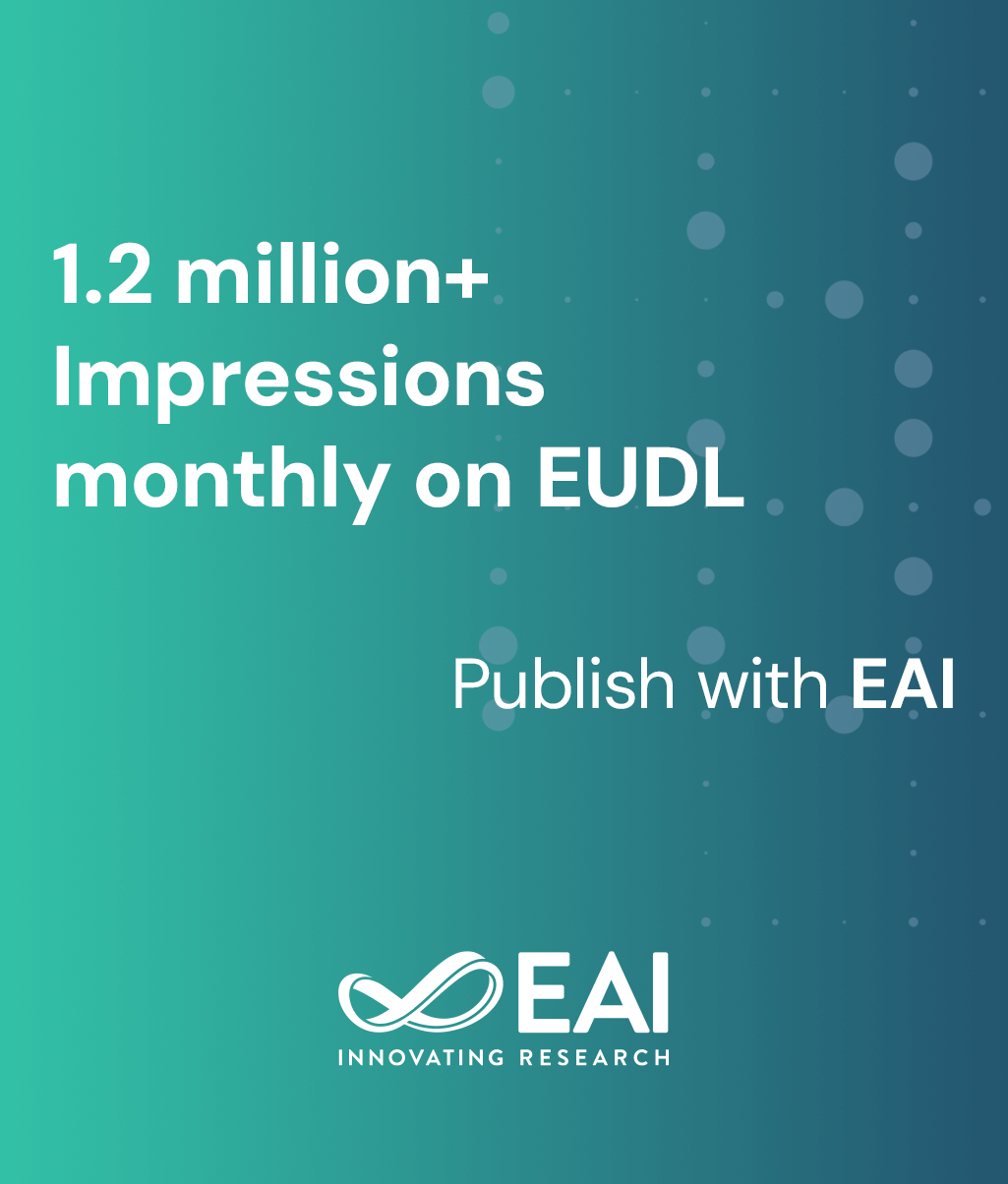
Research Article
The Smart Insole: A Pilot Study of Fall Detection
@INPROCEEDINGS{10.1007/978-3-030-34833-5_4, author={Xiaoye Qian and Haoyou Cheng and Diliang Chen and Quan Liu and Huan Chen and Haotian Jiang and Ming-Chun Huang}, title={The Smart Insole: A Pilot Study of Fall Detection}, proceedings={Body Area Networks: Smart IoT and Big Data for Intelligent Health Management. 14th EAI International Conference, BODYNETS 2019, Florence, Italy, October 2-3, 2019, Proceedings}, proceedings_a={BODYNETS}, year={2019}, month={11}, keywords={Pressure sensor array Fall detection Deep learning Smart insole Deep visualization}, doi={10.1007/978-3-030-34833-5_4} }
- Xiaoye Qian
Haoyou Cheng
Diliang Chen
Quan Liu
Huan Chen
Haotian Jiang
Ming-Chun Huang
Year: 2019
The Smart Insole: A Pilot Study of Fall Detection
BODYNETS
Springer
DOI: 10.1007/978-3-030-34833-5_4
Abstract
Falls are common events among human beings and raised a global health problem. Wearable sensors can provide quantitative assessments for fall-based movements. Automatic fall detection systems based on the wearable sensors are becoming popular in recent years. This paper proposes a new fall detection system based on the smart insole. Each smart insole contains pressure a pressure sensor array and can provide abundant pressure information during the daily activities. According to such information, the system can successfully distinguish the fall from other activities of daily livings (ADLs) using deep learning algorithms. To reduce the computational complexity through the classifiers, the raw data for each sensor in the time windows are utilized. Furthermore, the deep visualization approach is applied to provide an intuitive explanation of how the deep learning system works on distinguishing the fall events. Both quantitative and qualitative experiments are demonstrated in this paper to prove the feasibility and effectiveness of the proposed fall detection system.