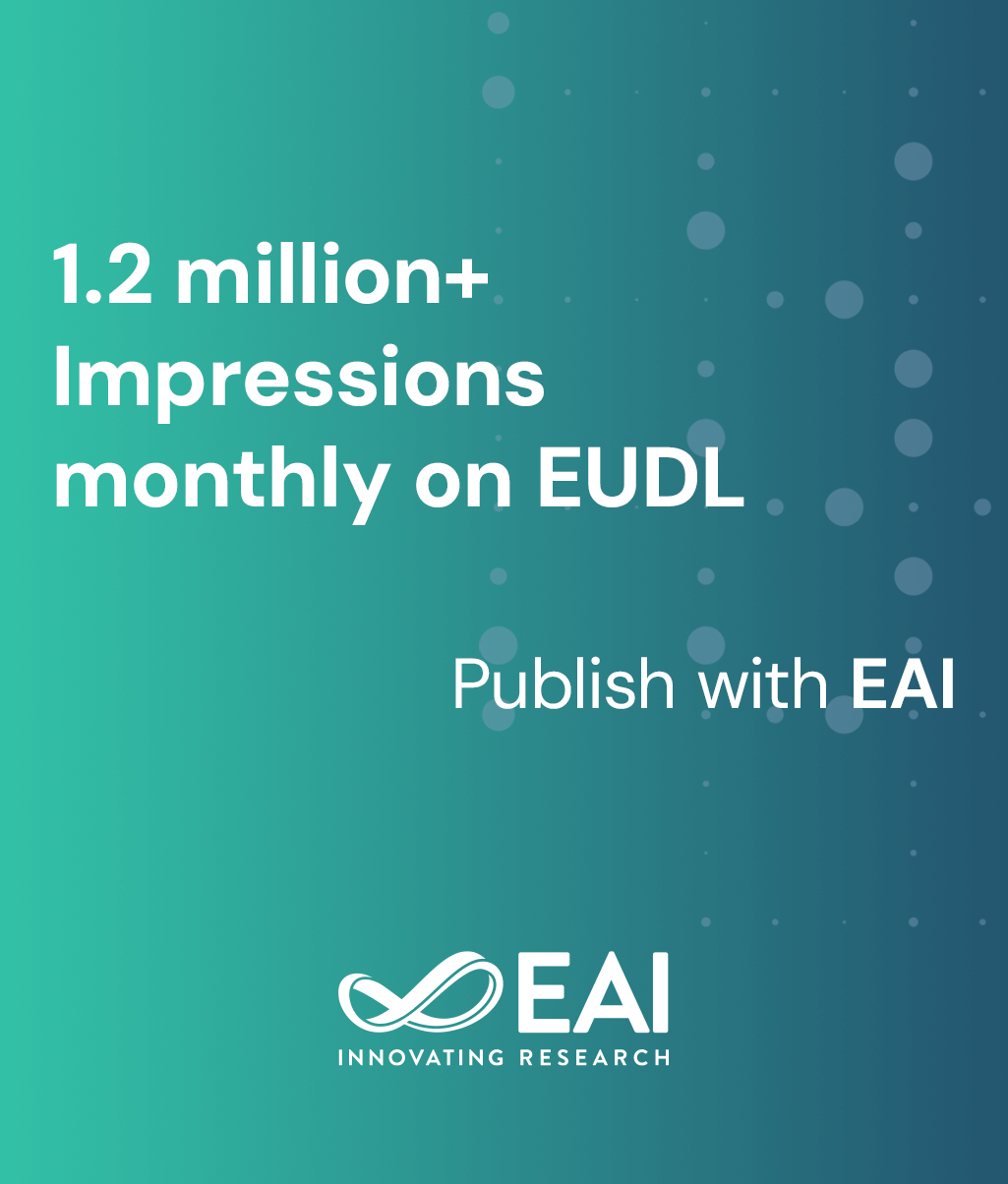
Research Article
Estimation of Skin Conductance Response Through Adaptive Filtering
@INPROCEEDINGS{10.1007/978-3-030-34833-5_17, author={Pietro Savazzi and Floriana Vasile and Natascia Brondino and Marco Vercesi and Pierluigi Politi}, title={Estimation of Skin Conductance Response Through Adaptive Filtering}, proceedings={Body Area Networks: Smart IoT and Big Data for Intelligent Health Management. 14th EAI International Conference, BODYNETS 2019, Florence, Italy, October 2-3, 2019, Proceedings}, proceedings_a={BODYNETS}, year={2019}, month={11}, keywords={Galvanic skin response Electrodermal activity Skin conductance response Adaptive filter Wearable sensor}, doi={10.1007/978-3-030-34833-5_17} }
- Pietro Savazzi
Floriana Vasile
Natascia Brondino
Marco Vercesi
Pierluigi Politi
Year: 2019
Estimation of Skin Conductance Response Through Adaptive Filtering
BODYNETS
Springer
DOI: 10.1007/978-3-030-34833-5_17
Abstract
The importance of medical wearable sensors is increasing in aiding both diagnostic and therapeutic protocols, in a wide area of health applications. Among them, the acquisition and analysis of electrodermal activity (EDA) may help in detecting seizures and different human emotional states. Nonnegative deconvolution represents an important step needed for decomposing the measured galvanic skin response (GSR) in its tonic and phasic components. In particular, the phasic component, also known as skin conductance response (SCR), is related to the sympathetic nervous system (SNS) activity, since it can be modeled as the linear convolution between the SCR driver events, modeled by sparse impulse signals, with an impulse response representing the sudomotor SNS innervation. In this paper, we propose a novel method for implementing this deconvolution by an adaptive filter, determined by solving a linear prediction problem, which results independent on the impulse response parameters, usually represented by sampling the biexponential Bateman function. The performance of the proposed approach is evaluated by using both synthetic and experimental data.