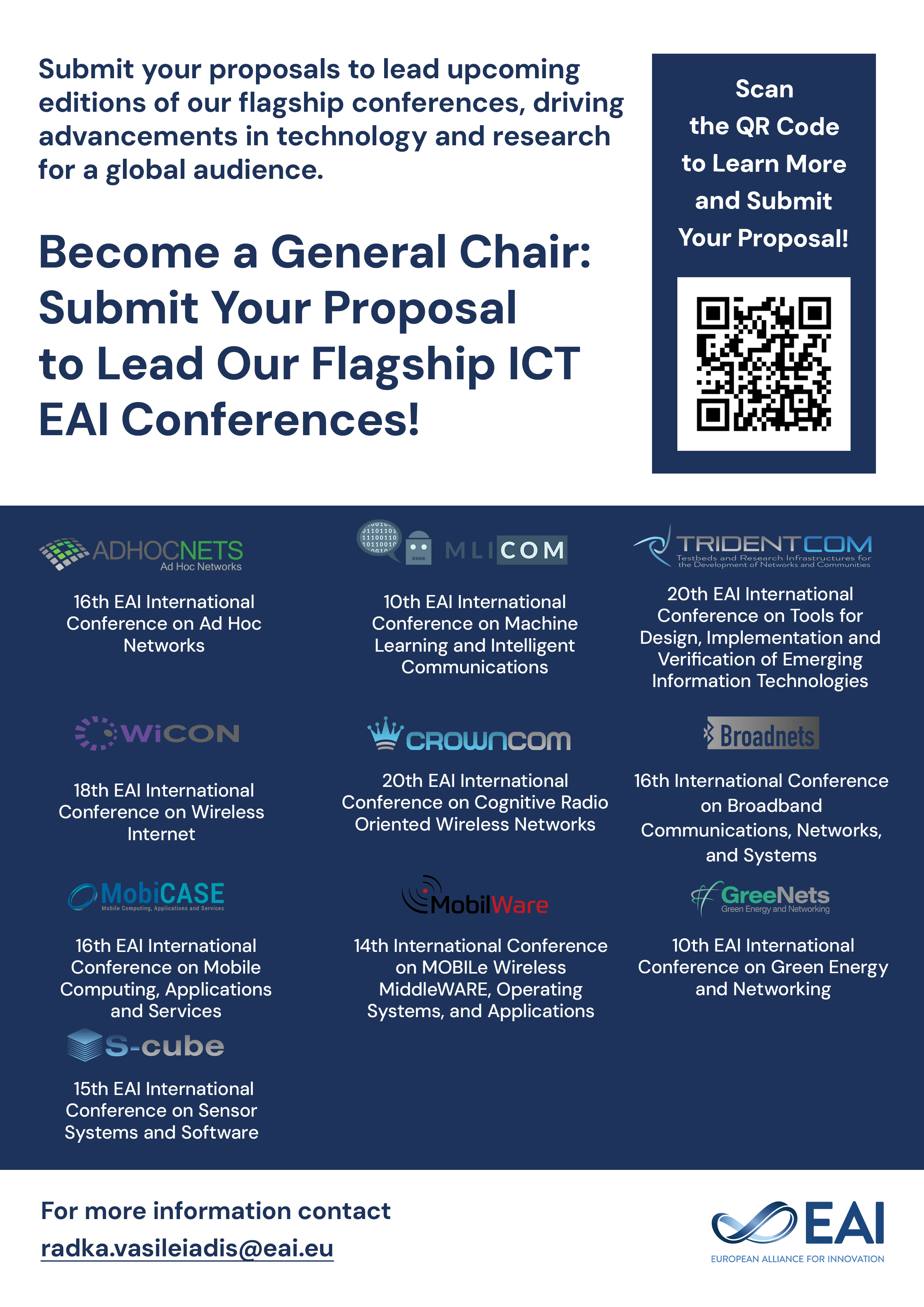
Research Article
Covering Diversification and Fairness for Better Recommendation (Short Paper)
@INPROCEEDINGS{10.1007/978-3-030-30146-0_54, author={Qing Yang and Li Han and Ya Zhou and Shaobing Liu and Jingwei Zhang and Zhongqin Bi and Fang Pan}, title={Covering Diversification and Fairness for Better Recommendation (Short Paper)}, proceedings={Collaborative Computing: Networking, Applications and Worksharing. 15th EAI International Conference, CollaborateCom 2019, London, UK, August 19-22, 2019, Proceedings}, proceedings_a={COLLABORATECOM}, year={2019}, month={8}, keywords={Diversified recommendation Recommendation fairness Recommendation evaluation}, doi={10.1007/978-3-030-30146-0_54} }
- Qing Yang
Li Han
Ya Zhou
Shaobing Liu
Jingwei Zhang
Zhongqin Bi
Fang Pan
Year: 2019
Covering Diversification and Fairness for Better Recommendation (Short Paper)
COLLABORATECOM
Springer
DOI: 10.1007/978-3-030-30146-0_54
Abstract
Smart applications are appealing an accurate matching between users and items, in which recommendation technologies are applied widely. Since recommendation serve for two roles, namely users and items, accuracy is not the only focus, the diversification and fairness should also be paid more attention for improving recommendation performance. The tradeoff among the accuracy, diversification and fairness on recommendation is bringing a big challenge. This paper proposed a novelty recommendation model to ensure the recommendation performance, which introduces a multi-variate linear regression model to cooperate with the collaborative filtering method. This study utilizes an improved similarity metrics to discover the closeness between users and item categories under the help of the collaborative filtering methods, and exploits the micro attribute information of items by a multi-variate linear regression model to decide the final recommended items. The experimental results show that our proposed method can provide better recommendation accuracy, diversification and fairness than the recommendation based on pure collaborative filtering method.