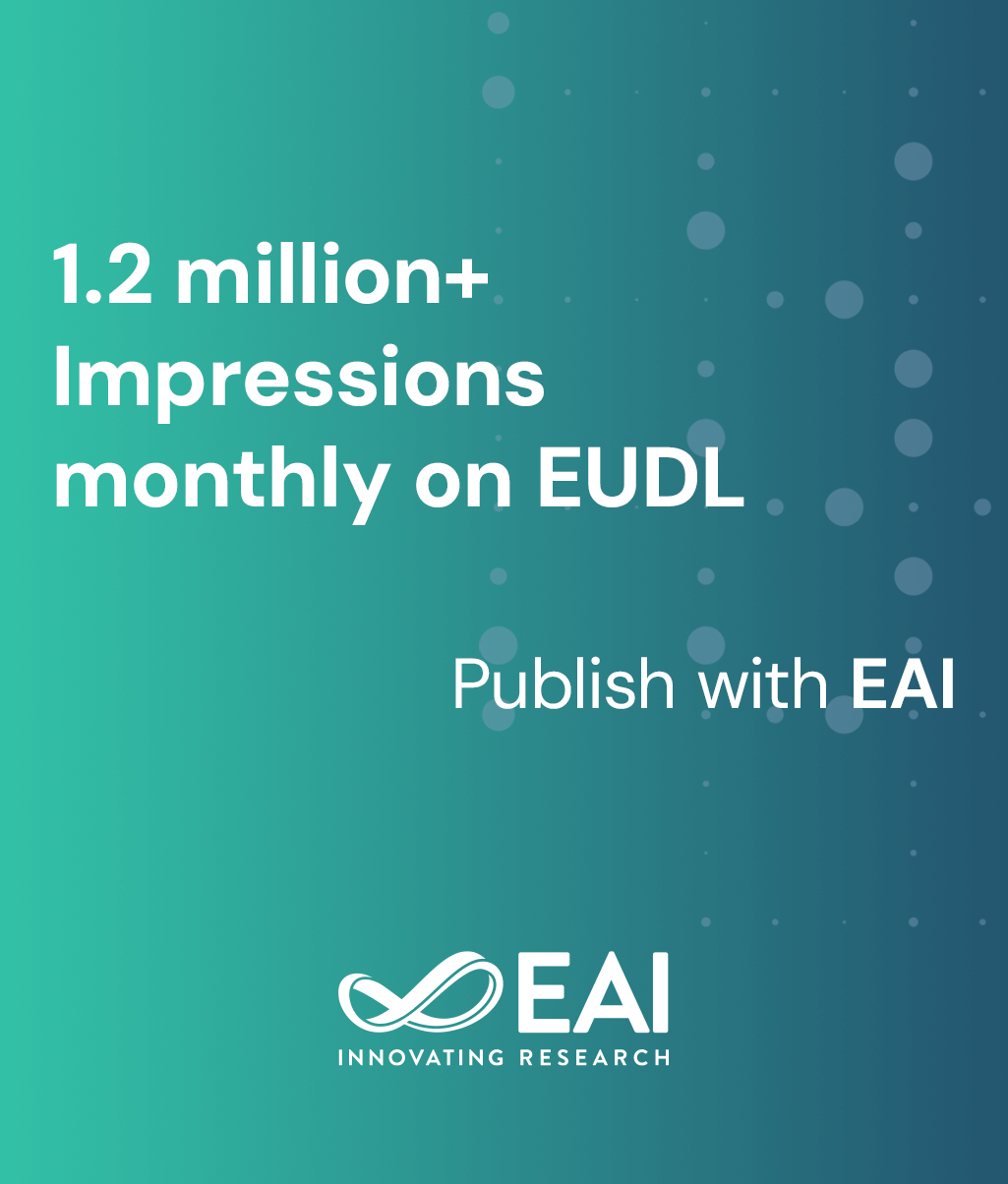
Research Article
Detecting Overlapping Communities of Nodes with Multiple Attributes from Heterogeneous Networks
@INPROCEEDINGS{10.1007/978-3-030-30146-0_51, author={Kamal Taha and Paul Yoo}, title={Detecting Overlapping Communities of Nodes with Multiple Attributes from Heterogeneous Networks}, proceedings={Collaborative Computing: Networking, Applications and Worksharing. 15th EAI International Conference, CollaborateCom 2019, London, UK, August 19-22, 2019, Proceedings}, proceedings_a={COLLABORATECOM}, year={2019}, month={8}, keywords={Social networks Heterogeneous information networks Community detection Overlapping communities Multi-domain community}, doi={10.1007/978-3-030-30146-0_51} }
- Kamal Taha
Paul Yoo
Year: 2019
Detecting Overlapping Communities of Nodes with Multiple Attributes from Heterogeneous Networks
COLLABORATECOM
Springer
DOI: 10.1007/978-3-030-30146-0_51
Abstract
Many methods have been proposed for detecting communities from heterogeneous information networks with general topologies. However, most of these methods can detect communities with homogeneous structures containing nodes with only a single attribute. Investigating methods for detecting communities containing nodes with multiple attributes from heterogeneous information networks with general topologies has been understudied. Such communities are realistic in real-world social structures and exhibits many interesting properties. Towards this, we propose a system called DOMAIN that can detect overlapping communities of nodes with multiple attributes from heterogeneous information networks with general topologies. The framework of DOMAIN focuses on domains (i.e., attributes) that describe human characteristics such as ethnicity, culture, religion, demographic, age, or the like. The ultimate objective of the framework is to detect the sub-communities with the possible number of domains, to which an active user belongs. The smaller a sub-community is, the more specific and granular its interests are. The interests of such a sub-community is the union of the interests and characteristics of the single domain communities, from which it is constructed. We evaluated DOMAIN by comparing it experimentally with three methods. Results revealed marked improvement.