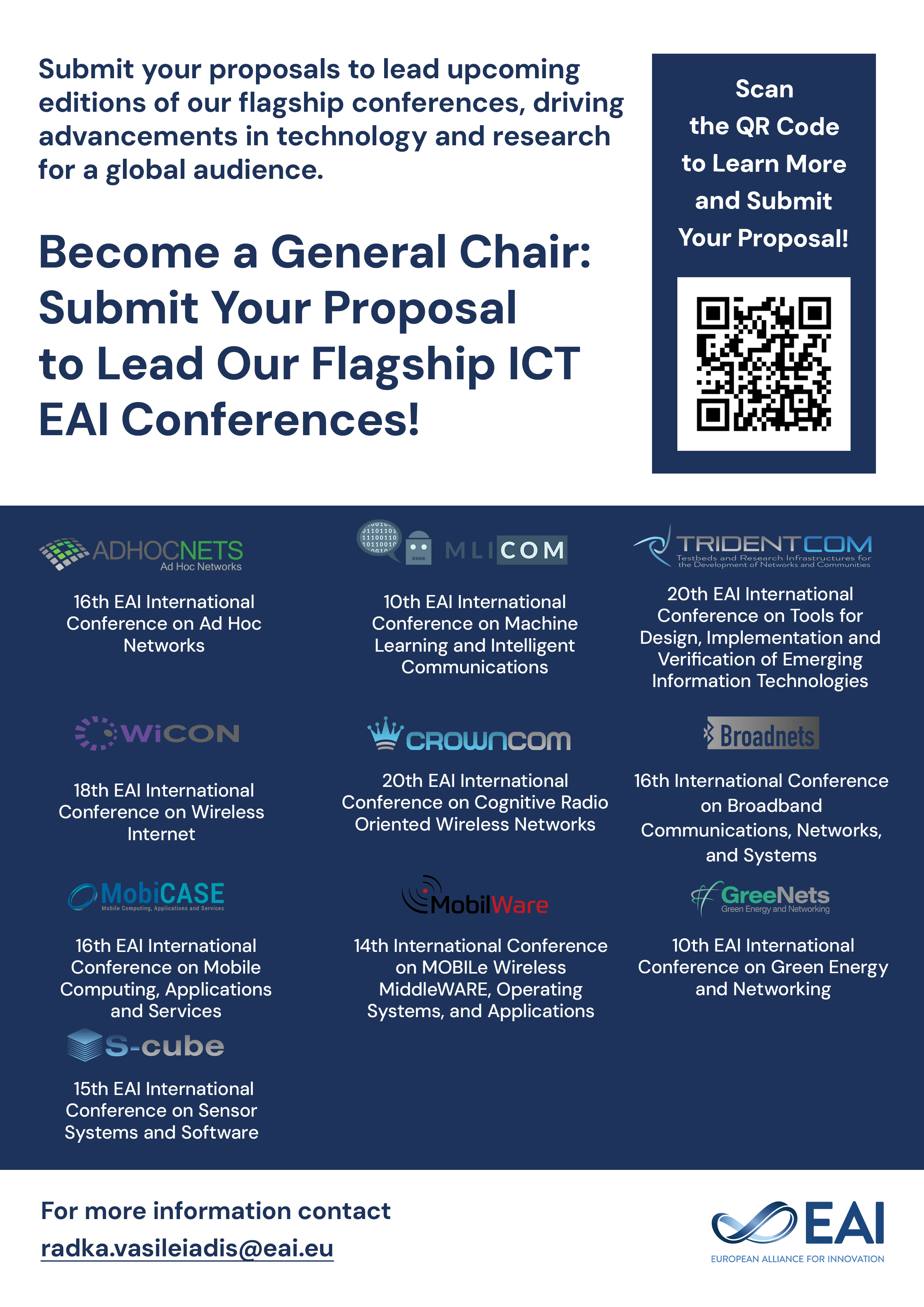
Research Article
Latent Flow Patterns Discovery by Dockless Bike Trajectory Data for Demand Analysis
@INPROCEEDINGS{10.1007/978-3-030-32388-2_54, author={Chao Ling and JingJing Gu and Ming Sun}, title={Latent Flow Patterns Discovery by Dockless Bike Trajectory Data for Demand Analysis}, proceedings={Machine Learning and Intelligent Communications. 4th International Conference, MLICOM 2019, Nanjing, China, August 24--25, 2019, Proceedings}, proceedings_a={MLICOM}, year={2019}, month={10}, keywords={Shared bikes DNN Graph clustering}, doi={10.1007/978-3-030-32388-2_54} }
- Chao Ling
JingJing Gu
Ming Sun
Year: 2019
Latent Flow Patterns Discovery by Dockless Bike Trajectory Data for Demand Analysis
MLICOM
Springer
DOI: 10.1007/978-3-030-32388-2_54
Abstract
The dockless shared bikes flourish as a new concept in recent years. It allows users to find bikes anywhere via a GPS-based mobile application, and flexible cycling and parking the bikes in the same way. From the bike trajectory data produced by Users, we can extract bike flow patterns for better urban planning and Point-of-Interest (POI) recommendation. In this paper, through conducting the spatio-temporal representations of bike activity acquired from bike trajectory logs, we first design a graph clustering model With sparsity constraints that combine time information to explore potential patterns of bike flow. Next, by comparing historical trajectory logs and POI information with the flow patterns, we dig out several typical categories of bike flow patterns, which can give suggestions for further urban planning and POI recommendation. Further, our experiments via Mobike trajectory data demonstrate the effectiveness of bike flow pattern discovery.