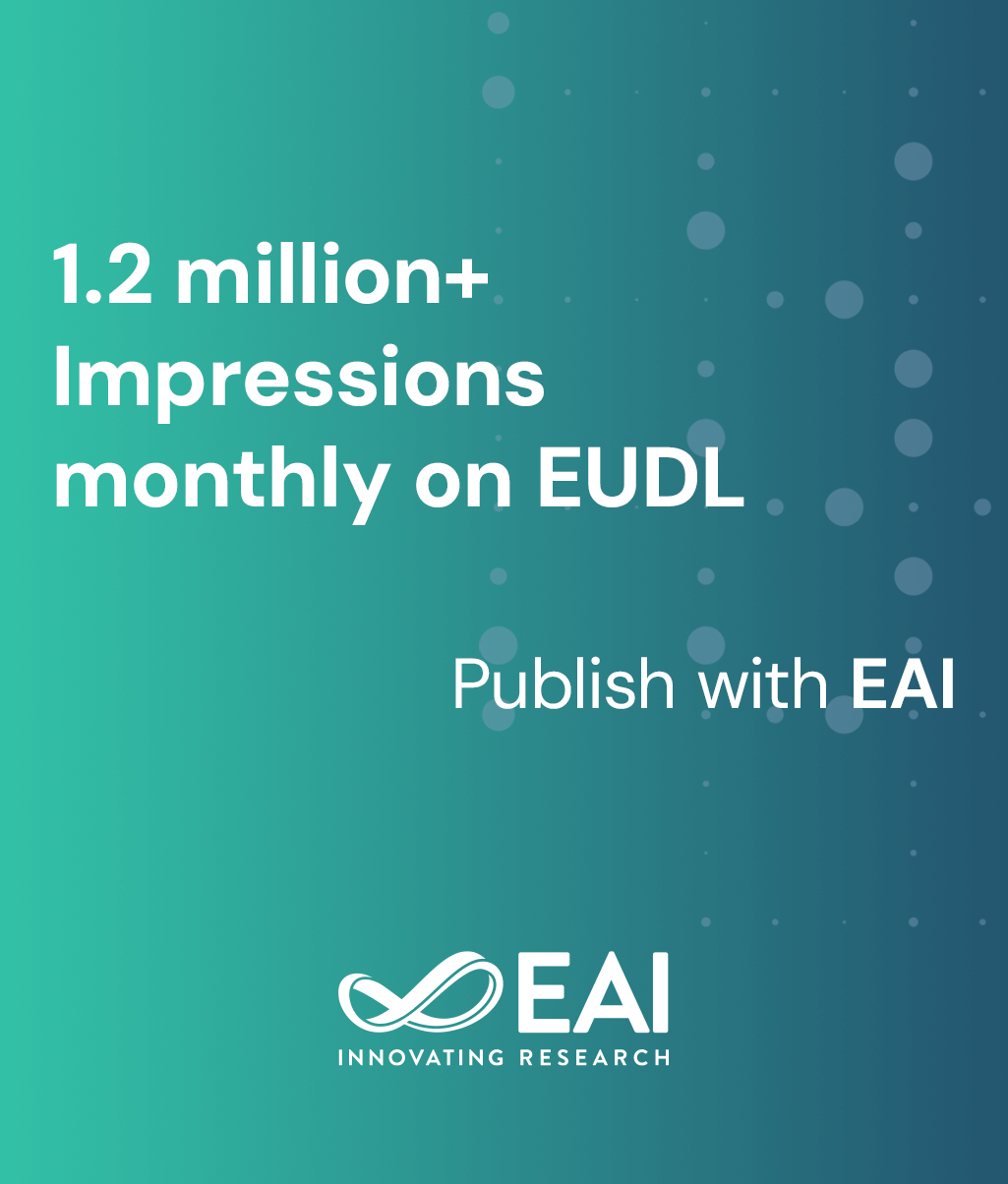
Research Article
Extracting Topics from Semi-structured Data for Enhancing Enterprise Knowledge Graphs
@INPROCEEDINGS{10.1007/978-3-030-30146-0_8, author={Neda Abolhassani and Lakshmish Ramaswamy}, title={Extracting Topics from Semi-structured Data for Enhancing Enterprise Knowledge Graphs}, proceedings={Collaborative Computing: Networking, Applications and Worksharing. 15th EAI International Conference, CollaborateCom 2019, London, UK, August 19-22, 2019, Proceedings}, proceedings_a={COLLABORATECOM}, year={2019}, month={8}, keywords={Topic modeling Knowledge graphs Semi-structured data MongoDB Gibbs Sampling Variational Bayes}, doi={10.1007/978-3-030-30146-0_8} }
- Neda Abolhassani
Lakshmish Ramaswamy
Year: 2019
Extracting Topics from Semi-structured Data for Enhancing Enterprise Knowledge Graphs
COLLABORATECOM
Springer
DOI: 10.1007/978-3-030-30146-0_8
Abstract
Unifying information across the organizational data silos that lack documentation, structure and automated semantic discovery has been of an intense interest in the recent years. Enterprise knowledge graph is a common tool of data integration and knowledge discovery and it has become a backbone to APIs that demand access to structured knowledge. A piece which was previously unnoticed in building enterprise knowledge graph, is adding an abstract layer of themes and concepts which is mapped to various documents stored as semi-structured files in databases. Augmenting enterprise knowledge graphs by concepts will help companies to find the trends in their data and get a holistic view over their entire data stores. Extracting topics from semi-structured data suffers from lack of corpus or description as its major challenge. In this research, we investigate the impact of self-supplementation of words and documents on probabilistic topic modeling upon semi-structured data. Another contribution of this paper is finding the best tuning of probabilistic topic modeling that fits semi-structured data. The extracted topics are potential summaries and concepts about the dataset. Moreover, they can be mapped to their sources of origin in order to extend the enterprise knowledge graph. We consider 2 inferencing techniques and demonstrate the results on real data pools from Open City data and Kaggle data containing 7.5 GB and 1.15 GB of data stored in MongoDB collections, respectively. We also propose a selection heuristic for effective identification of topics hidden in various data sources.