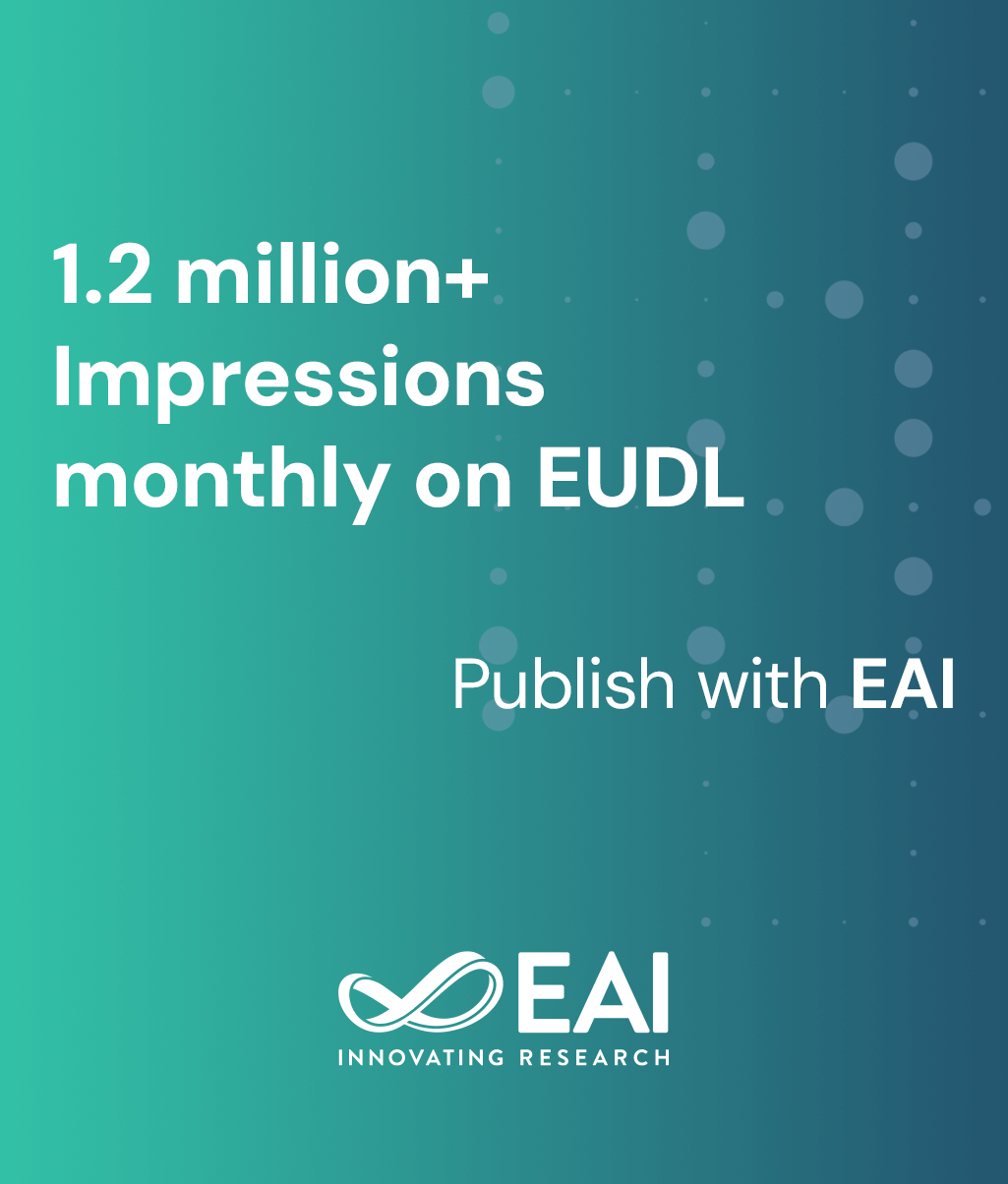
Research Article
Web Services Classification with Topical Attention Based Bi-LSTM
@INPROCEEDINGS{10.1007/978-3-030-30146-0_27, author={Yingcheng Cao and Jianxun Liu and Buqing Cao and Min Shi and Yiping Wen and Zhenlian Peng}, title={Web Services Classification with Topical Attention Based Bi-LSTM}, proceedings={Collaborative Computing: Networking, Applications and Worksharing. 15th EAI International Conference, CollaborateCom 2019, London, UK, August 19-22, 2019, Proceedings}, proceedings_a={COLLABORATECOM}, year={2019}, month={8}, keywords={Web services Bi-directional Long Short-Term Memory LDA topic model Web service classification Attention model}, doi={10.1007/978-3-030-30146-0_27} }
- Yingcheng Cao
Jianxun Liu
Buqing Cao
Min Shi
Yiping Wen
Zhenlian Peng
Year: 2019
Web Services Classification with Topical Attention Based Bi-LSTM
COLLABORATECOM
Springer
DOI: 10.1007/978-3-030-30146-0_27
Abstract
With the rapid growth of the number of Web services on the Internet, how to classify web services correctly and efficiently become more and more important in the development and application of Web services. Existing function-based service clustering techniques have some problems, such as the sparse document semantics, unconsidered word order and the context information, so the accuracy of service classification needs to be further improved. To address this problem, this paper exploits the attention mechanism to combine the local implicit state vector of Bi-LSTM and the global LDA topic vector, and proposes a method of Web services classification with topical attention based Bi-LSTM. Specifically, it uses Bi-LSTM to automatically learn the feature representation of Web service. Then, it utilizes the offline training to obtain the topic vector of Web service document and performs the topic attention strengthening processing for Web service feature representation, and obtains the importance or weight of the different words in Web service document. Finally, the enhanced Web service feature representation is used as the input of the softmax neural network layer to perform the classification prediction of Web service. The experimental results validate the efficiency and effectiveness of the proposed method.