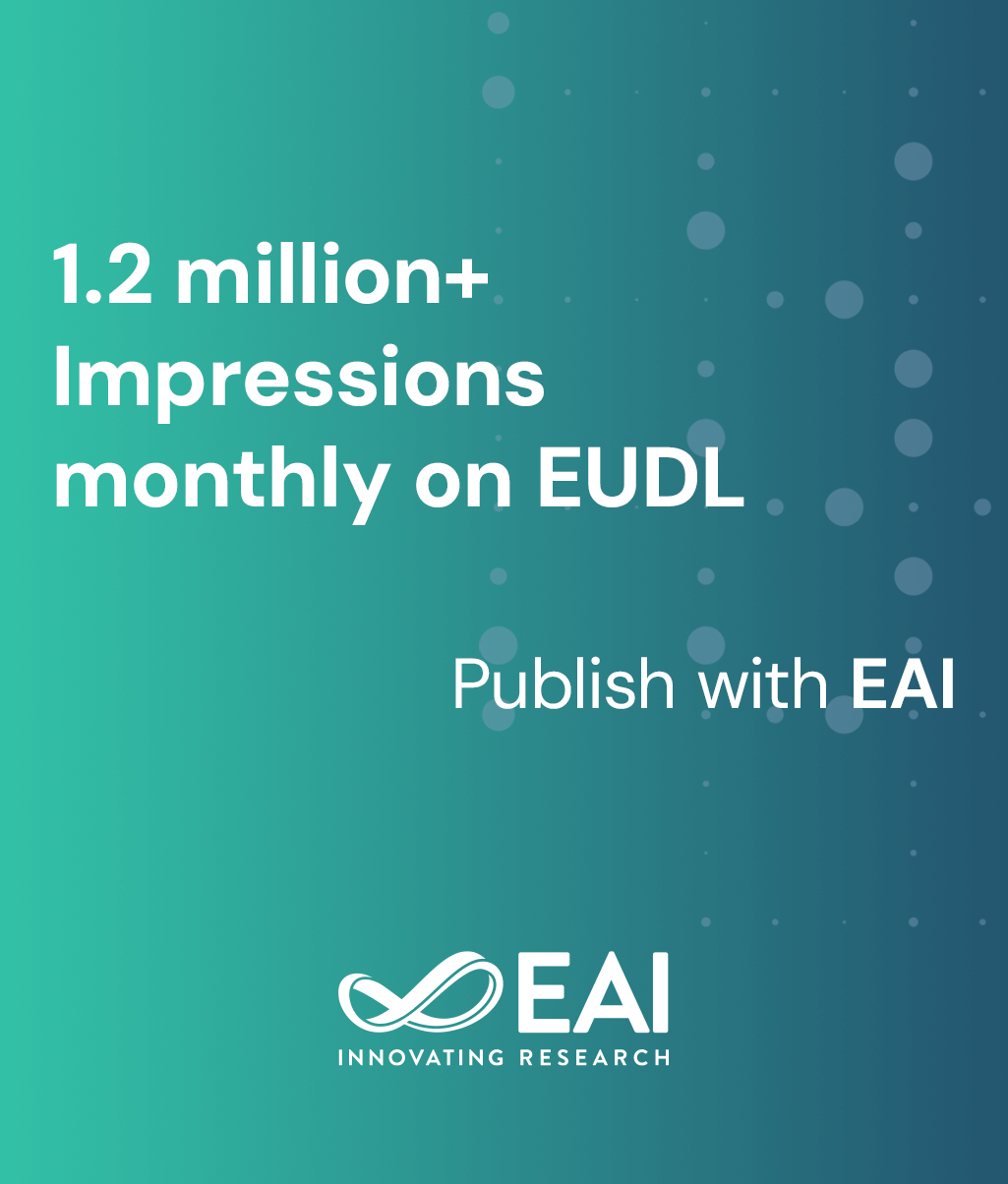
Research Article
Attention-Based Hybrid Model for Automatic Short Answer Scoring
@INPROCEEDINGS{10.1007/978-3-030-32216-8_37, author={Hui Qi and Yue Wang and Jinyu Dai and Jinqing Li and Xiaoqiang Di}, title={Attention-Based Hybrid Model for Automatic Short Answer Scoring}, proceedings={Simulation Tools and Techniques. 11th International Conference, SIMUtools 2019, Chengdu, China, July 8--10, 2019, Proceedings}, proceedings_a={SIMUTOOLS}, year={2019}, month={10}, keywords={Attention-based hybrid model Automatic short answer scoring BLSTM CNN}, doi={10.1007/978-3-030-32216-8_37} }
- Hui Qi
Yue Wang
Jinyu Dai
Jinqing Li
Xiaoqiang Di
Year: 2019
Attention-Based Hybrid Model for Automatic Short Answer Scoring
SIMUTOOLS
Springer
DOI: 10.1007/978-3-030-32216-8_37
Abstract
Neural network models have played an important role in text applications, such as document summaries and automatic short answer questions. In previous existing works, questions and answers are together used as input in recurrent neural networks (RNN) and convolutional neural networks (CNN), then output corresponding scores. This paper presents a method for measuring the score for short answer questions and answers. This paper makes scoring by establishing a hierarchical word-sentence model to represent questions and answers and using the attention mechanism to automatically determine the relative weight of questions and answers. Firstly, the model combines CNN and Bidirectional Long Short-Term Memory Networks (BLSTM) to extract the semantic features of questions and answers. Secondly, it captures the representation vector of relevant questions and answers from the sentence-level features. Finally, all feature vectors are concatenated and input to the output layer to obtain the corresponding score. Experiment results show that the model in this paper is better than multiple baselines.