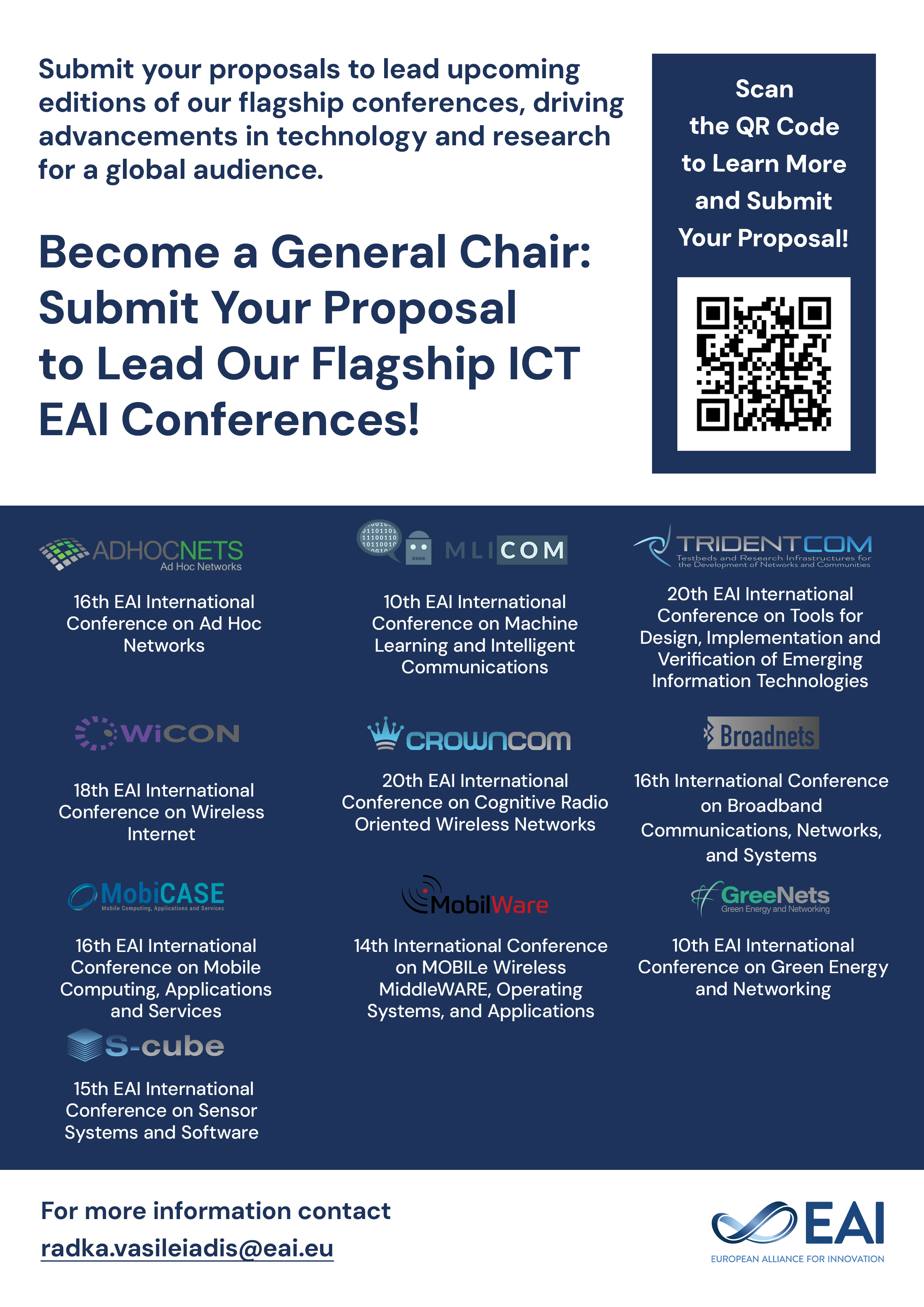
Research Article
A Q-Learning-Based Channel Selection and Data Scheduling Approach for High-Frequency Communications in Jamming Environment
@INPROCEEDINGS{10.1007/978-3-030-32388-2_13, author={Wen Li and Yuhua Xu and Qiuju Guo and Yuli Zhang and Dianxiong Liu and Yangyang Li and Wei Bai}, title={A Q-Learning-Based Channel Selection and Data Scheduling Approach for High-Frequency Communications in Jamming Environment}, proceedings={Machine Learning and Intelligent Communications. 4th International Conference, MLICOM 2019, Nanjing, China, August 24--25, 2019, Proceedings}, proceedings_a={MLICOM}, year={2019}, month={10}, keywords={Anti-jamming Dynamic spectrum access Q-learning High-frequency(HF) communication Markov decision process (MDP)}, doi={10.1007/978-3-030-32388-2_13} }
- Wen Li
Yuhua Xu
Qiuju Guo
Yuli Zhang
Dianxiong Liu
Yangyang Li
Wei Bai
Year: 2019
A Q-Learning-Based Channel Selection and Data Scheduling Approach for High-Frequency Communications in Jamming Environment
MLICOM
Springer
DOI: 10.1007/978-3-030-32388-2_13
Abstract
The existence of jammer and the limited buffer space bring major challenge to data transmission efficiency in high-frequency (HF) commuication. The data transmission problem of how to select transmission strategy with multi-channel and different buffer states to maximize the system throughput is studied in this paper. We model the data transmission problem as a Makov decision process (MDP). Then, a modified Q-learning with additional value is proposed to help transmitter to learn the appropriate strategy and improve the system throughput. The simulation results show the proposed Q-learning algorithm can converge to the optimal Q value. Simultaneously, the QL algorithm compared with the sensing algorithm has better system throughput and less packet loss.