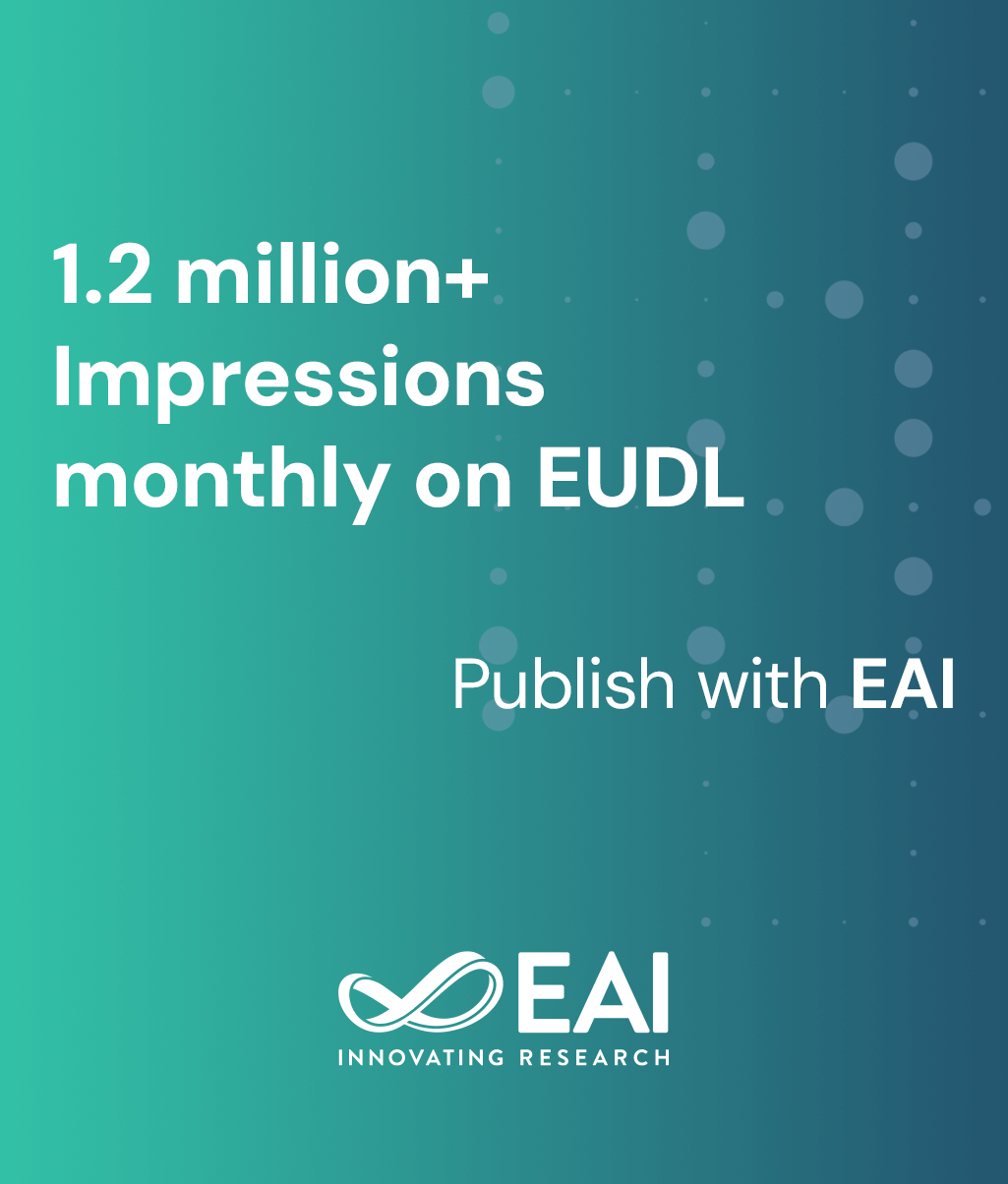
Research Article
Motion Classification Based on sEMG Signals Using Deep Learning
@INPROCEEDINGS{10.1007/978-3-030-32388-2_48, author={Shu Shen and Kang Gu and Xinrong Chen and Ruchuan Wang}, title={Motion Classification Based on sEMG Signals Using Deep Learning}, proceedings={Machine Learning and Intelligent Communications. 4th International Conference, MLICOM 2019, Nanjing, China, August 24--25, 2019, Proceedings}, proceedings_a={MLICOM}, year={2019}, month={10}, keywords={Surface electromyogram signal Deep learning Wearable device Motion recognition}, doi={10.1007/978-3-030-32388-2_48} }
- Shu Shen
Kang Gu
Xinrong Chen
Ruchuan Wang
Year: 2019
Motion Classification Based on sEMG Signals Using Deep Learning
MLICOM
Springer
DOI: 10.1007/978-3-030-32388-2_48
Abstract
Nowadays, surface electromyography (sEMG) signal plays an important role in helping physically disabled people during daily life. The development of electronic information technology has also led to the emergence of low-cost, multi-channel, wearable sEMG signal acquisition devices. Therefore, this paper proposes a new motion recognition model based on deep learning to improve the accuracy of motion recognition of sEMG signals. The model uses architecture including 6 convolutional layers and 6 pooling layers which enables the Batch Normalization layer to enhance network performance and prevent overfitting. In the experiment, NinaPro DB5 data set was used for training and testing. The data set consists of sEMG signal data collected by the double Myo armbands which contains data of 52 movements for 10 subjects. The results show that the accuracy of about 90% can be achieved when using 52 sEMG signal data from every single subject or all subjects.