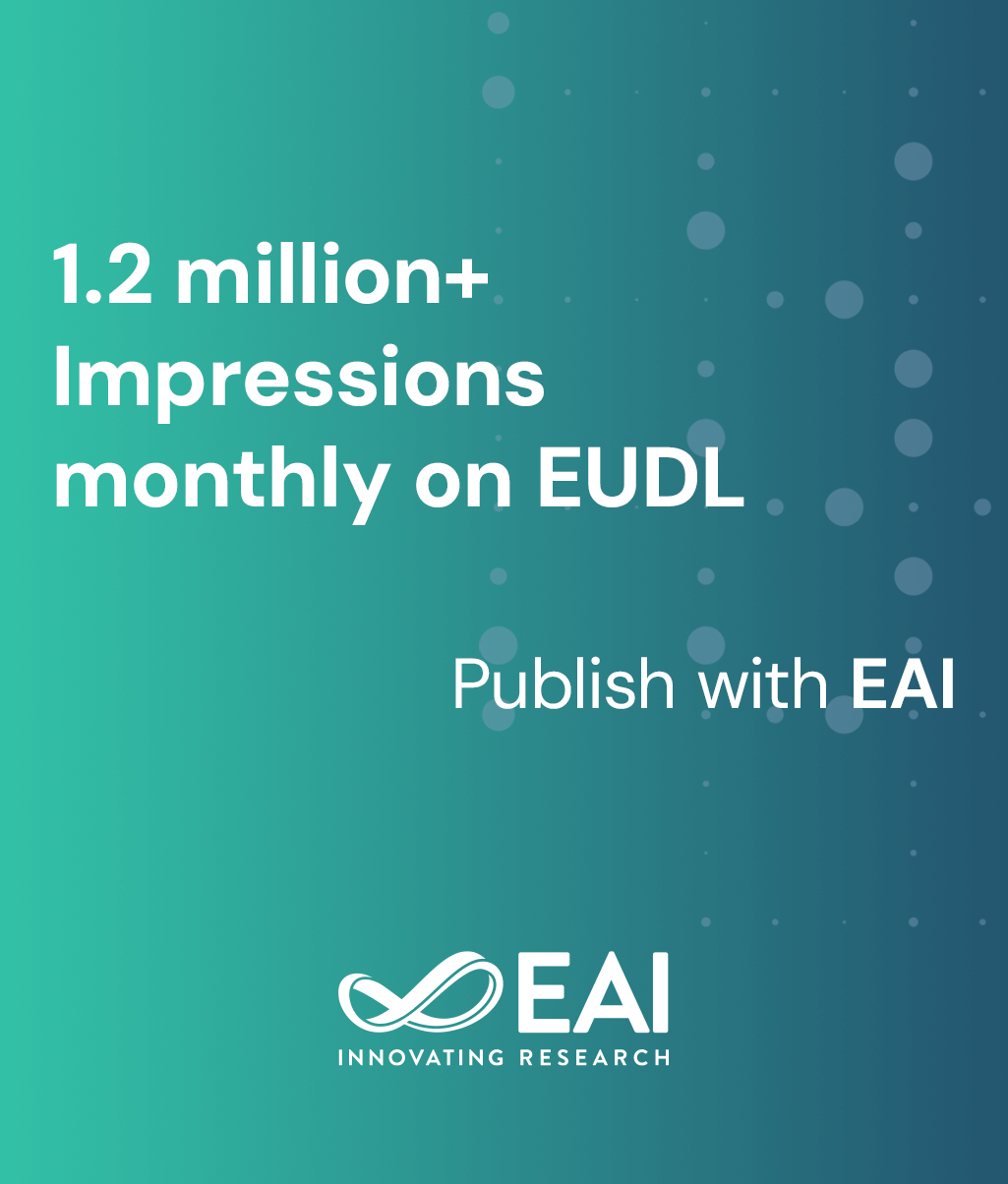
Research Article
Human Activity Recognition Using Wi-Fi Imaging with Deep Learning
@INPROCEEDINGS{10.1007/978-3-030-36442-7_2, author={Yubing Li and Yujiao Ma and Nan Yang and Wei Shi and Jizhong Zhao}, title={Human Activity Recognition Using Wi-Fi Imaging with Deep Learning}, proceedings={Broadband Communications, Networks, and Systems. 10th EAI International Conference, Broadnets 2019, Xi’an, China, October 27-28, 2019, Proceedings}, proceedings_a={BROADNETS}, year={2019}, month={12}, keywords={RFID Indoor localization Tag mutual interference}, doi={10.1007/978-3-030-36442-7_2} }
- Yubing Li
Yujiao Ma
Nan Yang
Wei Shi
Jizhong Zhao
Year: 2019
Human Activity Recognition Using Wi-Fi Imaging with Deep Learning
BROADNETS
Springer
DOI: 10.1007/978-3-030-36442-7_2
Abstract
Robots have been increasingly used in production line and real life, such as warehousing, logistics, security, smart home and so on. In most applications, localization is always one of the most basic tasks of the robot. To acquire the object location, existing work mainly relies on computer vision. Such methods encounter many problems in practice, such as high computational complexity, large influence by light conditions, and heavy crafting of pre-training. These problems have become one of the key factors that constrains the precise automation of robots. This paper proposes an RFID-based robot navigation and target localization scheme, which is easy to deploy, low cost, and can work in non-line-of-sight scenarios. The main contributions of this paper are as follows: 1. We collect the phase variation of the tag by a rotating reader antenna, and calculate the azimuth of the tag relative to the antenna by the channel similarity weighted average method. Then, the location of the tag is determined by the AoA method. 2. Based on the theory of tag equivalent circuit, antenna radiation field, and cylindrical symmetry oscillator mutual impedance, the phenomenon of RSS weakening of adjacent tags is analyzed. Based on this phenomenon, we achieve accurate target localization and multi-target relative localization by utilizing region segmentation and dynamic time warping algorithms. 3. The proposed scheme is lightweight and low-cost. We built a prototype system using commercial UHF RFID readers and passive tags, and conduct extensive experiments. The experimental results show that the model can effectively achieve the precise location of the robot and the object with an average error of 27 cm and 2 cm.