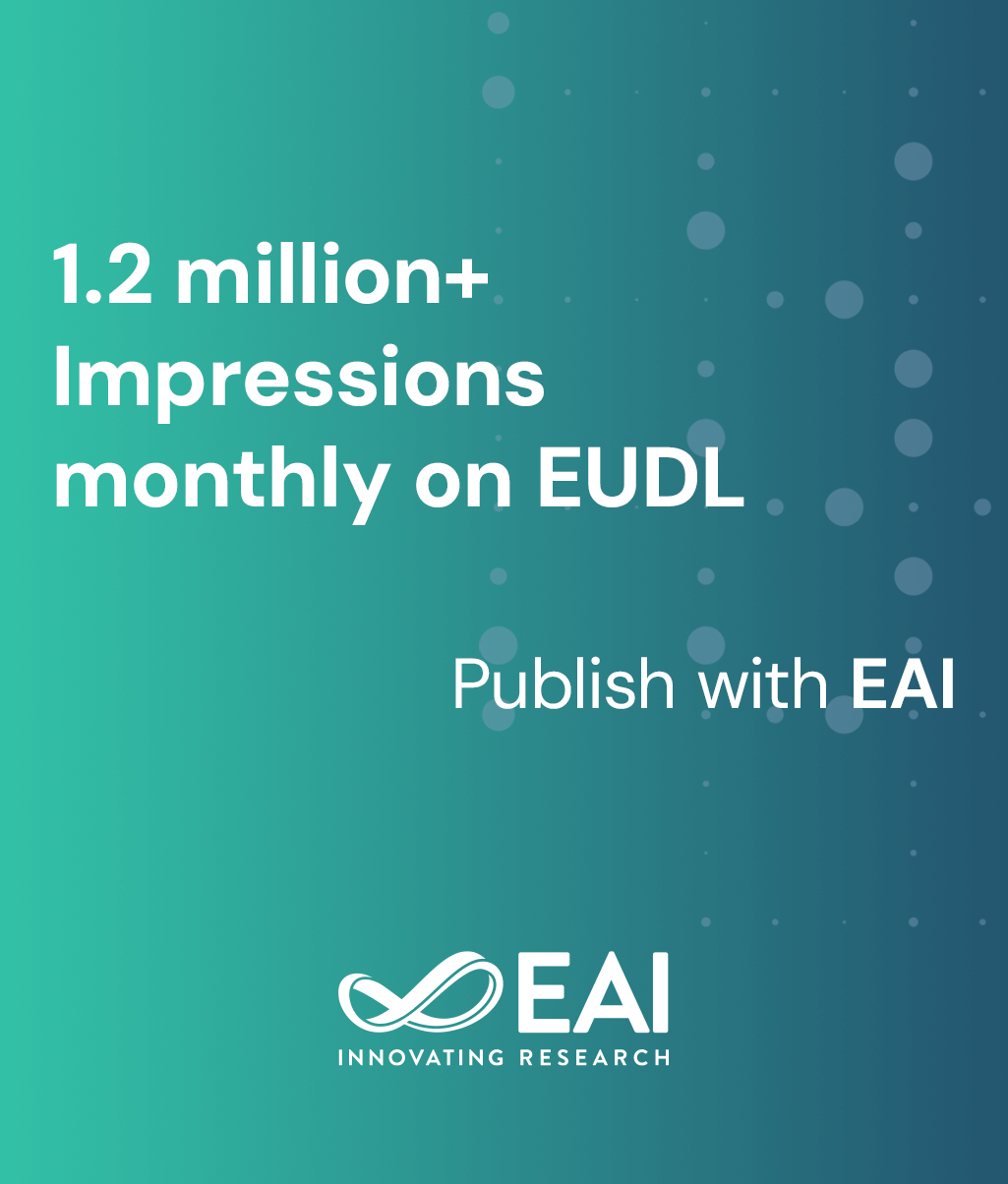
Research Article
Using LSTM GRU and Hybrid Models for Streamflow Forecasting
@INPROCEEDINGS{10.1007/978-3-030-32388-2_44, author={Abdullahi Muhammad and Xiaodong Li and Jun Feng}, title={Using LSTM GRU and Hybrid Models for Streamflow Forecasting}, proceedings={Machine Learning and Intelligent Communications. 4th International Conference, MLICOM 2019, Nanjing, China, August 24--25, 2019, Proceedings}, proceedings_a={MLICOM}, year={2019}, month={10}, keywords={LSTM MOPEX Recurrent neural networks Streamflow forecasting}, doi={10.1007/978-3-030-32388-2_44} }
- Abdullahi Muhammad
Xiaodong Li
Jun Feng
Year: 2019
Using LSTM GRU and Hybrid Models for Streamflow Forecasting
MLICOM
Springer
DOI: 10.1007/978-3-030-32388-2_44
Abstract
Forecasting streamflow discharge have economic impact as well as reducing the effects of floods in flood prone regimes by presenting early warning. To minimize it’s effects in these regimes, a powerful class of machine learning algorithms called long short-term memory (LSTM) and gated recurrent units (GRU) models, which have become popular in time series forecasting, because they are explicitly designed to avoid the long-term dependency problems is applied. LSTM and GRU models have also demonstrated their capacity in sequence modelling, speech recognition and streamflow forecasting. In this paper we proposed a hybrid model for streamflow forecasting using 35 consecutive years Model Parameter Estimation Experiment (MOPEX) data set of ten basins having different basin characteristics from different climatic regions in United States. The proposed hybrid model’s performance is compared to the conventional LSTM and GRU models. Our experiments on the 10 MOPEX’s river basins demonstrate that, although the proposed hybrid model outperforms conventional LSTM with respect to streamflow forecasting, but the performance is almost same with GRU and is therefore highly recommended as an efficient and reliable approach in hydrological fields.