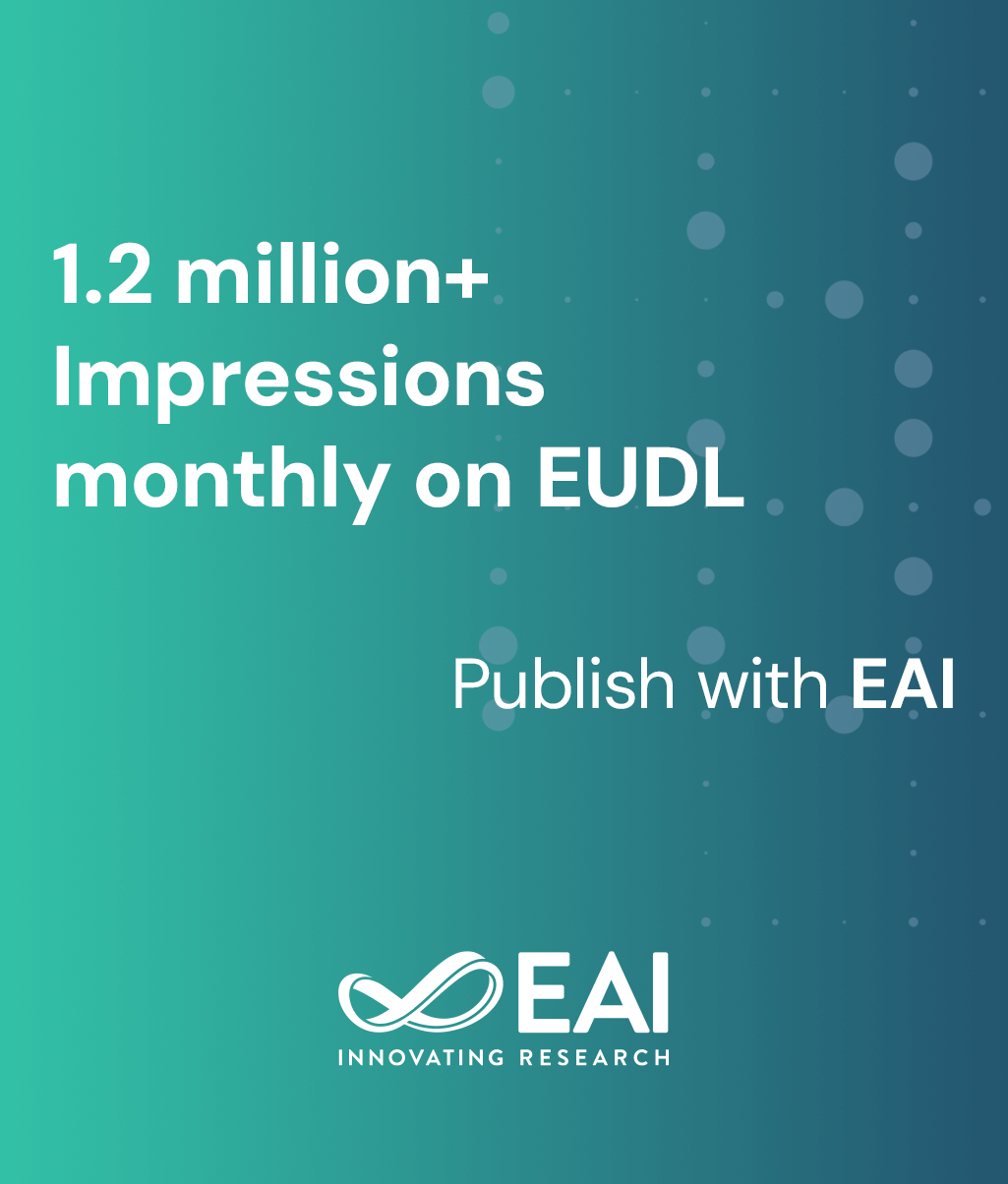
Research Article
Context-Aware Point-of-Interest Recommendation Algorithm with Interpretability
@INPROCEEDINGS{10.1007/978-3-030-30146-0_50, author={Guoming Zhang and Lianyong Qi and Xuyun Zhang and Xiaolong Xu and Wanchun Dou}, title={Context-Aware Point-of-Interest Recommendation Algorithm with Interpretability}, proceedings={Collaborative Computing: Networking, Applications and Worksharing. 15th EAI International Conference, CollaborateCom 2019, London, UK, August 19-22, 2019, Proceedings}, proceedings_a={COLLABORATECOM}, year={2019}, month={8}, keywords={Point-of-interest recommendation Interpretability Location based social network}, doi={10.1007/978-3-030-30146-0_50} }
- Guoming Zhang
Lianyong Qi
Xuyun Zhang
Xiaolong Xu
Wanchun Dou
Year: 2019
Context-Aware Point-of-Interest Recommendation Algorithm with Interpretability
COLLABORATECOM
Springer
DOI: 10.1007/978-3-030-30146-0_50
Abstract
With the rapid development of mobile Internet, smart devices, and positioning technologies, location-based social networks (LBSNs) are growing rapidly. In LBSNs, point-of-interest (POI) recommendation is a crucial personalized location service that has become a research hotspot. To address extreme sparsity of user check-in data, a growing line of research exploits spatial-temporal information, social relationship, content information, popularity, and other factors to improve recommendation performance. However, the temporal and spatial transfers of user preferences are seldom mentioned in existing works, and interpretability, which is an important factor to enhance credibility of recommendation result, is overlooked. To cope with these issues, we propose a context-aware POI recommendation framework, which integrates users’ long-term static and time-varying preferences to improve recommendation performance and provide explanations. Experimental results over two real-world LBSN datasets demonstrate that the proposed solution has better performance than other advanced POI recommendation approaches.