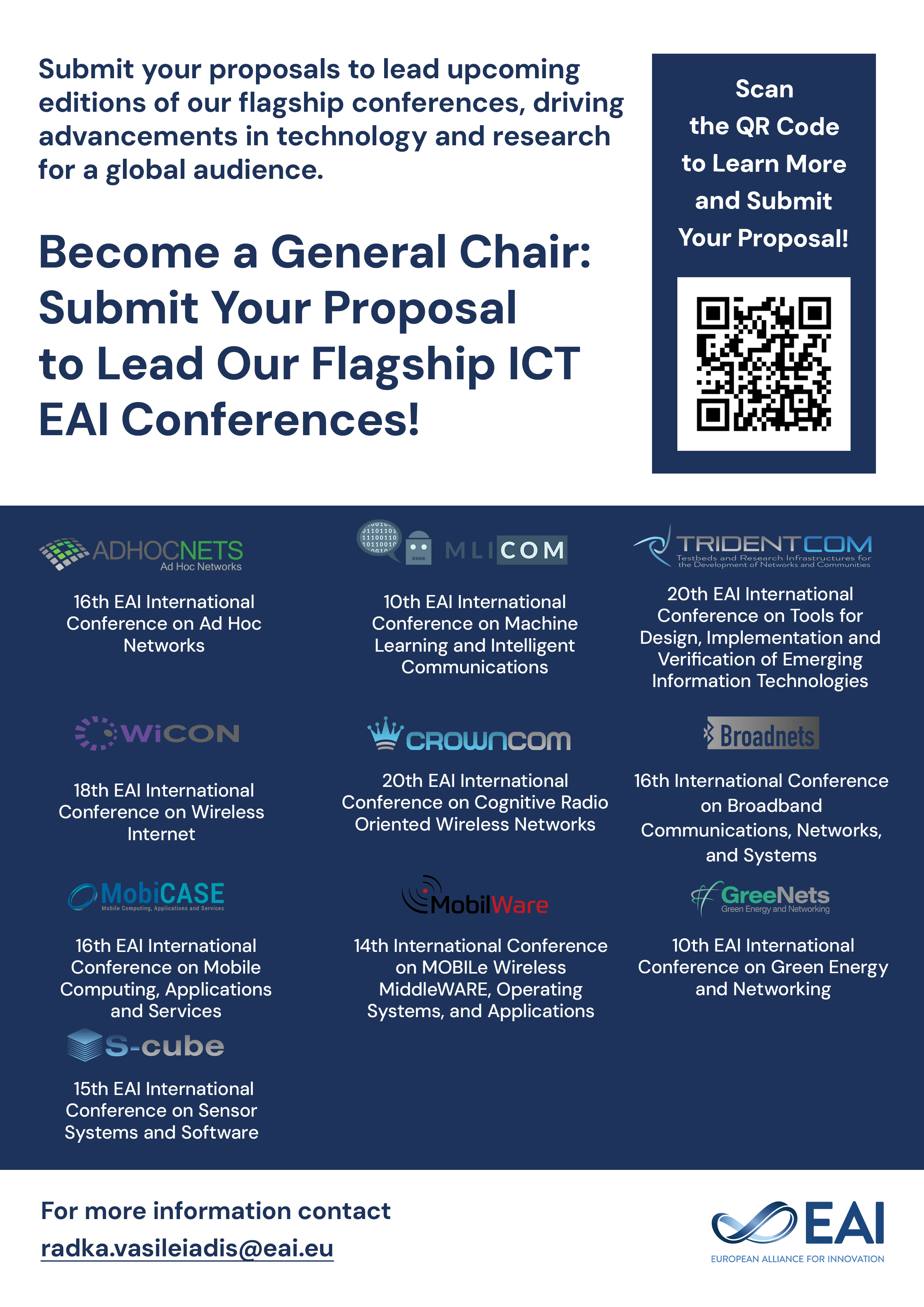
Research Article
An Accelerated PSO Based Self-organizing RBF Neural Network for Nonlinear System Identification and Modeling
136 downloads
@INPROCEEDINGS{10.1007/978-3-030-32388-2_63, author={Zohaib Ahmad and Cuili Yang and Junfei Qiao}, title={An Accelerated PSO Based Self-organizing RBF Neural Network for Nonlinear System Identification and Modeling}, proceedings={Machine Learning and Intelligent Communications. 4th International Conference, MLICOM 2019, Nanjing, China, August 24--25, 2019, Proceedings}, proceedings_a={MLICOM}, year={2019}, month={10}, keywords={Accelerated particle swarm optimization Radial basis function Nonlinear system modeling}, doi={10.1007/978-3-030-32388-2_63} }
- Zohaib Ahmad
Cuili Yang
Junfei Qiao
Year: 2019
An Accelerated PSO Based Self-organizing RBF Neural Network for Nonlinear System Identification and Modeling
MLICOM
Springer
DOI: 10.1007/978-3-030-32388-2_63
Abstract
In this paper, an accelerated particle swarm optimization (APSO) based radial basis function neural network (RBFNN) is designed for nonlinear system modeling. In APSO-RBFNN, the center, width of hidden neurons, weights of output layer and network size are optimized by using the APSO method. Two nonlinear system modeling experiments are used to illustrate the effectiveness of the proposed method. The simulation results show that the proposed method has obtained good performance in terms of network size and estimation accuracy.
Copyright © 2019–2024 ICST