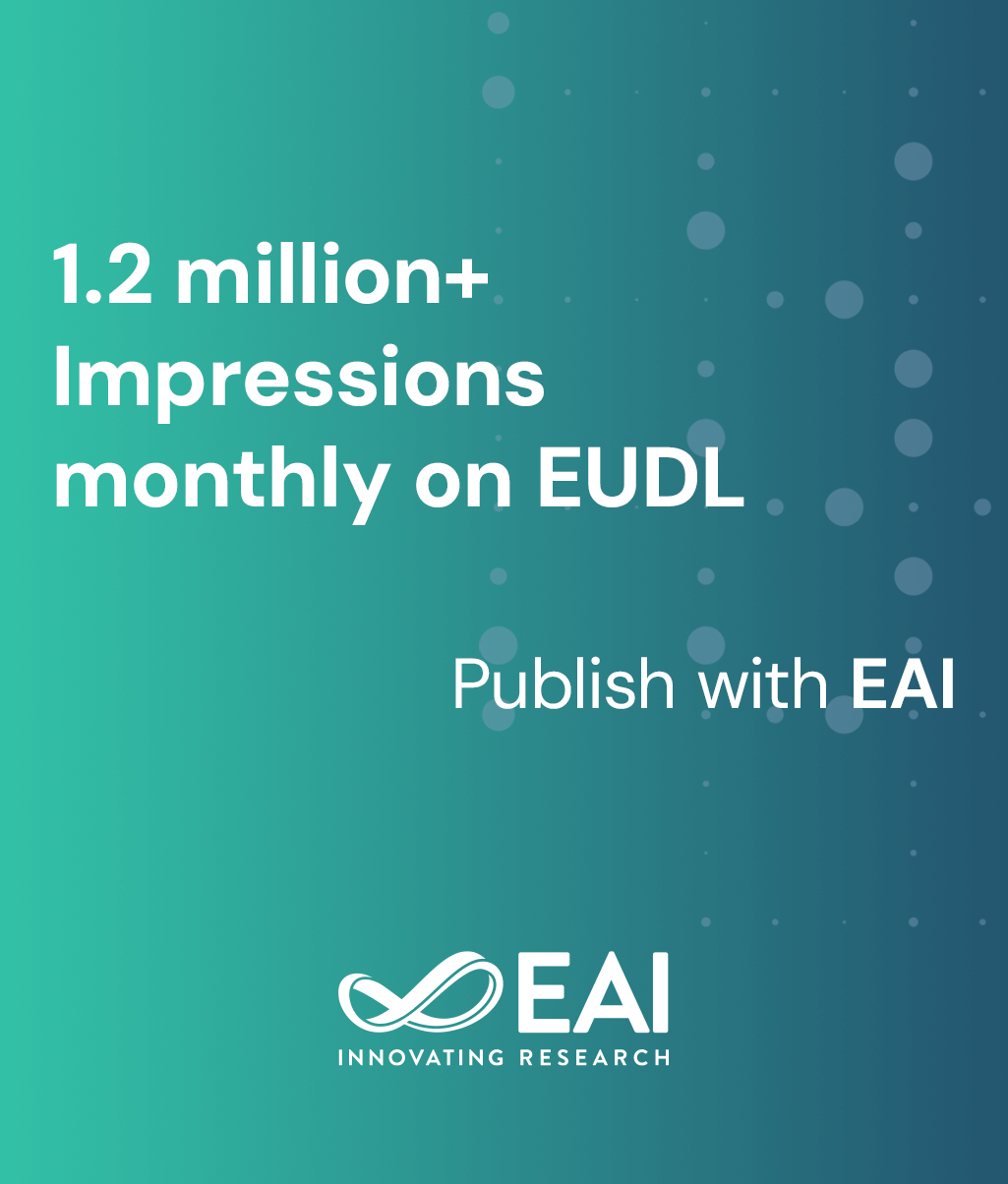
Research Article
Relation Extraction Toward Patent Domain Based on Keyword Strategy and Attention+BiLSTM Model (Short Paper)
@INPROCEEDINGS{10.1007/978-3-030-30146-0_28, author={Xueqiang Lv and Xiangru Lv and Xindong You and Zhian Dong and Junmei Han}, title={Relation Extraction Toward Patent Domain Based on Keyword Strategy and Attention+BiLSTM Model (Short Paper)}, proceedings={Collaborative Computing: Networking, Applications and Worksharing. 15th EAI International Conference, CollaborateCom 2019, London, UK, August 19-22, 2019, Proceedings}, proceedings_a={COLLABORATECOM}, year={2019}, month={8}, keywords={Patent terminology relation extraction Patent knowledge graph Keyword features BiLSTM Attention mechanism}, doi={10.1007/978-3-030-30146-0_28} }
- Xueqiang Lv
Xiangru Lv
Xindong You
Zhian Dong
Junmei Han
Year: 2019
Relation Extraction Toward Patent Domain Based on Keyword Strategy and Attention+BiLSTM Model (Short Paper)
COLLABORATECOM
Springer
DOI: 10.1007/978-3-030-30146-0_28
Abstract
Patent terminology relation extraction is of great significance to the construction of patent Knowledge graph. In order to solve the problem of long-distance dependency in traditional depth learning, a new method of patent terminology relation extraction is proposed, which combines attention mechanism and bi-directional LSTM model and with keyword strategy. Category keyword features in each sentence obtained by the improved TextRank with the patent text information vectorization added. BiLSTM neural work and attention mechanism are employed to extract the temporal information and sentence-level global feature information. Moreover, pooling layer is added to obtain the local features of the text. Finally, we fuse the global features and local features, and output the final classification results through the softmax classifier. The addition of category keywords improves the distinction of categories. Substantial experimental results demonstrate that the proposed model outperform the state-of-art neural model in patent terminology relation extraction.