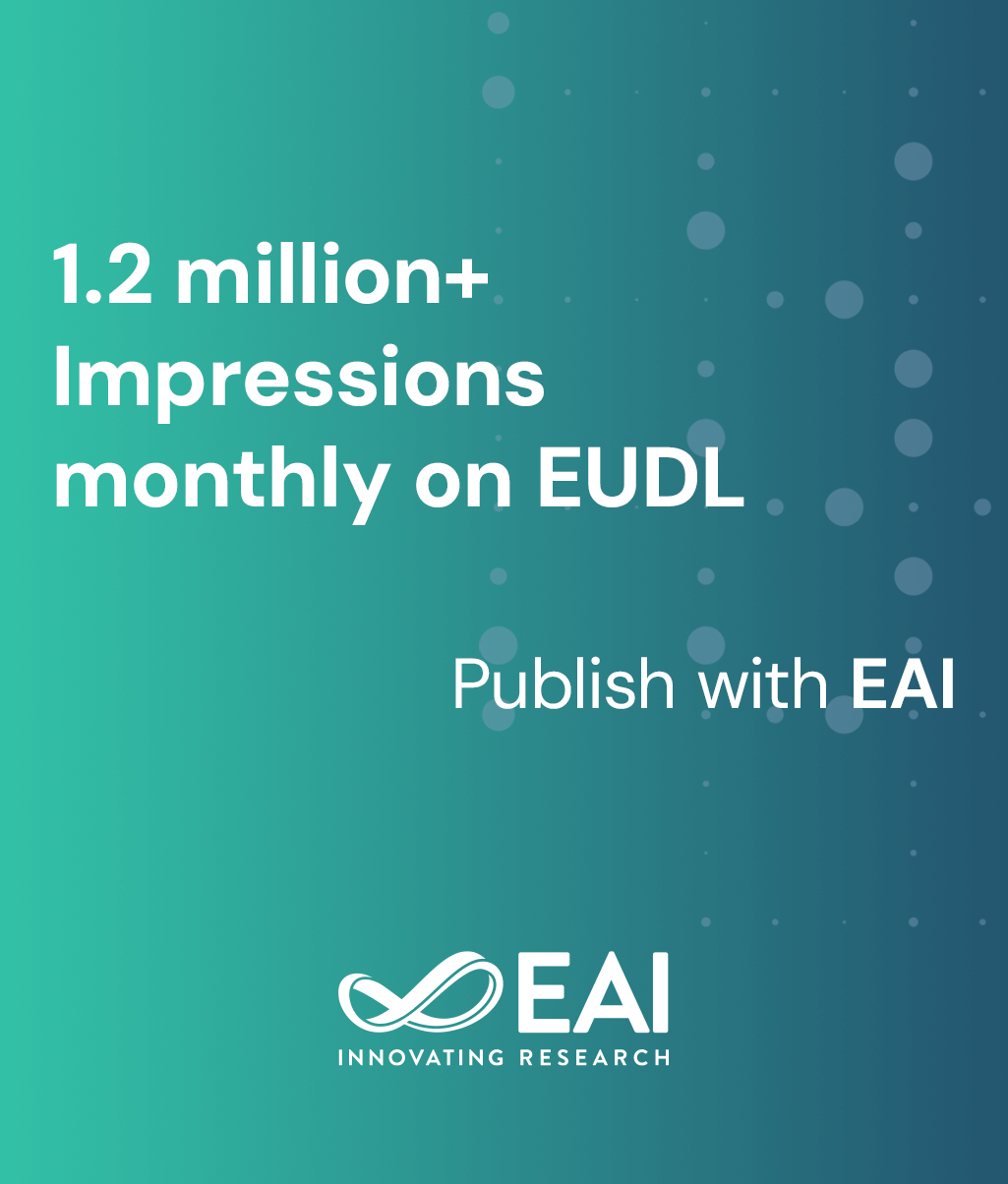
Research Article
A Bayesian Method for Link Prediction with Considering Path Information
@INPROCEEDINGS{10.1007/978-3-030-32388-2_31, author={Suyuan Zhang and Lunbo Li and Cunlai Pu and Siyuan Zhou}, title={A Bayesian Method for Link Prediction with Considering Path Information}, proceedings={Machine Learning and Intelligent Communications. 4th International Conference, MLICOM 2019, Nanjing, China, August 24--25, 2019, Proceedings}, proceedings_a={MLICOM}, year={2019}, month={10}, keywords={Link prediction Path information Bayesian method Structural similarity}, doi={10.1007/978-3-030-32388-2_31} }
- Suyuan Zhang
Lunbo Li
Cunlai Pu
Siyuan Zhou
Year: 2019
A Bayesian Method for Link Prediction with Considering Path Information
MLICOM
Springer
DOI: 10.1007/978-3-030-32388-2_31
Abstract
Predicting links among nodes in the network is an interesting and practical problem. Many link prediction methods based on local or global topology alone have been proposed. There is a need to combine these two types of methods to further improve the prediction performance. In line with this direction, we study the link prediction problem based on the Bayesian method and propose a new link prediction method, i.e., path-based Bayesian (PB) method. In this prediction method, we give the definition of clustering coefficients of paths and use it to quantify the contribution of paths to link generation. Then, we propose a new link prediction method by combining the clustering coefficient of paths and Bayesian theory. Simulation results on real-world networks show that our prediction method has higher prediction accuracy than the mainstream methods.