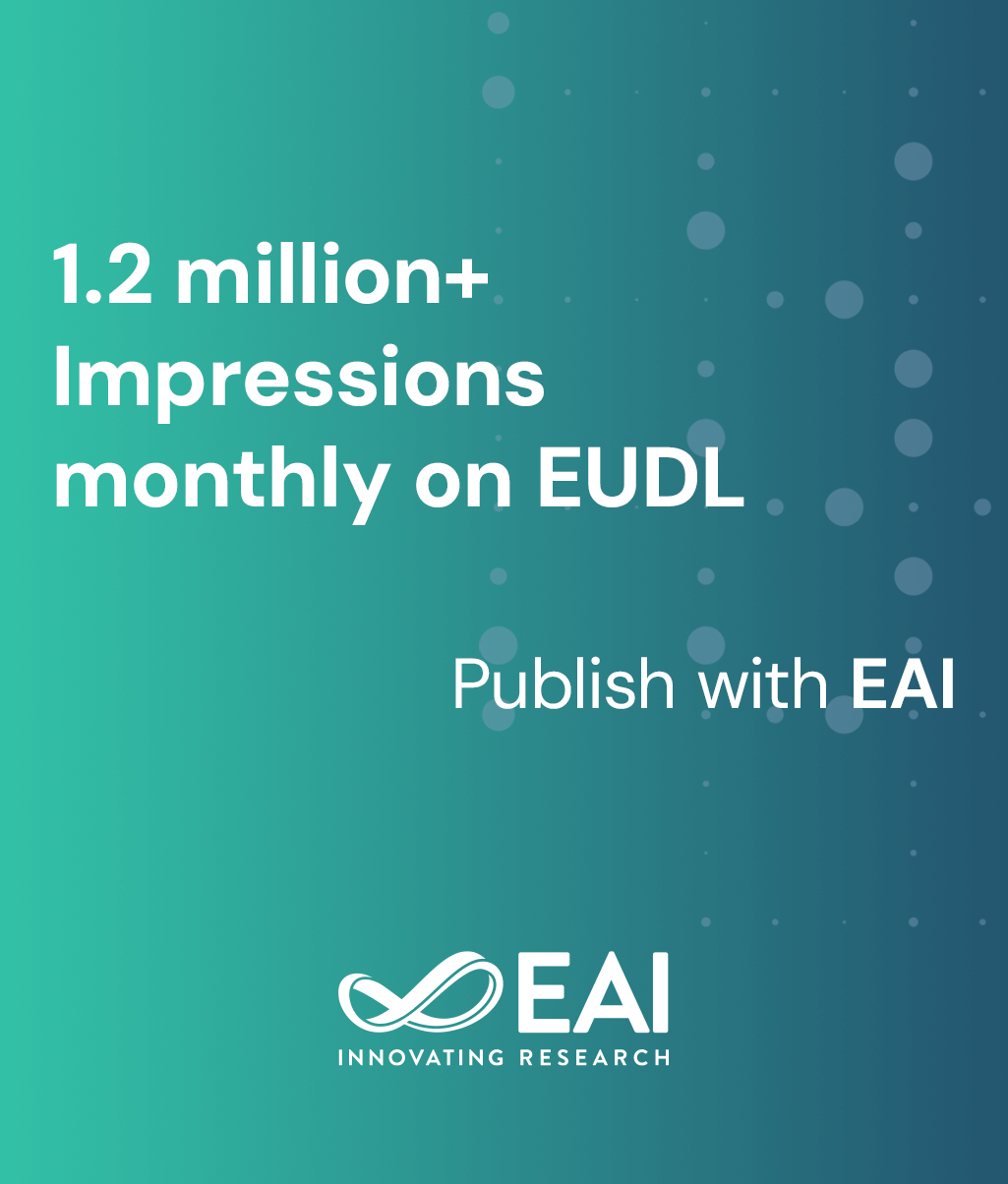
Research Article
Fair Resource Allocation Based on Deep Reinforcement Learning in Fog Networks
@INPROCEEDINGS{10.1007/978-3-030-37262-0_11, author={Huihui Xu and Yijun Zu and Fei Shen and Feng Yan and Fei Qin and Lianfeng Shen}, title={Fair Resource Allocation Based on Deep Reinforcement Learning in Fog Networks}, proceedings={Ad Hoc Networks. 11th EAI International Conference, ADHOCNETS 2019, Queenstown, New Zealand, November 18--21, 2019, Proceedings}, proceedings_a={ADHOCNETS}, year={2020}, month={1}, keywords={Fog computing Deep reinforcement learning Resource allocation}, doi={10.1007/978-3-030-37262-0_11} }
- Huihui Xu
Yijun Zu
Fei Shen
Feng Yan
Fei Qin
Lianfeng Shen
Year: 2020
Fair Resource Allocation Based on Deep Reinforcement Learning in Fog Networks
ADHOCNETS
Springer
DOI: 10.1007/978-3-030-37262-0_11
Abstract
As the terminal devices grow explosively, the resource in a fog network may not satisfy all the requirement of them. Thus scheduling the resource reasonably becomes a huge challenge in the future 5G network. In the paper, we propose a fair resource allocation algorithm based on deep reinforcement learning, which makes full use of the computational resource in a fog network. The goal of the algorithm is to complete processing the tasks fairly for all the user nodes (UNs). The fog nodes (FNs) are expected to assign their central processing unit (CPU) cores to process offloading tasks reasonably. We apply the Deep Q-Learning Network (DQN) to solve the problem of resource scheduling. Firstly, we establish an evaluation model of a priority to set the priority for the offloading tasks, which is related to the reward in the reinforcement learning. Secondly, the model of reinforcement learning is built by taking the situation of UNs and resource allocation scheme as the state of environment and the action of the agent, respectively. Subsequently, a loss function is analysed to update the parameters of a deep neural network. Finally, numerical simulations demonstrate the feasibility and effectiveness of our proposed algorithm.