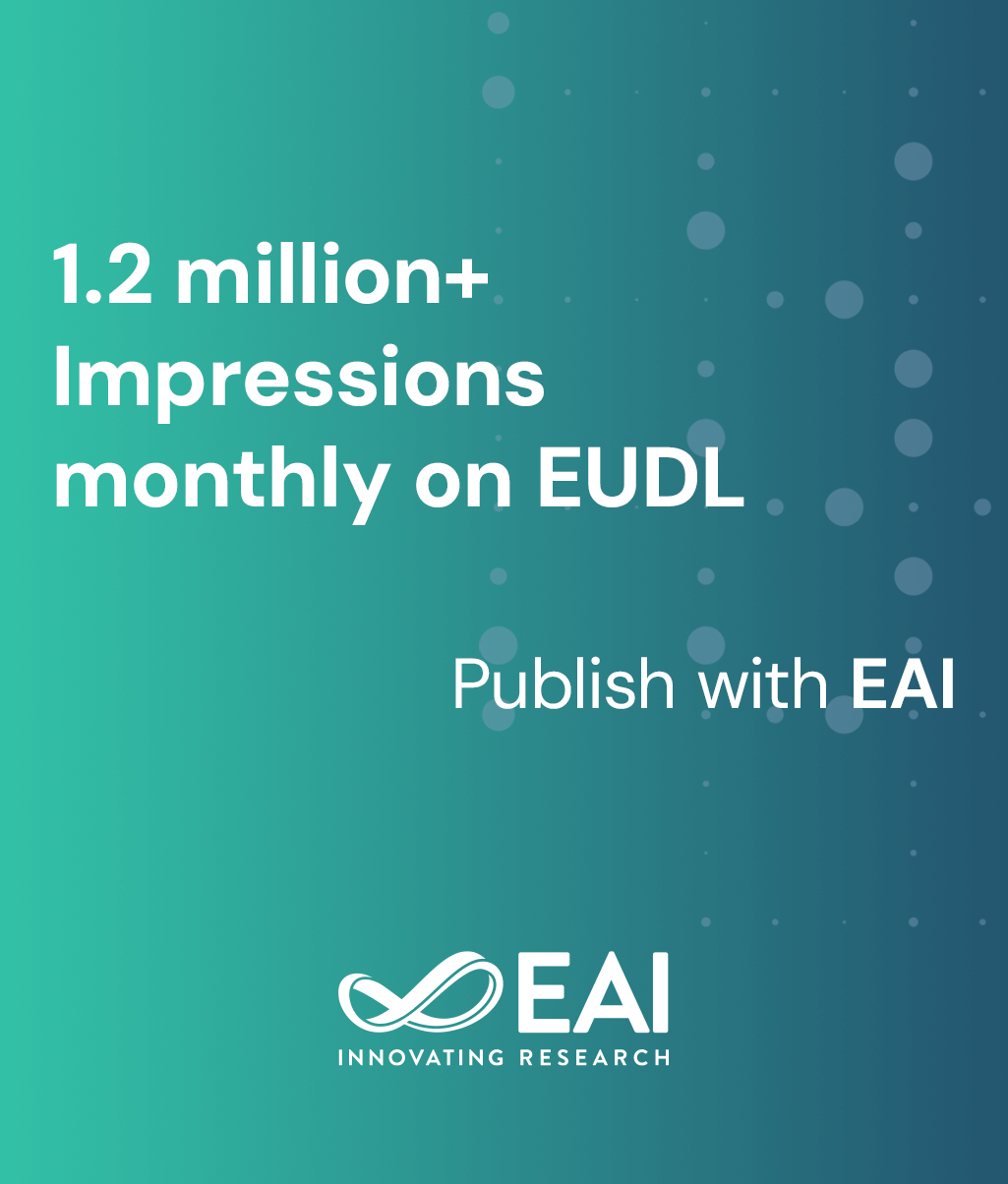
Research Article
An Efficient Federated Learning Scheme with Differential Privacy in Mobile Edge Computing
@INPROCEEDINGS{10.1007/978-3-030-32388-2_46, author={Jiale Zhang and Junyu Wang and Yanchao Zhao and Bing Chen}, title={An Efficient Federated Learning Scheme with Differential Privacy in Mobile Edge Computing}, proceedings={Machine Learning and Intelligent Communications. 4th International Conference, MLICOM 2019, Nanjing, China, August 24--25, 2019, Proceedings}, proceedings_a={MLICOM}, year={2019}, month={10}, keywords={Federated learning Mobile edge computing Deep neural network Differential privacy}, doi={10.1007/978-3-030-32388-2_46} }
- Jiale Zhang
Junyu Wang
Yanchao Zhao
Bing Chen
Year: 2019
An Efficient Federated Learning Scheme with Differential Privacy in Mobile Edge Computing
MLICOM
Springer
DOI: 10.1007/978-3-030-32388-2_46
Abstract
In this paper, we consider a mobile edge computing (MEC) system that multiple users participate in the federated learning protocol by jointly training a deep neural network (DNN) with their private training datasets. The main challenges of applying federated learning to MEC are: (1) it incurs tremendous computational cost by carrying out the deep neural network training phase on the resource-constraint mobile edge devices; (2) existing literature demonstrates that the parameters of a DNN trained on a dataset can be exploited to partially reconstruct the training samples in original dataset. To address the aforementioned issues, we introduce an efficiently private federated learning scheme in mobile edge computing, named FedMEC, with model partition technique and differential privacy method in this work. The experimental results demonstrate that our proposed FedMEC scheme can achieve high model accuracy under different perturbation strengths.