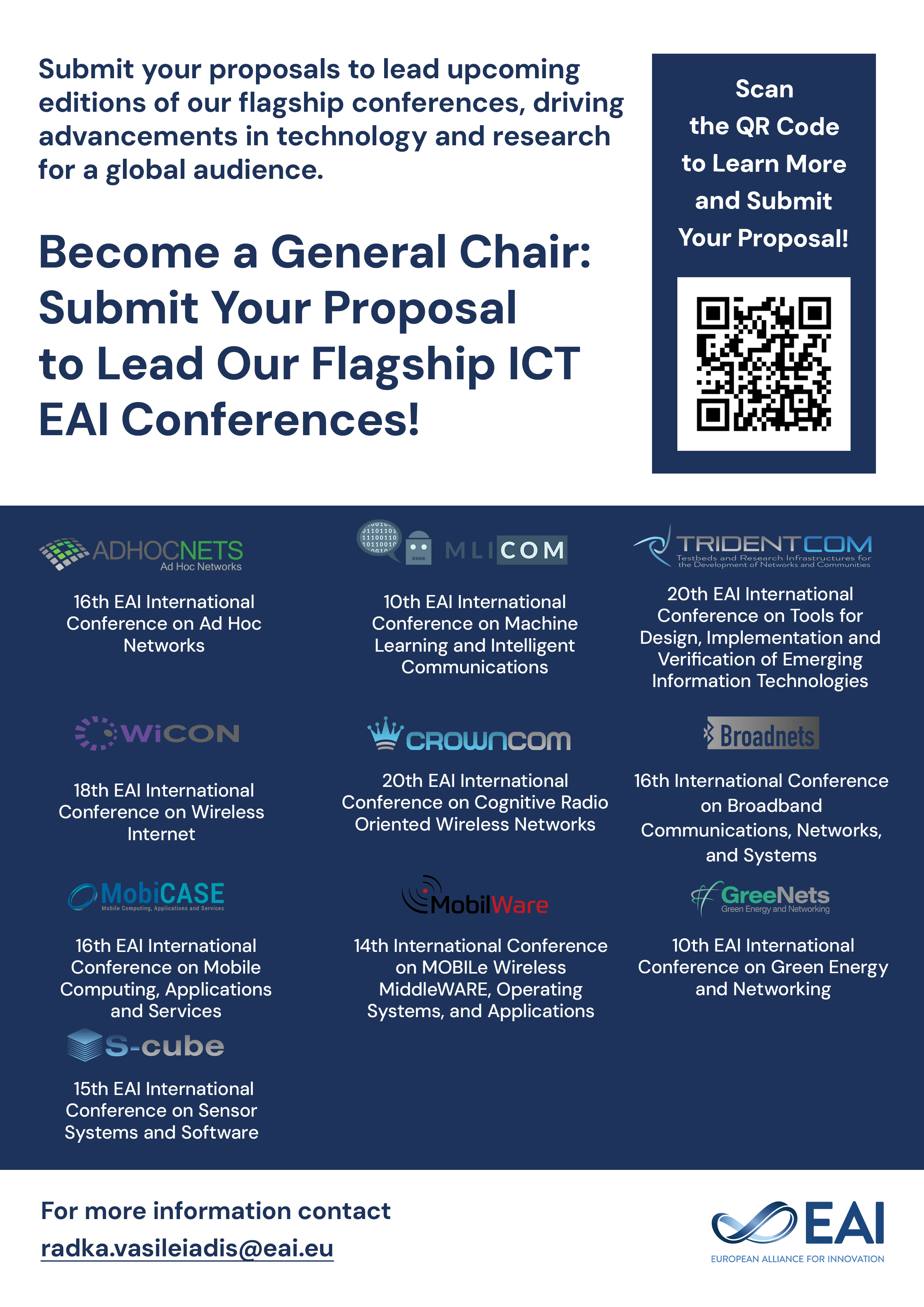
Research Article
A Data-Driven Approach for Network Intrusion Detection and Monitoring Based on Kernel Null Space
155 downloads
@INPROCEEDINGS{10.1007/978-3-030-30149-1_11, author={Thu Truong and Phuong Ta and Quoc Nguyen and Huu Nguyen and Kim Tran}, title={A Data-Driven Approach for Network Intrusion Detection and Monitoring Based on Kernel Null Space}, proceedings={Industrial Networks and Intelligent Systems. 5th EAI International Conference, INISCOM 2019, Ho Chi Minh City, Vietnam, August 19, 2019, Proceedings}, proceedings_a={INISCOM}, year={2019}, month={9}, keywords={Network security Kernel Quantile Estimator One-class classification Kernel Null Space Support vector machine}, doi={10.1007/978-3-030-30149-1_11} }
- Thu Truong
Phuong Ta
Quoc Nguyen
Huu Nguyen
Kim Tran
Year: 2019
A Data-Driven Approach for Network Intrusion Detection and Monitoring Based on Kernel Null Space
INISCOM
Springer
DOI: 10.1007/978-3-030-30149-1_11
Abstract
In this study, we propose a new approach to determine intrusions of network in real-time based on statistical process control technique and kernel null space method. The training samples in a class are mapped to a single point using the Kernel Null Foley-Sammon Transform. The Novelty Score are computed from testing samples in order to determine the threshold for the real-time detection of anomaly. The efficiency of the proposed method is illustrated over the KDD99 data set. The experimental results show that our new method outperforms the OCSVM and the original Kernel Null Space method by 1.53% and 3.86% respectively in terms of accuracy.
Copyright © 2019–2025 ICST