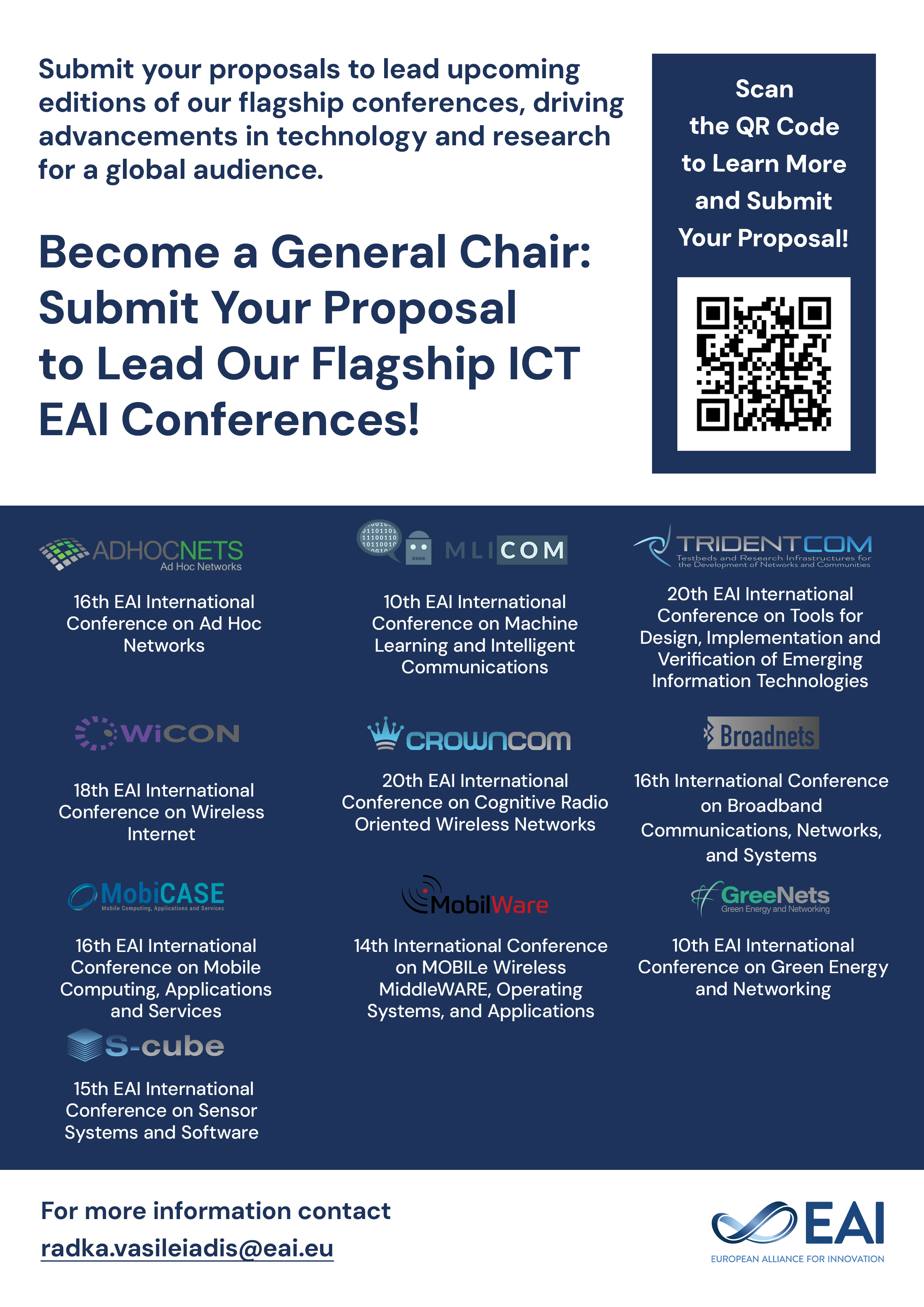
Research Article
A New MCMC Particle Filter Resampling Algorithm Based on Minimizing Sampling Variance
@INPROCEEDINGS{10.1007/978-3-030-32216-8_23, author={Juan Tian and Dan Li}, title={A New MCMC Particle Filter Resampling Algorithm Based on Minimizing Sampling Variance}, proceedings={Simulation Tools and Techniques. 11th International Conference, SIMUtools 2019, Chengdu, China, July 8--10, 2019, Proceedings}, proceedings_a={SIMUTOOLS}, year={2019}, month={10}, keywords={PF-resampling MCMC PSO Minimizing sampling variance}, doi={10.1007/978-3-030-32216-8_23} }
- Juan Tian
Dan Li
Year: 2019
A New MCMC Particle Filter Resampling Algorithm Based on Minimizing Sampling Variance
SIMUTOOLS
Springer
DOI: 10.1007/978-3-030-32216-8_23
Abstract
In order to solve the problem of particle divergence caused by deviation of sample distribution before and after resampling, a new Markov Chain Monte Carlo (MCMC) resampling algorithm based on minimizing sampling variance is proposed. First, MCMC transfer in which Particle Swarm Optimization (PSO) is possessed as the transfer kernel to construct Markov Chain is applied to the impoverished sample to combat sample degeneracy as well as sample impoverishment. Second, the algorithm takes the weighted variance as the cost function to measure the difference between the weighted particle discrete distribution before and after the resampling process, and optimizes the previous MCMC resampling by the minimum sampling variance criterion. Finally Experiment result shows that the algorithm can overcome particle impoverishment and realize the identical distribution of particles before and after resampling.