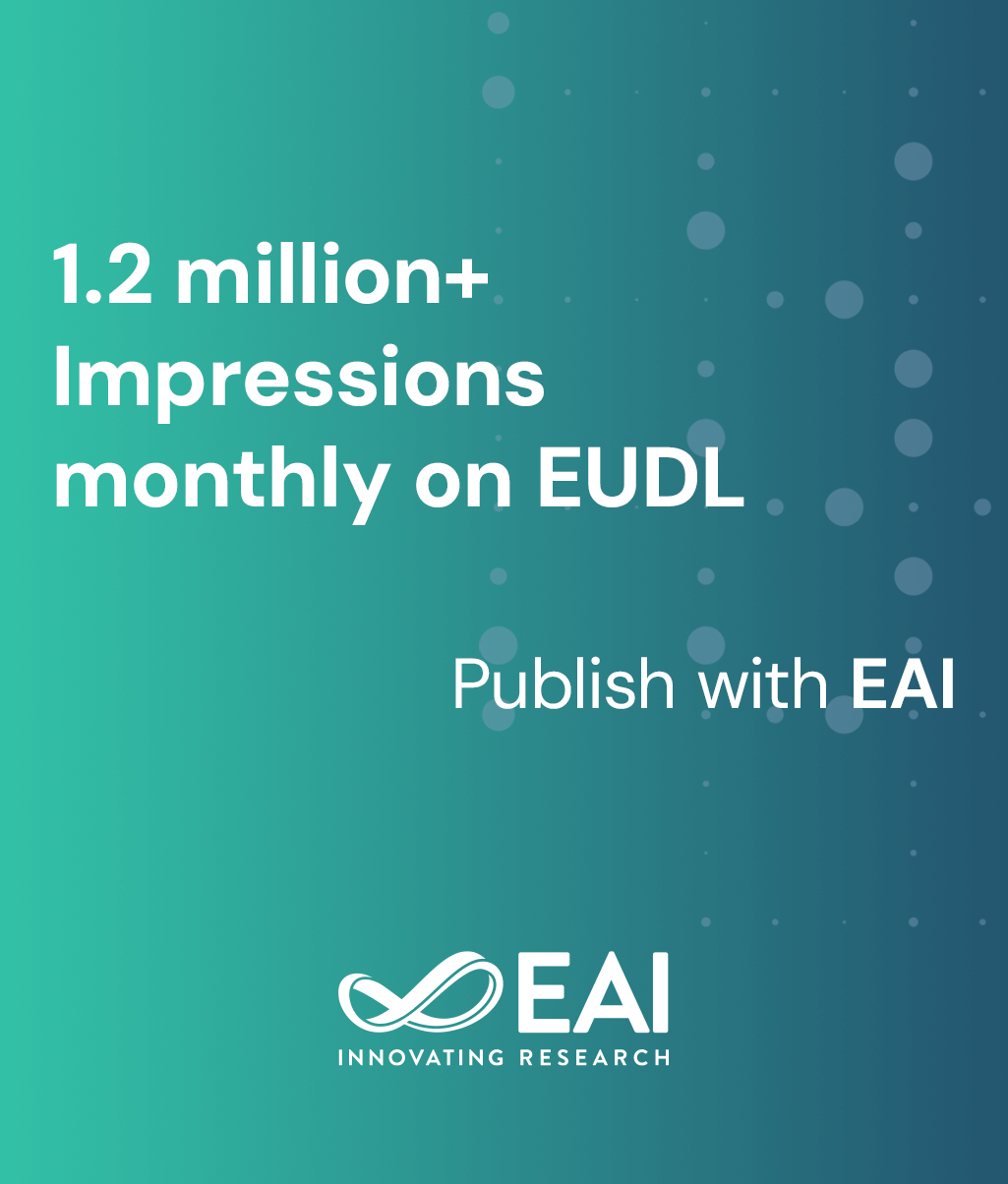
Research Article
Matrix-Variate Restricted Boltzmann Machine Classification Model
@INPROCEEDINGS{10.1007/978-3-030-32216-8_47, author={Jinghua Li and Pengyu Tian and Dehui Kong and Lichun Wang and Shaofan Wang and Baocai Yin}, title={Matrix-Variate Restricted Boltzmann Machine Classification Model}, proceedings={Simulation Tools and Techniques. 11th International Conference, SIMUtools 2019, Chengdu, China, July 8--10, 2019, Proceedings}, proceedings_a={SIMUTOOLS}, year={2019}, month={10}, keywords={ClassMVRBM MVRBM RBM}, doi={10.1007/978-3-030-32216-8_47} }
- Jinghua Li
Pengyu Tian
Dehui Kong
Lichun Wang
Shaofan Wang
Baocai Yin
Year: 2019
Matrix-Variate Restricted Boltzmann Machine Classification Model
SIMUTOOLS
Springer
DOI: 10.1007/978-3-030-32216-8_47
Abstract
Recently, Restricted Boltzmann Machine (RBM) has demonstrated excellent capacity of modelling vector variable. A variant of RBM, Matrix-variate Restricted Boltzmann Machine (MVRBM), extends the ability of RBM and is able to model matrix-variate data directly without vectorized process. However, MVRBM is still an unsupervised generative model, and is usually used to feature extraction or initialization of deep neural network. When MVRBM is used to classify, additional classifiers are necessary. This paper proposes a Matrix-variate Restricted Boltzmann Machine Classification Model (ClassMVRBM) to classify 2D data directly. In the novel ClassMVRBM, classification constraint is introduced to MVRBM. On one hand, the features extracted by MVRBM are more discriminative, on the other hand, the proposed model can be directly used to classify. Experiments on some publicly available databases demonstrate that the classification performance of ClassMVRBM has been largely improved, resulting in higher image classification accuracy than conventional unsupervised RBM, its variants and Restricted Boltzmann Machine Classification Model (ClassRBM).