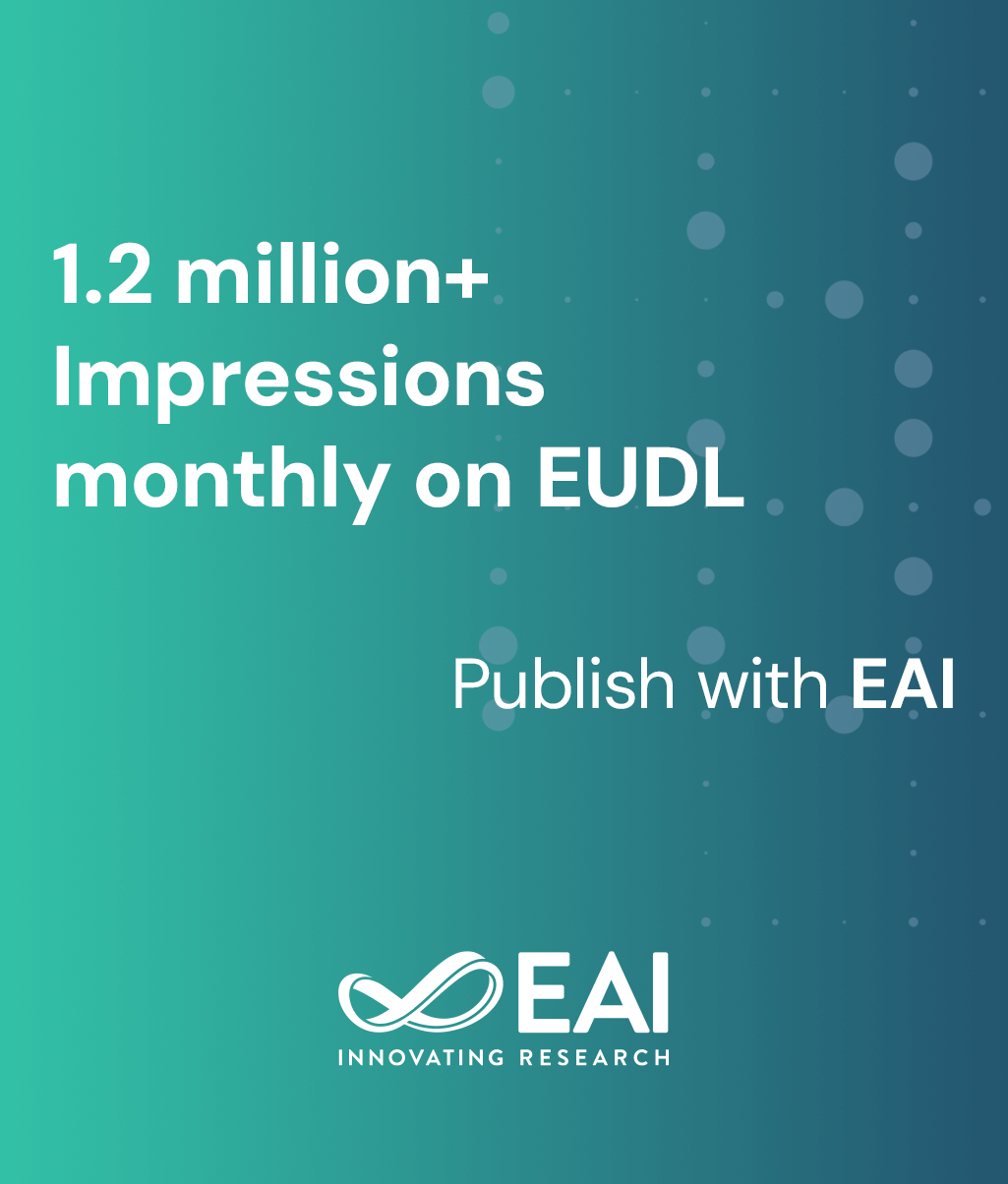
Research Article
Knowledge Graph Embedding Based on Hyperplane and Quantitative Credibility
@INPROCEEDINGS{10.1007/978-3-030-32388-2_50, author={Shuo Chen and Lin Qiao and Biqi Liu and Jue Bo and Yuanning Cui and Jing Li}, title={Knowledge Graph Embedding Based on Hyperplane and Quantitative Credibility}, proceedings={Machine Learning and Intelligent Communications. 4th International Conference, MLICOM 2019, Nanjing, China, August 24--25, 2019, Proceedings}, proceedings_a={MLICOM}, year={2019}, month={10}, keywords={Knowledge graph Time aware Representation learning Link Prediction}, doi={10.1007/978-3-030-32388-2_50} }
- Shuo Chen
Lin Qiao
Biqi Liu
Jue Bo
Yuanning Cui
Jing Li
Year: 2019
Knowledge Graph Embedding Based on Hyperplane and Quantitative Credibility
MLICOM
Springer
DOI: 10.1007/978-3-030-32388-2_50
Abstract
Knowledge representation learning is one of the research hotspots in the field of knowledge graph in recent years. How to improve the training algorithm of knowledge representation model and improve the accuracy of knowledge graph knowledge completion prediction is the main research goal in this field. Applying more implicit semantic information to model training is the primary means of improving accuracy. The traditional method does not consider the change of knowledge validity with time. So for this problem, we study the distribution law of quantitative changes of knowledge, design the model to simulate the quantitative changes of knowledge, put forward the concept of quantitative credibility, and apply it to the training algorithm of the model, and put forward A new learning method of knowledge representation QCHyTE. We compare the trained model with the best-recognized algorithms, and the results show that our improved algorithm greatly improves the prediction accuracy of the model.