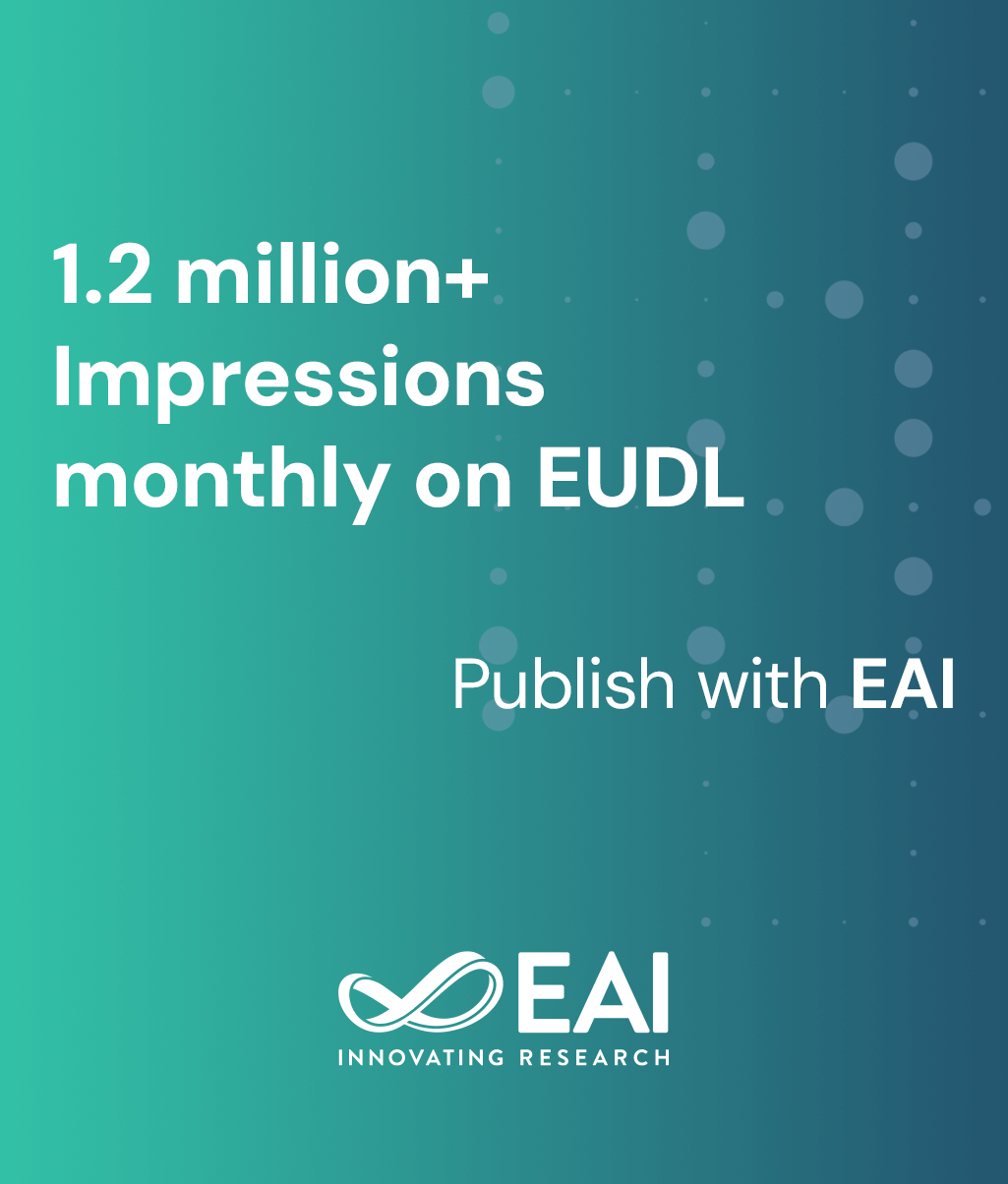
Research Article
Multi-agent Reinforcement Learning for Joint Wireless and Computational Resource Allocation in Mobile Edge Computing System
@INPROCEEDINGS{10.1007/978-3-030-37262-0_12, author={Yawen Zhang and Weiwei Xia and Feng Yan and Huaqing Cheng and Lianfeng Shen}, title={Multi-agent Reinforcement Learning for Joint Wireless and Computational Resource Allocation in Mobile Edge Computing System}, proceedings={Ad Hoc Networks. 11th EAI International Conference, ADHOCNETS 2019, Queenstown, New Zealand, November 18--21, 2019, Proceedings}, proceedings_a={ADHOCNETS}, year={2020}, month={1}, keywords={Mobile edge computing Joint resource allocation Multi-agent reinforcement learning Variable learning rate}, doi={10.1007/978-3-030-37262-0_12} }
- Yawen Zhang
Weiwei Xia
Feng Yan
Huaqing Cheng
Lianfeng Shen
Year: 2020
Multi-agent Reinforcement Learning for Joint Wireless and Computational Resource Allocation in Mobile Edge Computing System
ADHOCNETS
Springer
DOI: 10.1007/978-3-030-37262-0_12
Abstract
Mobile edge computing (MEC) is a new paradigm to provide computing capabilities at the edge of pervasive radio access networks in close proximity to intelligent terminals. In this paper, a resource allocation strategy based on the variable learning rate multi-agent reinforcement learning (VLR-MARL) algorithm is proposed in the MEC system to maximize the long term utility of all intelligent terminals while ensuring the intelligent terminals’ quality of service requirement. The novelty of this algorithm is that each agent only needs to maintain its own action value function so that the computationally expensive issue with the large action space can be avoided. Moreover, the learning rate is changed according to the expected payoff of the current strategy to speed up convergence and get the optimal solution. Simulation results show our algorithm performs better than other reinforcement learning algorithm both on the learning speed and users’ long term utilities.