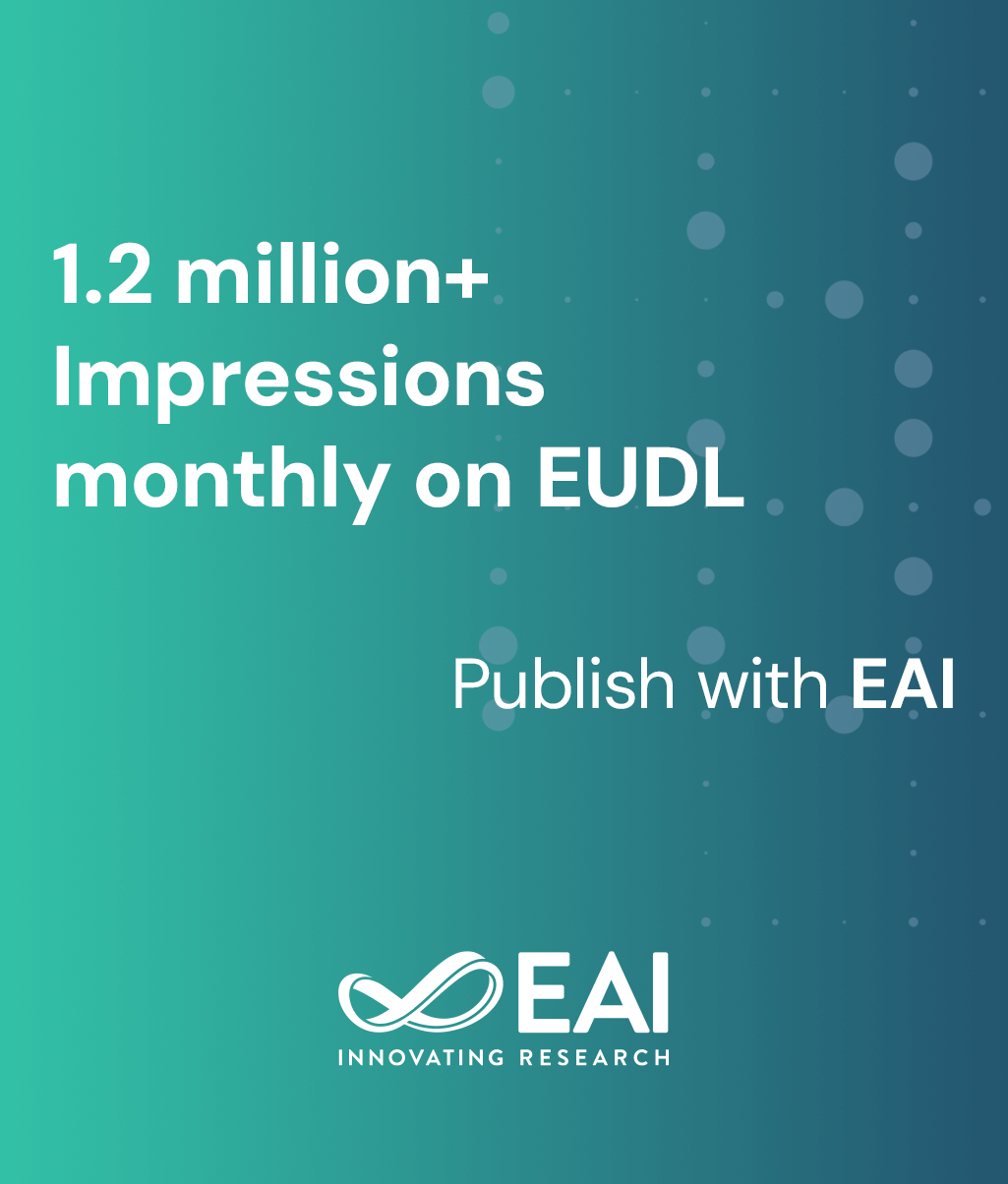
Research Article
Network Traffic Model with Multi-fractal Discrete Wavelet Transform in Power Telecommunication Access Networks
@INPROCEEDINGS{10.1007/978-3-030-32216-8_5, author={Yi Lu and Huan Li and Bin Lu and Yun Zhao and Dongdong Wang and Xiaoli Gong and Xin Wei}, title={Network Traffic Model with Multi-fractal Discrete Wavelet Transform in Power Telecommunication Access Networks}, proceedings={Simulation Tools and Techniques. 11th International Conference, SIMUtools 2019, Chengdu, China, July 8--10, 2019, Proceedings}, proceedings_a={SIMUTOOLS}, year={2019}, month={10}, keywords={Network traffic Multi-fractal Discrete wavelet transform Power telecommunication access networks}, doi={10.1007/978-3-030-32216-8_5} }
- Yi Lu
Huan Li
Bin Lu
Yun Zhao
Dongdong Wang
Xiaoli Gong
Xin Wei
Year: 2019
Network Traffic Model with Multi-fractal Discrete Wavelet Transform in Power Telecommunication Access Networks
SIMUTOOLS
Springer
DOI: 10.1007/978-3-030-32216-8_5
Abstract
With the development of communication networks, a lot of new applications emerge in the power telecommunication access networks, which have many new features and properties of the network traffic. These features are important for modeling the network traffic in the network-level. This paper propose a new feature extraction and network traffic model method. Firstly, we analyze the features of network traffic in time-frequency domain. Then, we use discrete wavelet transform to exploit the features of network traffic in the time domain and frequency domain. We run multi-fractal discrete wavelet transform (MDWT) for network traffic to decompose them into different frequency component and train an artificial neural network to predict the low- and high-frequency components of network traffic, and use them to reconstruct the network traffic. Finally, in order to validate our network traffic model, we conduct the network traffic prediction on the actual data. Simulation results show that our approach is feasible.