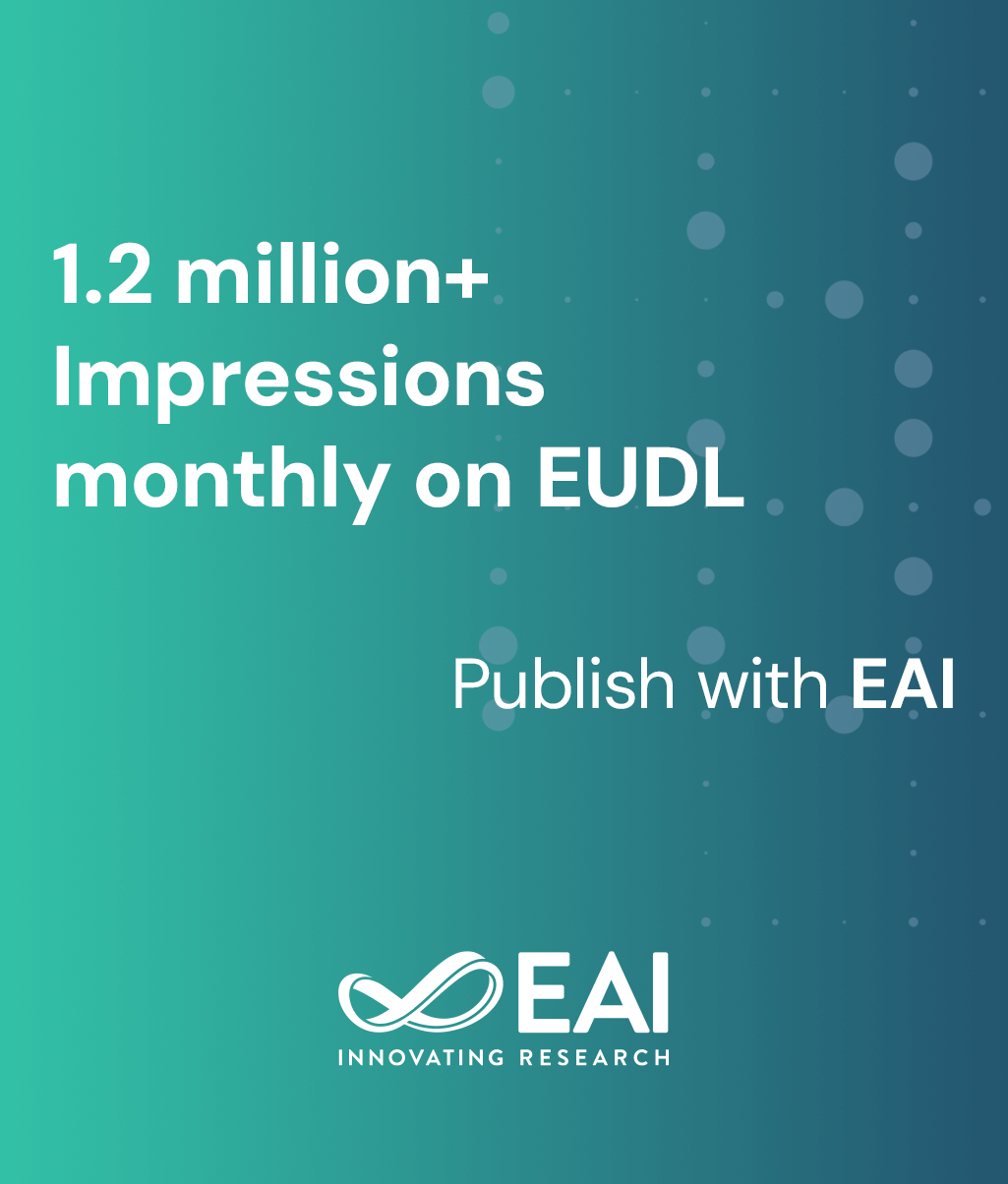
Research Article
A Smart Topology Construction Method for Anti-tracking Network Based on the Neural Network
@INPROCEEDINGS{10.1007/978-3-030-30146-0_31, author={Changbo Tian and YongZheng Zhang and Tao Yin and Yupeng Tuo and Ruihai Ge}, title={A Smart Topology Construction Method for Anti-tracking Network Based on the Neural Network}, proceedings={Collaborative Computing: Networking, Applications and Worksharing. 15th EAI International Conference, CollaborateCom 2019, London, UK, August 19-22, 2019, Proceedings}, proceedings_a={COLLABORATECOM}, year={2019}, month={8}, keywords={Anti-tracking network Topology Neural network Cyber security}, doi={10.1007/978-3-030-30146-0_31} }
- Changbo Tian
YongZheng Zhang
Tao Yin
Yupeng Tuo
Ruihai Ge
Year: 2019
A Smart Topology Construction Method for Anti-tracking Network Based on the Neural Network
COLLABORATECOM
Springer
DOI: 10.1007/978-3-030-30146-0_31
Abstract
Anti-tracking network is the effective method to protect the network users’ privacy confronted with the increasingly rampant network monitoring and network tracing. But the architecture of the current anti-tracking network is easy to be attacked, traced and undermined. In this paper, We propose smart topology construction method (STon) to provide the self-management and self-optimization of topology for anti-tracking network. We firstly deploy the neural network on each node of the anti-tracking network. Each node can collect its local network state and calculate the network state parameters by the neural network to decide the link state with other nodes. At last, each node optimizes its local topology according to the link state. With the collaboration of all nodes in the network, the network can achieve the self-management and self-optimization of its own topology. The experimental results showes that STon has a better robustness, communication efficiency and anti-tracking performance than the current popular P2P structures.