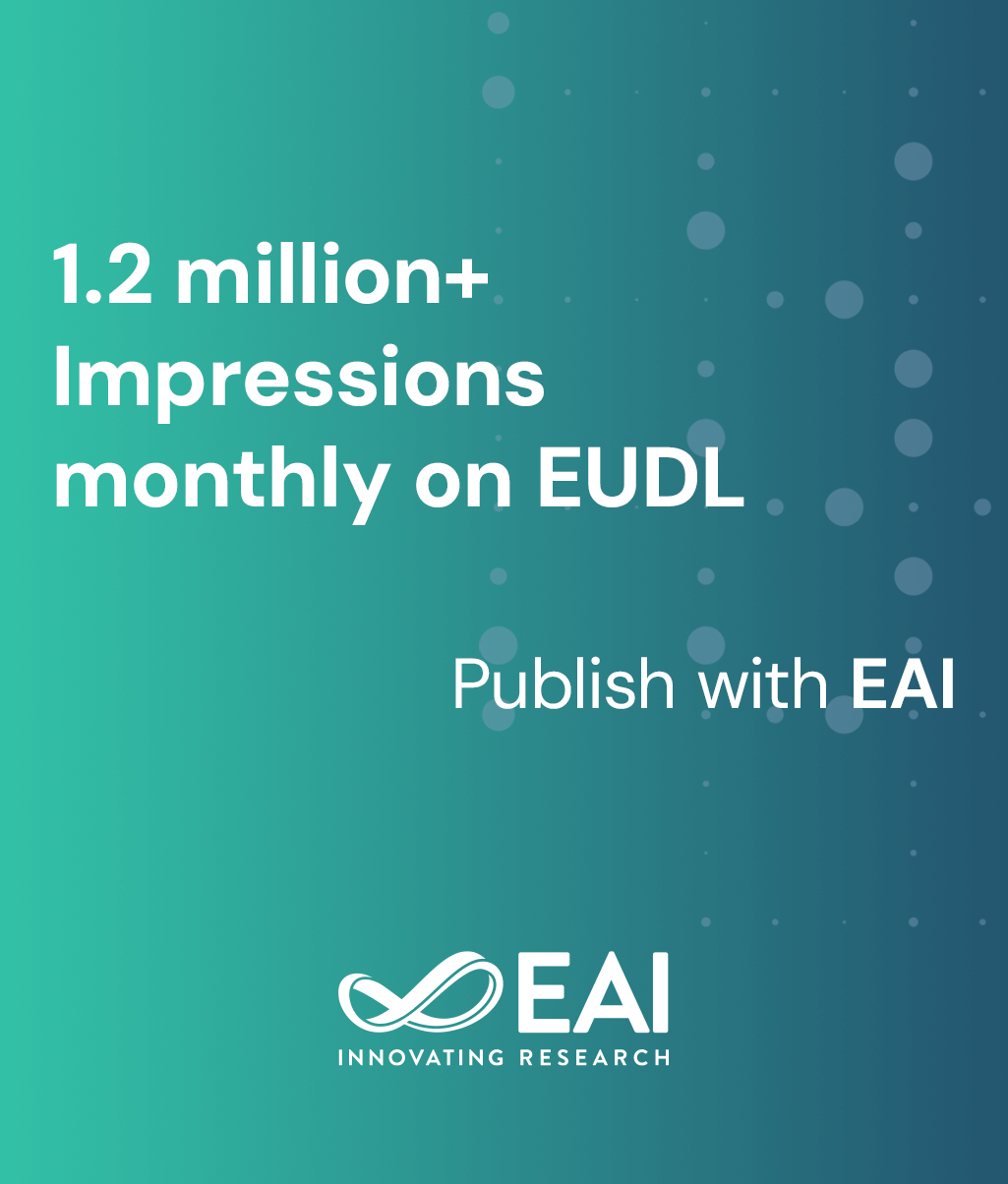
Research Article
High-Dimensional Data Anomaly Detection Framework Based on Feature Extraction of Elastic Network
@INPROCEEDINGS{10.1007/978-3-030-32388-2_1, author={Yang Shen and Jue Bo and KeXin Li and Shuo Chen and Lin Qiao and Jing Li}, title={High-Dimensional Data Anomaly Detection Framework Based on Feature Extraction of Elastic Network}, proceedings={Machine Learning and Intelligent Communications. 4th International Conference, MLICOM 2019, Nanjing, China, August 24--25, 2019, Proceedings}, proceedings_a={MLICOM}, year={2019}, month={10}, keywords={Elastic network Feature extraction Anomaly detection High-dimensional data Data mining}, doi={10.1007/978-3-030-32388-2_1} }
- Yang Shen
Jue Bo
KeXin Li
Shuo Chen
Lin Qiao
Jing Li
Year: 2019
High-Dimensional Data Anomaly Detection Framework Based on Feature Extraction of Elastic Network
MLICOM
Springer
DOI: 10.1007/978-3-030-32388-2_1
Abstract
Although appropriate feature extraction can improve the performance of anomaly detection, it is a challenging task due to the complex interaction between features, the mixture of irrelevant features and relevant features, and the unavailability of data tags. When conventional anomaly detection methods deal with the problem of anomaly detection of high dimensional data, the performance of anomaly detection will be degraded due to the existence of irrelevant features. This paper proposed a method of feature extraction and anomaly detection for high dimensional data based on elastic network, which can filter irrelevant features and improve the accuracy and efficiency of anomaly detection. In this paper, an outlier scoring method was used to score the outliers of the original data, and then outliers and the original data were input into the elastic network for sparse regression. After feature extraction of elastic network, those irrelevant features to abnormal data are ignored, thus reducing the dimension of data. Finally, high-dimensional data are detected efficiently according to extracted features. In the experimental stage, we used the high-dimensional anomaly dataset provided by ODDS to detect the performance of the proposed method based on AUC detection accuracy, ROC curve, feature number, convergence speed and other indicators. The results show that the proposed method not only can effectively extract the features related to high-dimensional anomaly data, but also the detection accuracy of outliers has been greatly improved.