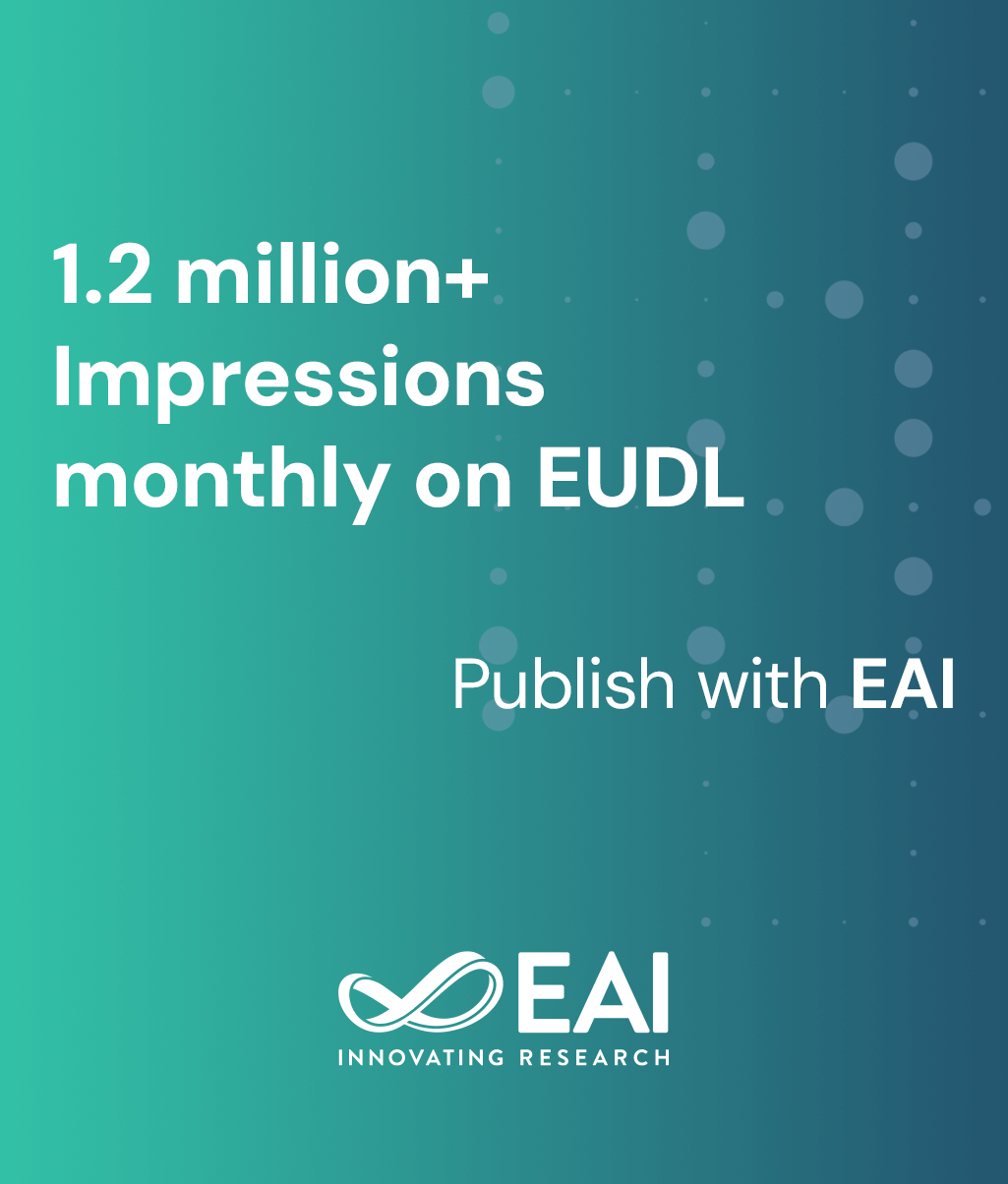
Research Article
A Linear Regression-Based Prediction Method to Traffic Flow for Low-Power WAN with Smart Electric Power Allocations
@INPROCEEDINGS{10.1007/978-3-030-32216-8_12, author={Bing Liu and Fanbo Meng and Yun Zhao and Xinge Qi and Bin Lu and Kai Yang and Xiao Yan}, title={A Linear Regression-Based Prediction Method to Traffic Flow for Low-Power WAN with Smart Electric Power Allocations}, proceedings={Simulation Tools and Techniques. 11th International Conference, SIMUtools 2019, Chengdu, China, July 8--10, 2019, Proceedings}, proceedings_a={SIMUTOOLS}, year={2019}, month={10}, keywords={Network traffic Low-power WAN Linear regression Traffic modeling Traffic prediction}, doi={10.1007/978-3-030-32216-8_12} }
- Bing Liu
Fanbo Meng
Yun Zhao
Xinge Qi
Bin Lu
Kai Yang
Xiao Yan
Year: 2019
A Linear Regression-Based Prediction Method to Traffic Flow for Low-Power WAN with Smart Electric Power Allocations
SIMUTOOLS
Springer
DOI: 10.1007/978-3-030-32216-8_12
Abstract
Currently power telecommunication access networks have many new requirements to meet the low-power WAN with smart electric power allocations. In such a case, network traffic in the low-power WAN has exhibited new features and there are some challenges for network managements. This paper uses the linear regression model to propose a new method to model and predict network traffic. Firstly, network traffic is modeled as a linear regression model according to the regression model theory. Then the linear regression modeling method is used to capture network traffic features. By calculating the parameters of the model, it can be decided correctly. Then, we can predict network traffic accurately. Simulation results show that our approach is effective and promising.