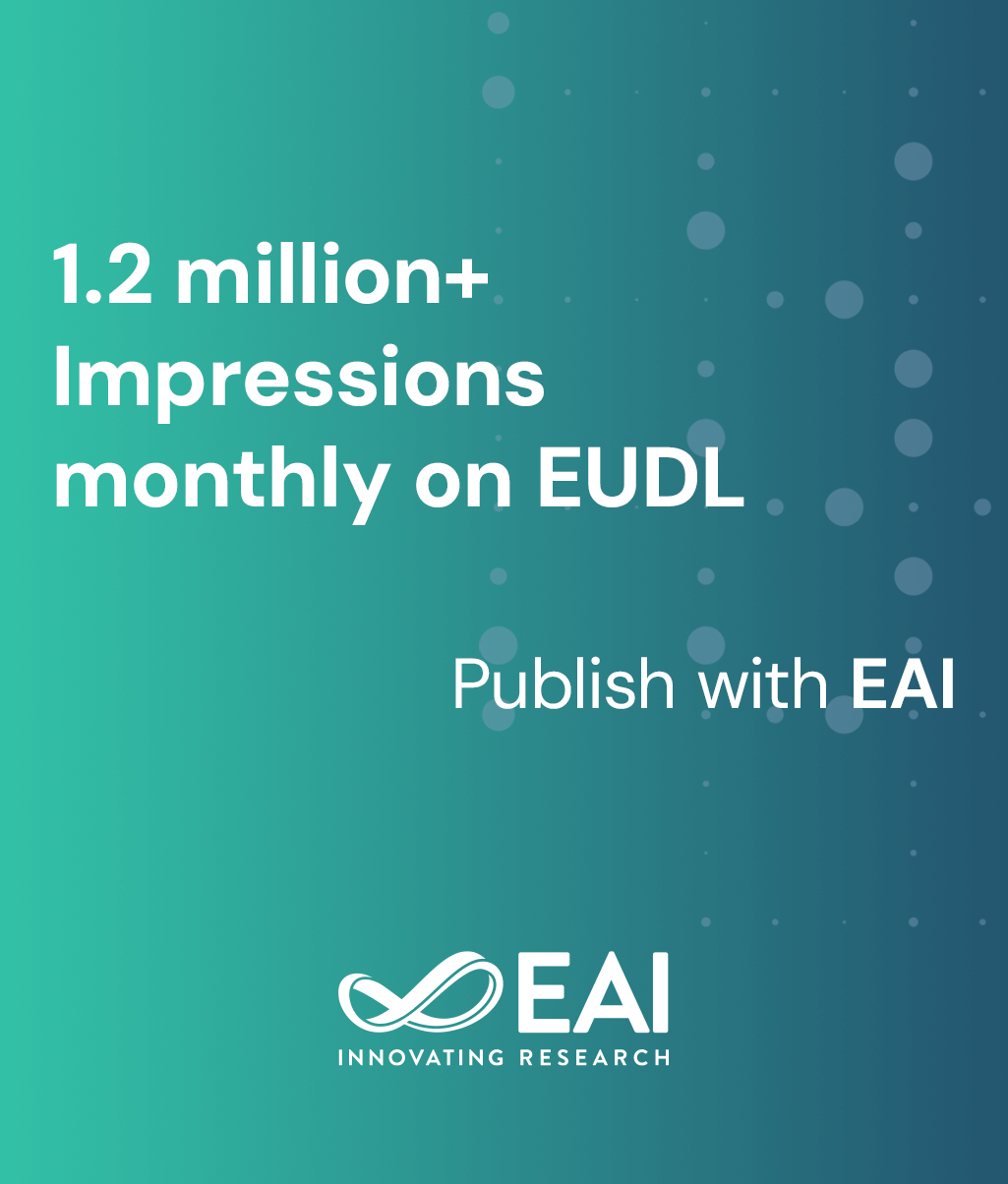
Research Article
Data Cleaning Based on Multi-sensor Spatiotemporal Correlation
@INPROCEEDINGS{10.1007/978-3-030-32388-2_20, author={Baozhu Shao and Chunhe Song and Zhongfeng Wang and Zhexi Li and Shimao Yu and Peng Zeng}, title={Data Cleaning Based on Multi-sensor Spatiotemporal Correlation}, proceedings={Machine Learning and Intelligent Communications. 4th International Conference, MLICOM 2019, Nanjing, China, August 24--25, 2019, Proceedings}, proceedings_a={MLICOM}, year={2019}, month={10}, keywords={Data cleaning Spatiotemporal correlation Sensor networks}, doi={10.1007/978-3-030-32388-2_20} }
- Baozhu Shao
Chunhe Song
Zhongfeng Wang
Zhexi Li
Shimao Yu
Peng Zeng
Year: 2019
Data Cleaning Based on Multi-sensor Spatiotemporal Correlation
MLICOM
Springer
DOI: 10.1007/978-3-030-32388-2_20
Abstract
Sensor-based condition monitoring systems are becoming an important part of modern industry. However, the data collected from sensor nodes are usually unreliable and inaccurate. It is very critical to clean the sensor data before using them to detect actual events occurred in the physical world. Popular data cleaning methods, such as moving average and stacked denoise autoencoder, cannot meet the requirements of accuracy, energy efficiency or computation limitation in many sensor related applications. In this paper, we propose a data cleaning method based on multi-sensor spatiotemporal correlation. Specifically, we find out and repair the abnormal data according to the correlation of sensor data in adjacent time and adjacent space. Real data based simulation shows the effectiveness of our proposed method.