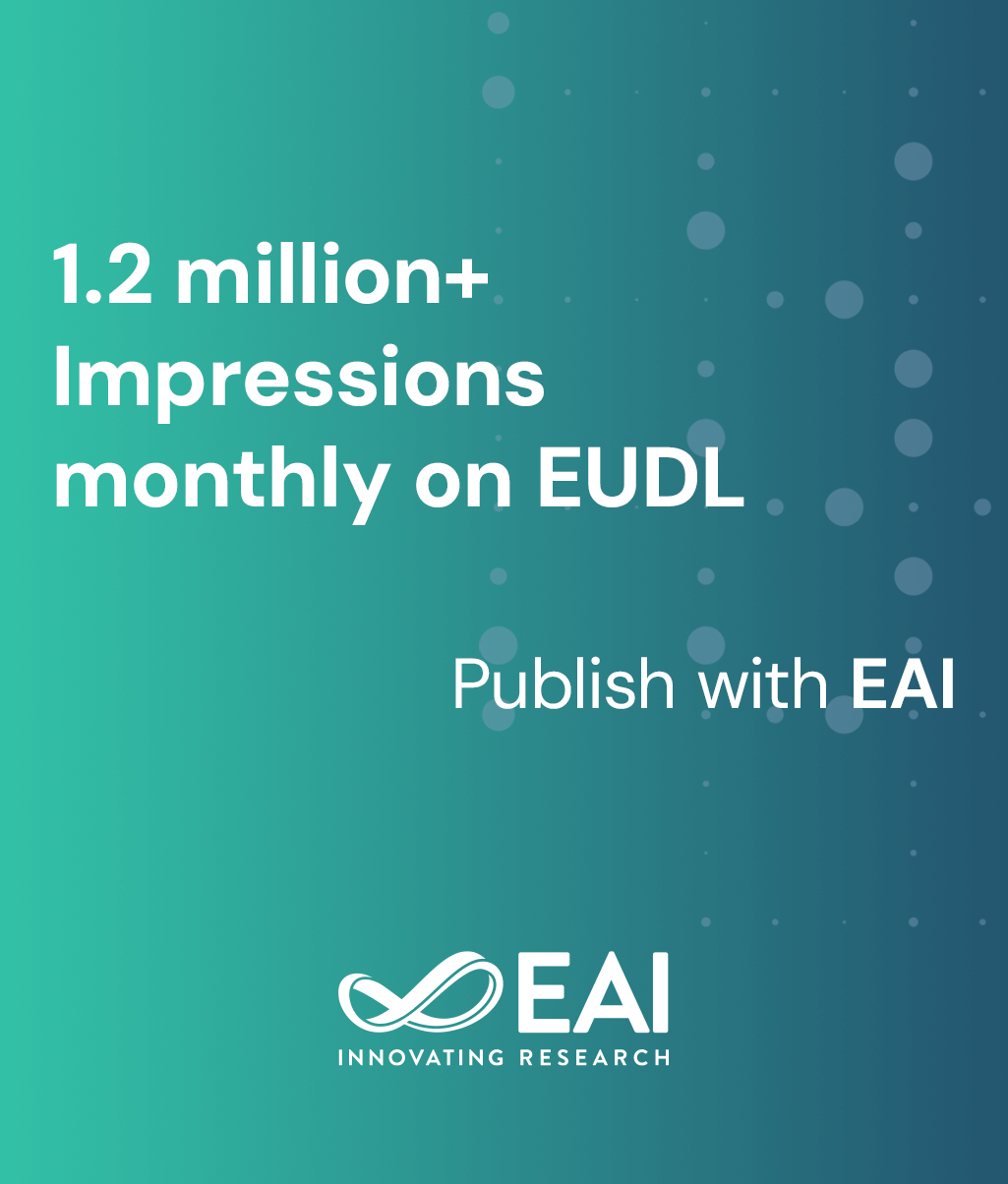
Research Article
Towards Efficient Pairwise Ranking for Service Using Multidimensional Classification
@INPROCEEDINGS{10.1007/978-3-030-30146-0_44, author={Yingying Yuan and Jiwei Huang and Yeping Zhu and Yufei Hu}, title={Towards Efficient Pairwise Ranking for Service Using Multidimensional Classification}, proceedings={Collaborative Computing: Networking, Applications and Worksharing. 15th EAI International Conference, CollaborateCom 2019, London, UK, August 19-22, 2019, Proceedings}, proceedings_a={COLLABORATECOM}, year={2019}, month={8}, keywords={Service ranking Multidimensional classification Pairwise ranking Markov model}, doi={10.1007/978-3-030-30146-0_44} }
- Yingying Yuan
Jiwei Huang
Yeping Zhu
Yufei Hu
Year: 2019
Towards Efficient Pairwise Ranking for Service Using Multidimensional Classification
COLLABORATECOM
Springer
DOI: 10.1007/978-3-030-30146-0_44
Abstract
With the growing popularity of services which meet the divergent requirements from users, service selection and recommendation have drawn significant attention in services computing community. Service ranking is the most important part in service selection and recommendation. Although there have been several existing approaches of service ranking which is basically rating-based, suffering from the heterogeneity of ranking criteria from users. Moreover, the efficiency of such comparison-based approaches is the bottleneck in reality. To attack these challenges, an efficient pairwise ranking scheme with multidimensional classification is proposed in this paper, which also fully considers the context information of service and users. Furthermore, the scheme is able to mitigate data sparsity of users similarity matrix and improve accuracy. Next, we introduce a random walk model for ranking formulation, and propose a Markov chain based approach to obtain the global ranking. Finally, the efficacy of our approach is validated by experiments adopting the real-world YELP dataset.