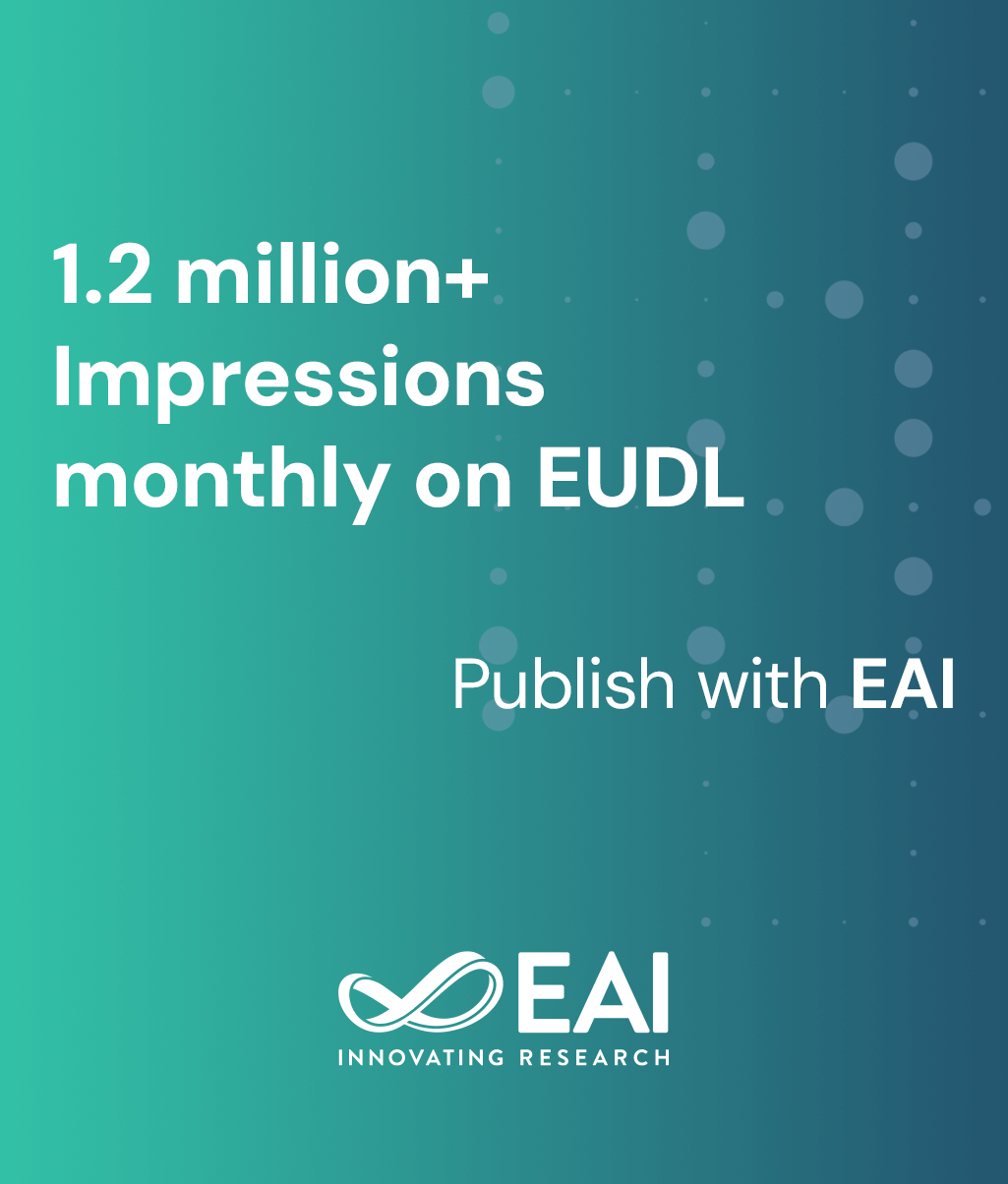
Research Article
Positive-Unlabeled Learning for Sentiment Analysis with Adversarial Training
@INPROCEEDINGS{10.1007/978-3-030-30146-0_25, author={Yueshen Xu and Lei Li and Jianbin Huang and Yuyu Yin and Wei Shao and Zhida Mai and Lei Hei}, title={Positive-Unlabeled Learning for Sentiment Analysis with Adversarial Training}, proceedings={Collaborative Computing: Networking, Applications and Worksharing. 15th EAI International Conference, CollaborateCom 2019, London, UK, August 19-22, 2019, Proceedings}, proceedings_a={COLLABORATECOM}, year={2019}, month={8}, keywords={Sentiment analysis PU learning problem Adversarial training LSTM Attention mechanism}, doi={10.1007/978-3-030-30146-0_25} }
- Yueshen Xu
Lei Li
Jianbin Huang
Yuyu Yin
Wei Shao
Zhida Mai
Lei Hei
Year: 2019
Positive-Unlabeled Learning for Sentiment Analysis with Adversarial Training
COLLABORATECOM
Springer
DOI: 10.1007/978-3-030-30146-0_25
Abstract
Sentiment classification is a critical task in sentiment analysis and other text mining applications. As a sub-problem of sentiment classification, positive and unlabeled learning or positive-unlabeled learning (PU learning) problem widely exists in real-world cases, but it has not been given enough attention. In this paper, we aim to solve PU learning problem under the framework of adversarial training and neural network. We propose a novel model for PU learning problem, which is based on adversarial training and attention-based long short-term memory (LSTM) network. In our model, we design a new adversarial training technique. We conducted extensive experiments on two real-world datasets. The experimental results demonstrate that our proposed model outperforms the compared methods, including the well-known traditional methods and state-of-the-art methods. We also report the training time, and discuss the sensitivity of our model to parameters.