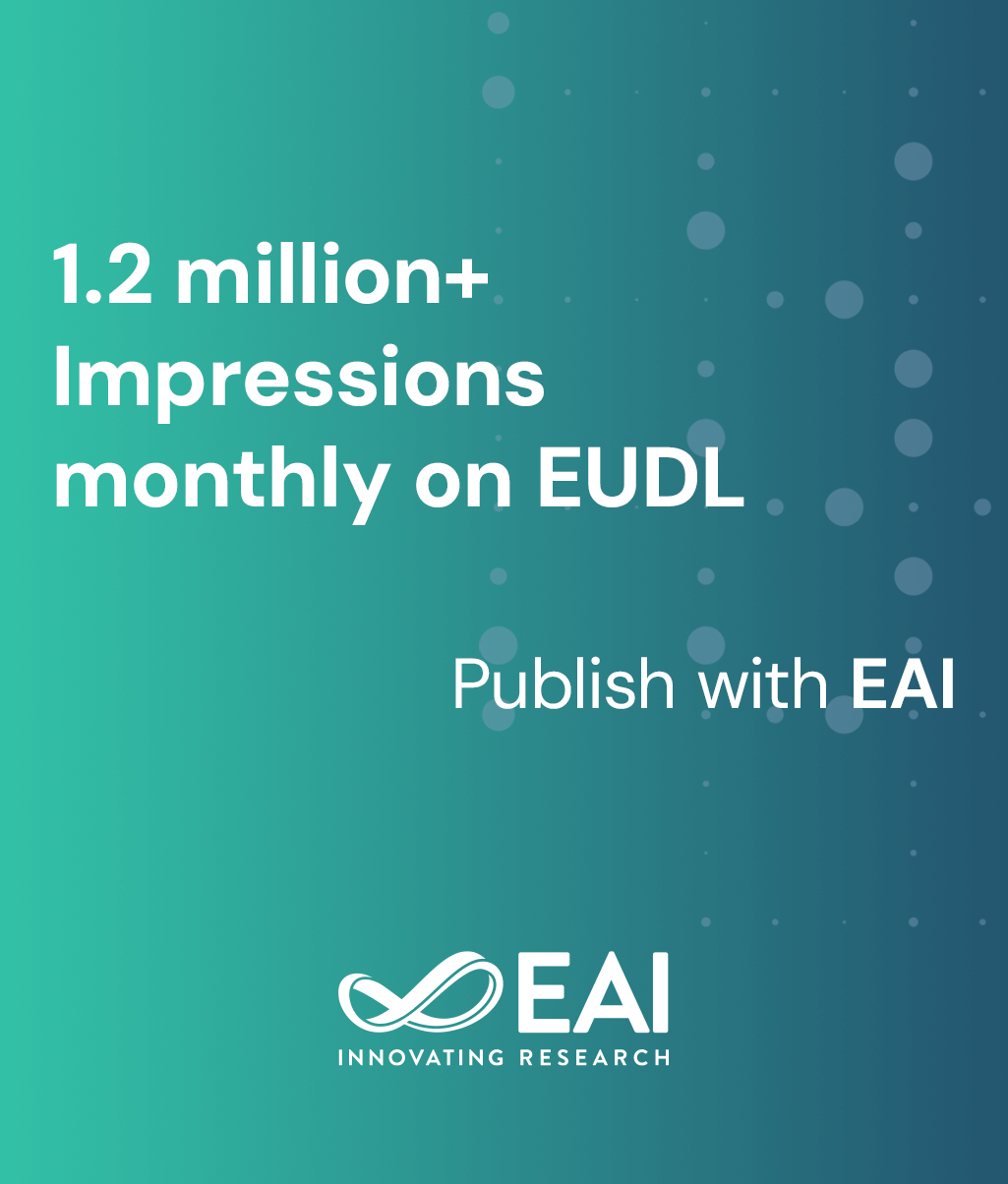
Research Article
Non-negative Matrix Factorization with Community Kernel for Dynamic Community Detection
@INPROCEEDINGS{10.1007/978-3-030-32388-2_64, author={Saisai Liu and Zhengyou Xia}, title={Non-negative Matrix Factorization with Community Kernel for Dynamic Community Detection}, proceedings={Machine Learning and Intelligent Communications. 4th International Conference, MLICOM 2019, Nanjing, China, August 24--25, 2019, Proceedings}, proceedings_a={MLICOM}, year={2019}, month={10}, keywords={Community detection Community kernel Data mining INMF NMF}, doi={10.1007/978-3-030-32388-2_64} }
- Saisai Liu
Zhengyou Xia
Year: 2019
Non-negative Matrix Factorization with Community Kernel for Dynamic Community Detection
MLICOM
Springer
DOI: 10.1007/978-3-030-32388-2_64
Abstract
Finding community structures from user data has become a hot topic in network analysis. However, there are rarely effective algorithms about the dynamic community detection. To recalculate the whole previous nodes and deal with excessive calculation is used to solve the problem of dynamically adding community nodes in the previous researches. In this paper, we propose an incremental community detection algorithm without recalculating the whole previous nodes using the incremental non-negative matrix factorization (INMF). In this algorithm, community kernel nodes with the largest node degree and adjacent triangle ratio is selected to calculate the data feature matrix, then the complexity of the calculations is largely simplified by reducing the dimension of the data feature matrix. We also propose a strategy to solve the problem of ensuring the feature space dimension and community number of NMF. We discuss our method with several previous ones on real data, and the results show that our method is effective and accurate in find potential communities.