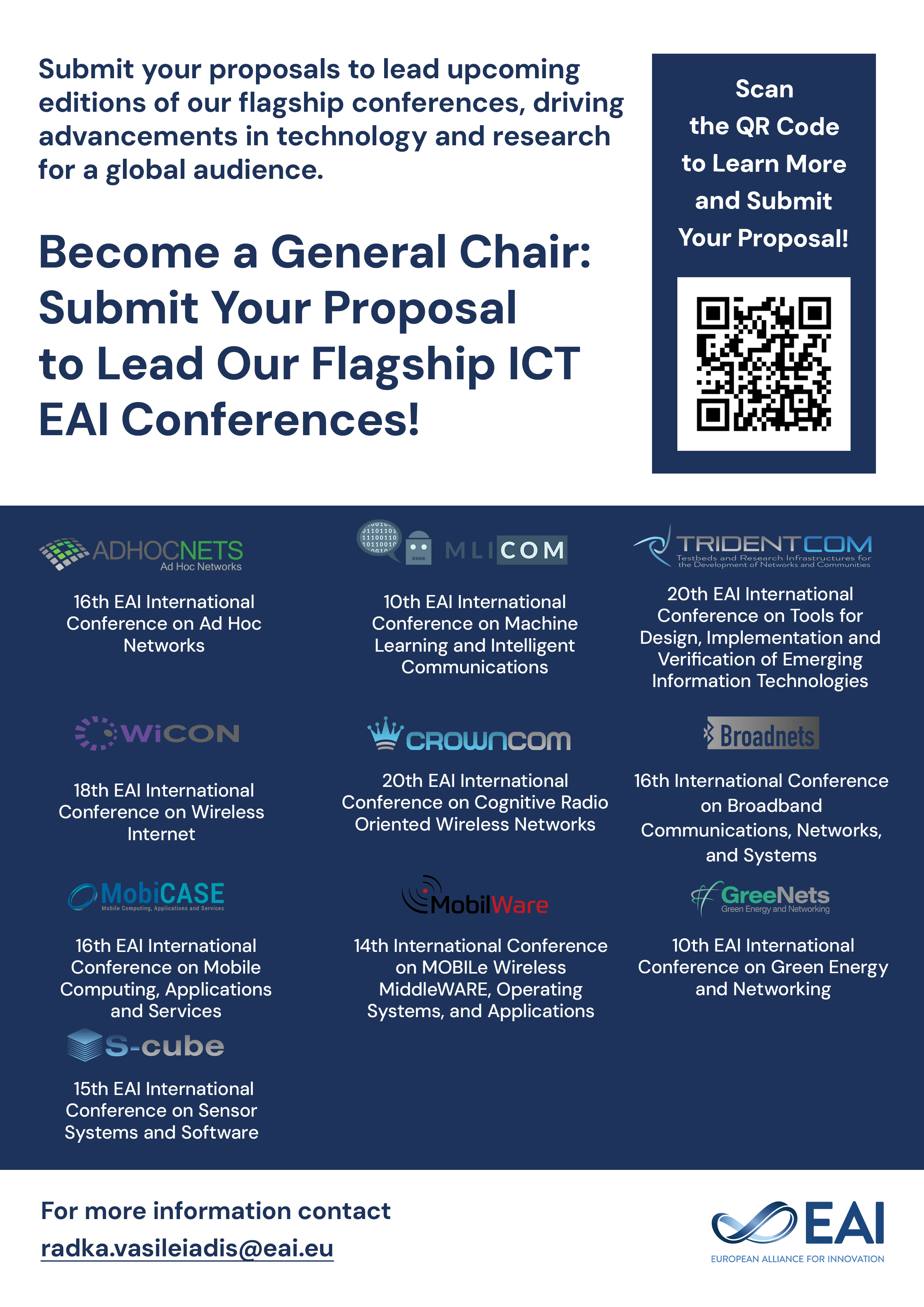
Research Article
Transaction Cost Analysis via Label-Spreading Learning
@INPROCEEDINGS{10.1007/978-3-030-32388-2_38, author={Pangjing Wu and Xiaodong Li}, title={Transaction Cost Analysis via Label-Spreading Learning}, proceedings={Machine Learning and Intelligent Communications. 4th International Conference, MLICOM 2019, Nanjing, China, August 24--25, 2019, Proceedings}, proceedings_a={MLICOM}, year={2019}, month={10}, keywords={Label-spreading Semi-supervised learning Algorithm trading}, doi={10.1007/978-3-030-32388-2_38} }
- Pangjing Wu
Xiaodong Li
Year: 2019
Transaction Cost Analysis via Label-Spreading Learning
MLICOM
Springer
DOI: 10.1007/978-3-030-32388-2_38
Abstract
When the investment institution analyzes the transaction cost of stock orders, it is costly to obtain the transaction cost of the stock orders by trading it. In contrast, many simulated trading orders cannot get the exact transaction cost. Due to the lack of enough labeled data, it is usually hard to use a supervised learner to estimate accurate transaction cost of stock orders. Label-spreading, a graph-based semi-supervised learner, can integrate a small number of labeled real orders and a large number of unlabeled simulated orders, and train a learner simultaneously. Using a RBF kernel, the learner constructs a graph structure through the spatial similarity measure between the transaction cost samples, and propagates the label through edges of graph in high-dimensional space. The results of experiments show that the label-spreading learner can make full use of the information of unlabeled data to improve classification of transaction cost.