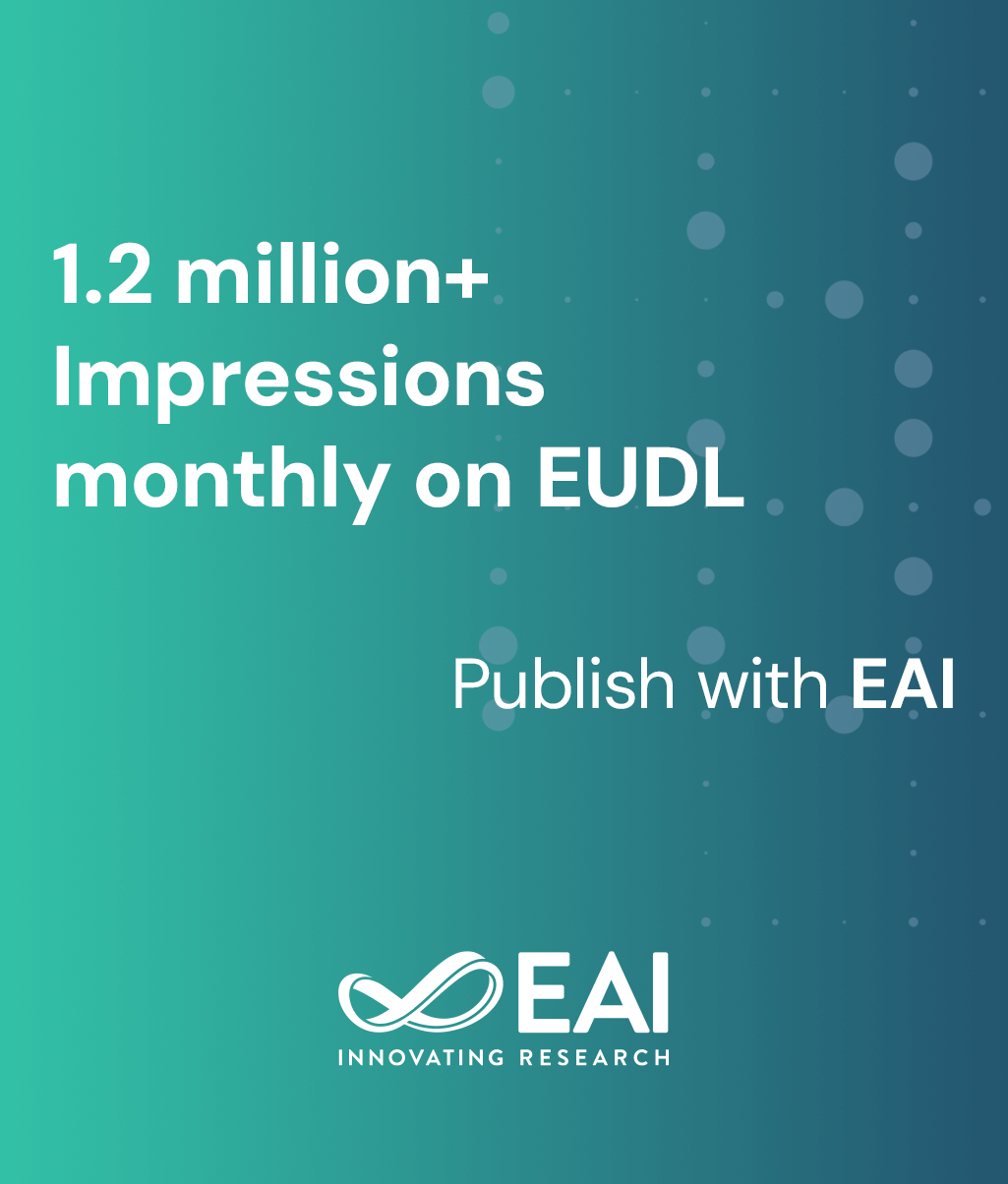
Research Article
A Dynamic Planning Framework for QoS-Based Mobile Service Composition Under Cloud-Edge Hybrid Environments
@INPROCEEDINGS{10.1007/978-3-030-30146-0_5, author={Honghao Gao and Wanqiu Huang and Qiming Zou and Xiaoxian Yang}, title={A Dynamic Planning Framework for QoS-Based Mobile Service Composition Under Cloud-Edge Hybrid Environments}, proceedings={Collaborative Computing: Networking, Applications and Worksharing. 15th EAI International Conference, CollaborateCom 2019, London, UK, August 19-22, 2019, Proceedings}, proceedings_a={COLLABORATECOM}, year={2019}, month={8}, keywords={Cloud-edge hybrid environments Mobile service composition Service QoS Service failure Dynamic reconfiguration}, doi={10.1007/978-3-030-30146-0_5} }
- Honghao Gao
Wanqiu Huang
Qiming Zou
Xiaoxian Yang
Year: 2019
A Dynamic Planning Framework for QoS-Based Mobile Service Composition Under Cloud-Edge Hybrid Environments
COLLABORATECOM
Springer
DOI: 10.1007/978-3-030-30146-0_5
Abstract
In cloud-edge hybrid environments, when QoS constraints of the SOA-based mobile service composition change, a dynamic reconfiguration needs to be performed. Different from the traditional cloud service, the cloud-edge hybrid environment has the characteristics of limited resource storage, limited energy at the edge and uncertain users who move frequently. Dynamic reconfiguration in this mode is challenging. QoS is an important indicator of service evaluation. Most studies focus on only the static QoS attributes of the service. However, the QoS of a service is not statically constant; it changes dynamically over time. Therefore, to avoid the immediate failure of the service and ensure the stability of the mobile service composition after dynamic reconfiguration, an LSTM neural network is applied to predict the future QoS value for candidate service. This value is used as a service evaluation indicator during dynamic reconfiguration. Then, attributes such as energy consumption, traffic and moving track are considered. A cost-reward mechanism is constructed to calculate the cost and reward of the service when it is invoked. The reasonable restriction conditions are added for controlling dynamic reconfiguration. Finally, the dynamic reconfiguration problem-solving process and framework for mobile service composition based on QoS in a cloud-edge hybrid environment is introduced, guiding the mobile service composition dynamic reconfiguration task in cloud-edge hybrid environments.