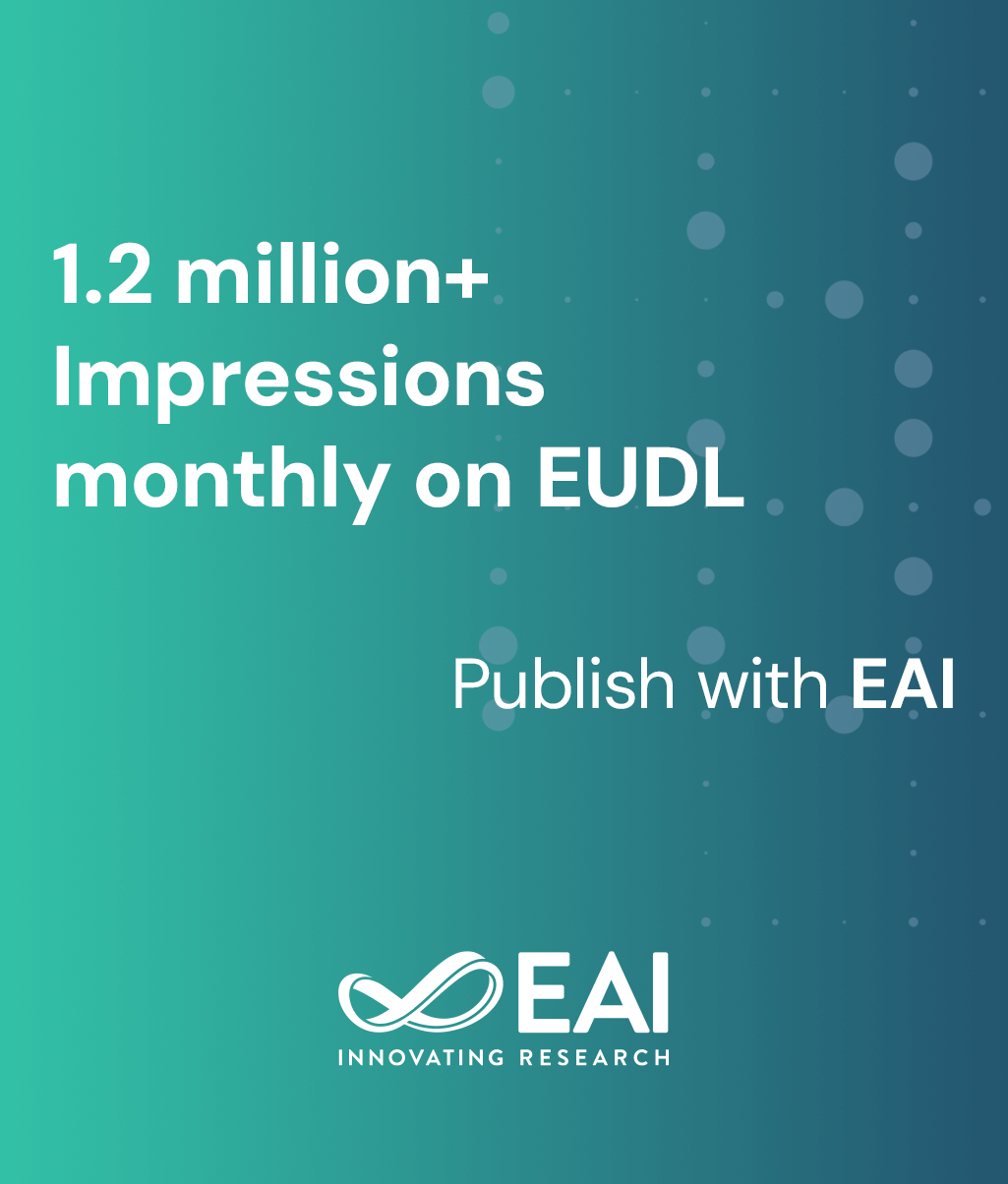
Research Article
Dynamic IFFSM Modeling Using IFHMM-Based Bayesian Non-parametric Learning for Energy Disaggregation in Smart Solar Home System
@INPROCEEDINGS{10.1007/978-3-030-36442-7_11, author={Kalthoum Zaouali and Mohamed Ammari and Amine Chouaieb and Ridha Bouallegue}, title={Dynamic IFFSM Modeling Using IFHMM-Based Bayesian Non-parametric Learning for Energy Disaggregation in Smart Solar Home System}, proceedings={Broadband Communications, Networks, and Systems. 10th EAI International Conference, Broadnets 2019, Xi’an, China, October 27-28, 2019, Proceedings}, proceedings_a={BROADNETS}, year={2019}, month={12}, keywords={Bayesian Non-Parametric (BNP) Energy disaggregation Infinite Factorial Finite State Machine (IFFSM) Infinite Factorial Hidden Markov Model (IFHMM) Non-Intrusive Load Monitoring (NILM) Solar Home System (SHS)}, doi={10.1007/978-3-030-36442-7_11} }
- Kalthoum Zaouali
Mohamed Ammari
Amine Chouaieb
Ridha Bouallegue
Year: 2019
Dynamic IFFSM Modeling Using IFHMM-Based Bayesian Non-parametric Learning for Energy Disaggregation in Smart Solar Home System
BROADNETS
Springer
DOI: 10.1007/978-3-030-36442-7_11
Abstract
Recently, the analysis and recognition of each appliance’s energy consumption are fundamental in smart homes and smart buildings systems. Our paper presents a novel Non-Intrusive Load Monitoring (NILM) recognition method based on Bayesian Non-Parametric (BNP) learning approach to solve the problem of energy disaggregation for smart Solar Home System (SHS). Several researches assumed that there is prior information about the household appliances in order to restrict those that do not hold the maximum expectation for inference. Therefore, to deal with the unknown number of electrical appliances in a SHS, we have adapted a dynamic Infinite Factorial Hidden Markov Model (IFHMM) -based Infinite Factorial Finite State Machine (IFFSM) to our NILM times-series modeling as an unsupervised BNP learning method. Our suggested method can grip with few or nappropriate learning data as well as to standardize electrical appliance modeling. Our proposed method outperforms FHMM-based FSM modeling results illustrated in literature.