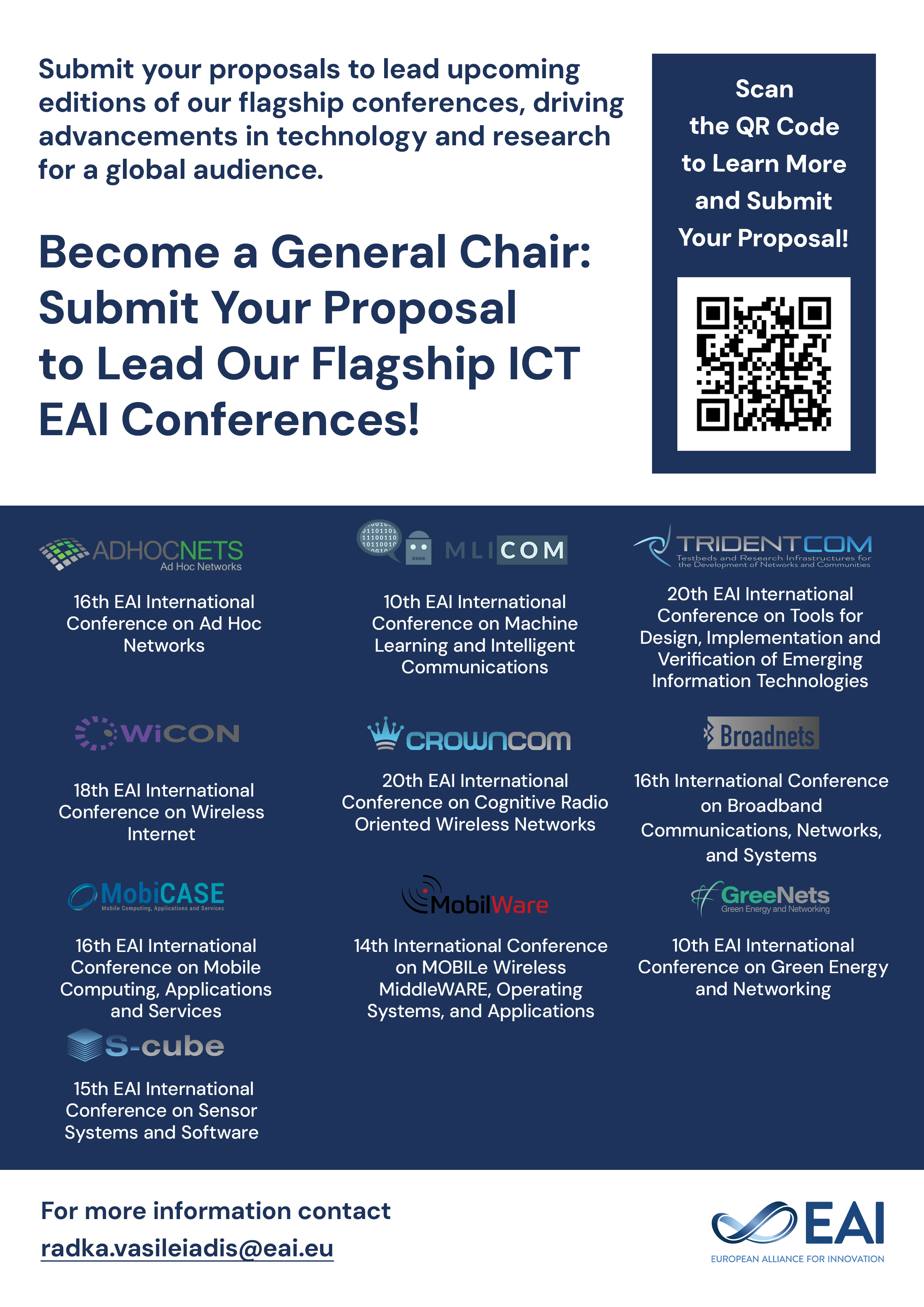
Research Article
Vertical Hand Position Estimation with Wearable Differential Barometery Supported by RFID Synchronization
@INPROCEEDINGS{10.1007/978-3-030-34833-5_3, author={Hymalai Bello and Jhonny Rodriguez and Paul Lukowicz}, title={Vertical Hand Position Estimation with Wearable Differential Barometery Supported by RFID Synchronization}, proceedings={Body Area Networks: Smart IoT and Big Data for Intelligent Health Management. 14th EAI International Conference, BODYNETS 2019, Florence, Italy, October 2-3, 2019, Proceedings}, proceedings_a={BODYNETS}, year={2019}, month={11}, keywords={Relative pressure Barometer RFID Order picking Wearable sensing}, doi={10.1007/978-3-030-34833-5_3} }
- Hymalai Bello
Jhonny Rodriguez
Paul Lukowicz
Year: 2019
Vertical Hand Position Estimation with Wearable Differential Barometery Supported by RFID Synchronization
BODYNETS
Springer
DOI: 10.1007/978-3-030-34833-5_3
Abstract
We demonstrate how a combination of a wrist-worn and stationary barometer can be used to track the vertical position of the user’s Hand with an accuracy in the range of 30 cm. To this end, the two barometers synchronized each time an RFID reader detects them being in proximity of each other. The accuracy is sufficient to detect a specific shelve of a cupboard on which something has been placed or determine if the user’s hand is touching his/her head or the torso. The advantage of the method over IMU based approaches is that it requires only a wrist-worn sensor (as could be implemented in a smartwatch) and a reference either in an often access location in the environment or a pocket (e.g.in the smartphone) and it does not depend on a stable magnetic environment. The proposed system was tested in two different activities: Shelve recognition in a warehouse picking scenario and movement of the arm to specific body locations. Despite the simplicity of our method, it shows initial results between 55–62% and 73–91% accuracy, respectively.