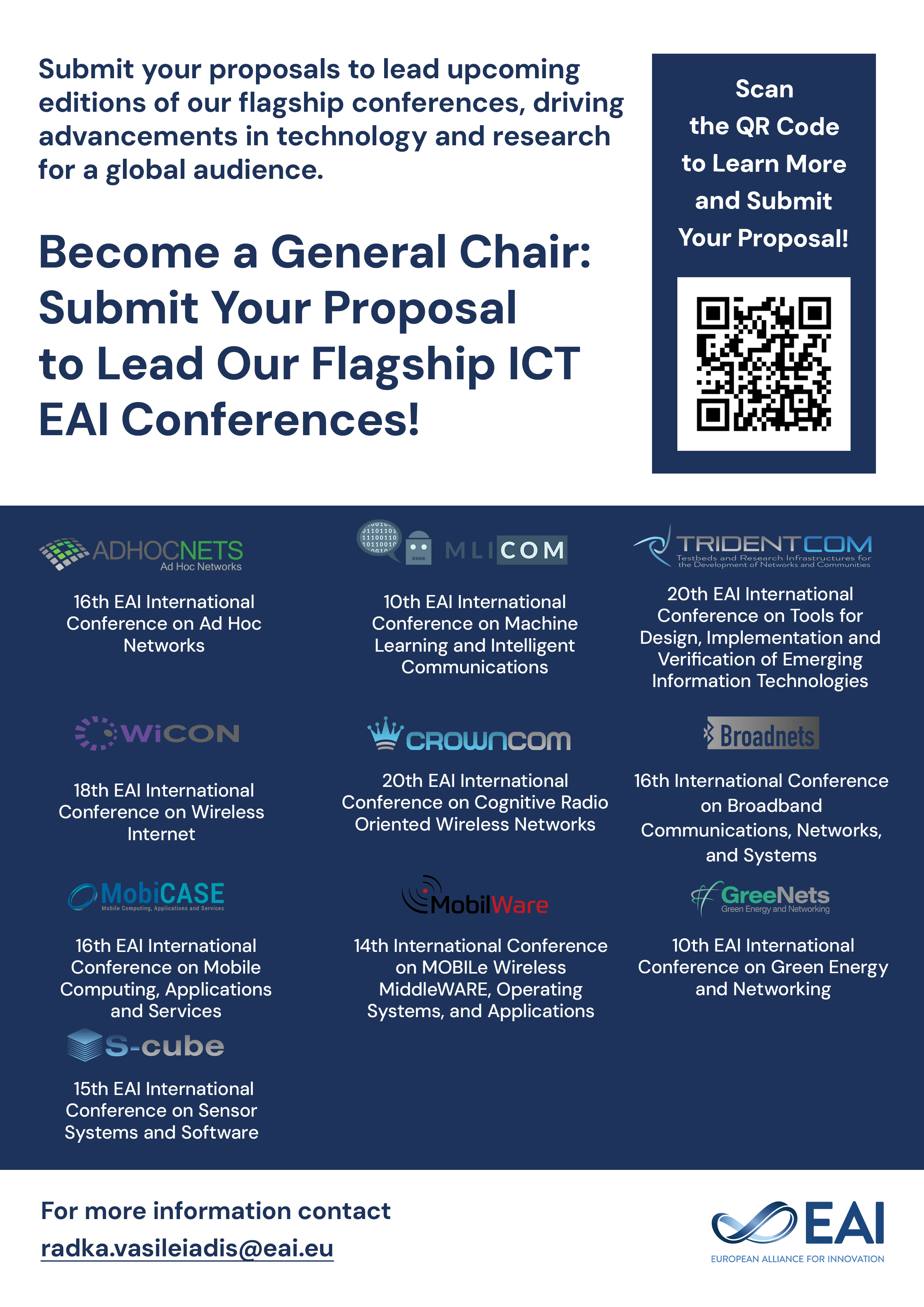
Research Article
Group Walking Recognition Based on Smartphone Sensors
@INPROCEEDINGS{10.1007/978-3-030-34833-5_8, author={Qimeng Li and Raffaele Gravina and Sen Qiu and Zhelong Wang and Weilin Zang and Ye Li}, title={Group Walking Recognition Based on Smartphone Sensors}, proceedings={Body Area Networks: Smart IoT and Big Data for Intelligent Health Management. 14th EAI International Conference, BODYNETS 2019, Florence, Italy, October 2-3, 2019, Proceedings}, proceedings_a={BODYNETS}, year={2019}, month={11}, keywords={Multi-user activity Group membership Walking group clustering Wearable sensors}, doi={10.1007/978-3-030-34833-5_8} }
- Qimeng Li
Raffaele Gravina
Sen Qiu
Zhelong Wang
Weilin Zang
Ye Li
Year: 2019
Group Walking Recognition Based on Smartphone Sensors
BODYNETS
Springer
DOI: 10.1007/978-3-030-34833-5_8
Abstract
Human group activity represents a potentially valuable contextually relevant source of information, which can be analyzed to support diverse human-centric applications. In recent year, more and more sensors are being pervasively spread in daily living environments, so giving excellent opportunities for using ubiquitous sensing to recognize group activities. In this paper, we used smartphone-based data and edge computing technologies to address group activity recognition, with particular focus on group walking. The data is provided by two groups of participants using a smartphone with embedded 9-DoF inertial sensors; several features are generated to identify group membership of each subject. Our results showed that the accelerometer rarely can be used alone to identify the group motion; in most situations, multiple sensor sources are required to determine group membership. Moreover, the use of 9-DoF sensors to identify group affiliation is still challenging, because, in a multi-user scenario, individual behaviors often have mutual contingency; therefore, the concept of proximity is also introduced to improve the classification algorithm.