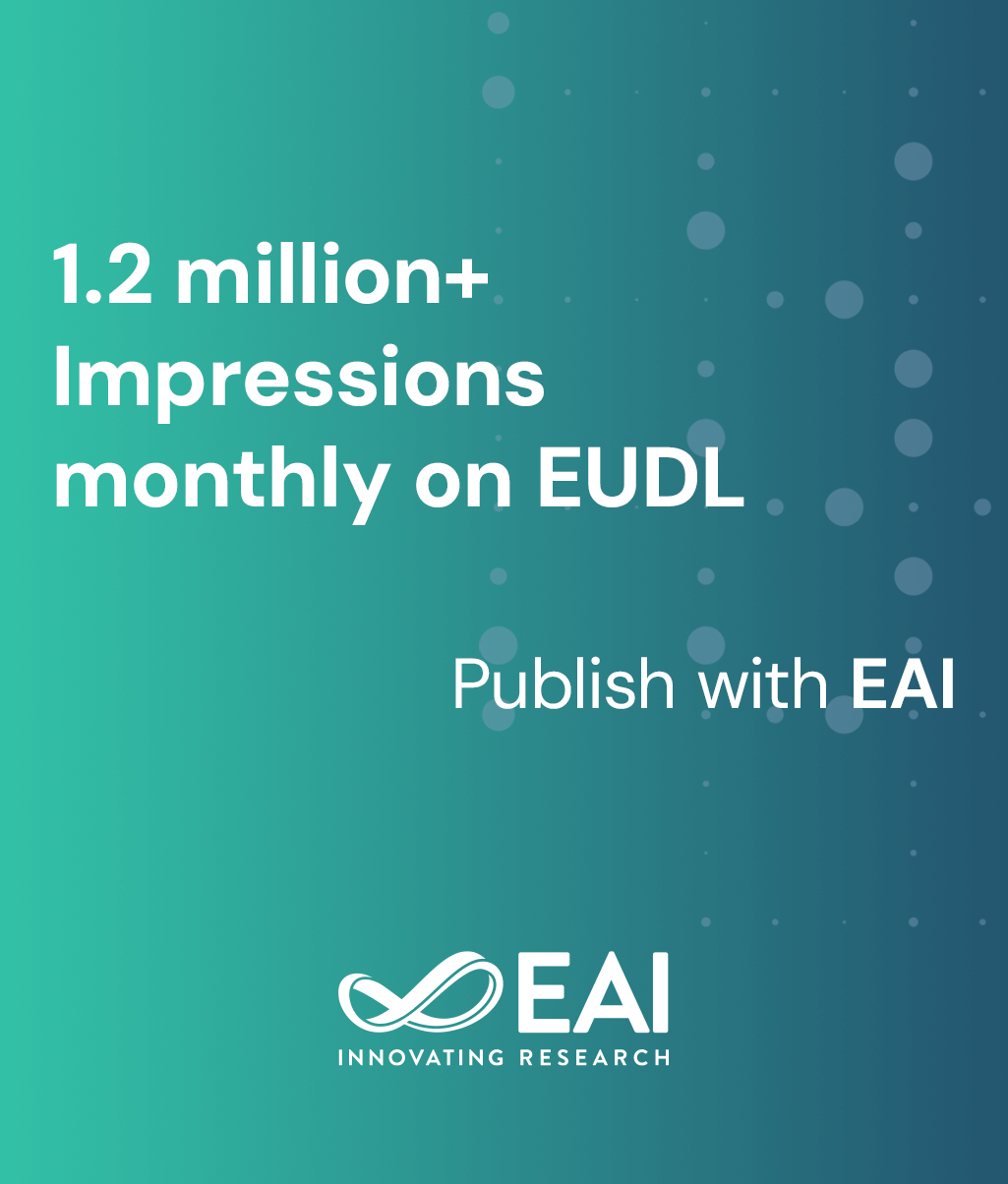
Research Article
A Study of RNN Based Online Handwritten Uyghur Word Recognition Using Different Word Transcriptions
@INPROCEEDINGS{10.1007/978-3-030-32216-8_50, author={Wujiahemaiti Simayi and Mayire Ibrayim and Askar Hamdulla}, title={A Study of RNN Based Online Handwritten Uyghur Word Recognition Using Different Word Transcriptions}, proceedings={Simulation Tools and Techniques. 11th International Conference, SIMUtools 2019, Chengdu, China, July 8--10, 2019, Proceedings}, proceedings_a={SIMUTOOLS}, year={2019}, month={10}, keywords={Online handwriting recognition Recurrent neural networks Connectionist temporal classification Uyghur word transcription}, doi={10.1007/978-3-030-32216-8_50} }
- Wujiahemaiti Simayi
Mayire Ibrayim
Askar Hamdulla
Year: 2019
A Study of RNN Based Online Handwritten Uyghur Word Recognition Using Different Word Transcriptions
SIMUTOOLS
Springer
DOI: 10.1007/978-3-030-32216-8_50
Abstract
Recurrent neural networks-RNN based online handwriting Uyghur word recognition experiments are conducted applying connectionist temporal classification in this paper. Handwritten trajectory is fed to the network without explicit or implicit character segmentation. The network is trained to transcribe the input word trajectory to a string of characters directly. According to the writing characteristics of Uyghur, experiments are designed using two Unicode word transcriptions respectively based on 32+2 basic character types and 128 specific character forms to represent a word. The training process and recognition results based on same network architecture show that both transcription methods are applicable. The word transcription system using basic 34 character types showed better performance than the one using 128 specific character forms in our experiments. 13.96%, 14.73% character error rates (CER) have been observed respectively for char34 system and char128 system.