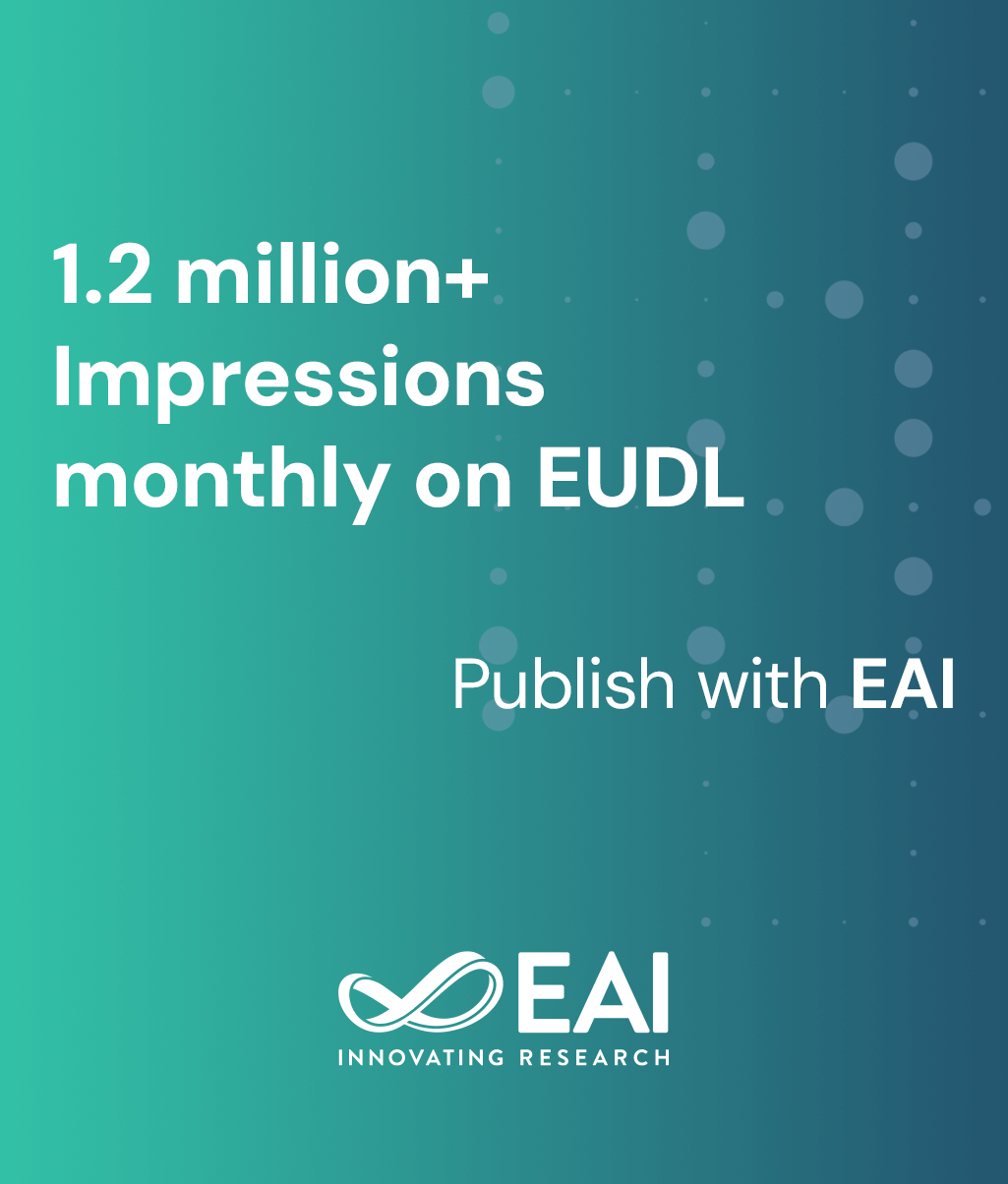
Research Article
Toward Detection of Driver Drowsiness with Commercial Smartwatch and Smartphone
@INPROCEEDINGS{10.1007/978-3-030-36442-7_15, author={Liangliang Lin and Hongyu Yang and Yang Liu and Haoyuan Zheng and Jizhong Zhao}, title={Toward Detection of Driver Drowsiness with Commercial Smartwatch and Smartphone}, proceedings={Broadband Communications, Networks, and Systems. 10th EAI International Conference, Broadnets 2019, Xi’an, China, October 27-28, 2019, Proceedings}, proceedings_a={BROADNETS}, year={2019}, month={12}, keywords={Arm gesture Non-cooperative target Localization Smart-watch}, doi={10.1007/978-3-030-36442-7_15} }
- Liangliang Lin
Hongyu Yang
Yang Liu
Haoyuan Zheng
Jizhong Zhao
Year: 2019
Toward Detection of Driver Drowsiness with Commercial Smartwatch and Smartphone
BROADNETS
Springer
DOI: 10.1007/978-3-030-36442-7_15
Abstract
In the life, there are always many objects that are unable to actively contact with us, such as keychains, glasses and mobile phones. In general, they are referred to non-cooperative targets. Non-cooperative targets are often overlooked by users while being hard to find. It will be convenient if we can localize those non-cooperative targets. We propose a non-cooperative target localization system which based on MEMS. We detect the arm posture changes of the user by using the MEMS sensors which embedded in the smart watch. First distinguish the arm motions, identify the final motion, and then perform the localization. There are two essential models in our system. The first step is arm gesture estimation model which based on MESE sensor in smart watch. we first collect the MEMS sensor data from the watch. And then the arm kinematic model and formulate the mathematical relationship between arm degrees of freedom with and the gestures of watch. We compare the results of the four actions which are important in the later model with the Kinect observations. The errors in the space are less than 0.14 m. The second step is non-cooperative target localization model that based on the first step. We use the 5-degrees data of the arm to train the classification model and identify the key actions in the scene. In this step, we estimate the location of non-cooperative targets through the type of interactive actions. To demonstrate the effectiveness of our system, we implement it on tracking keys and mobile phones in practice. The experiments show that the localization accuracy is >83%.