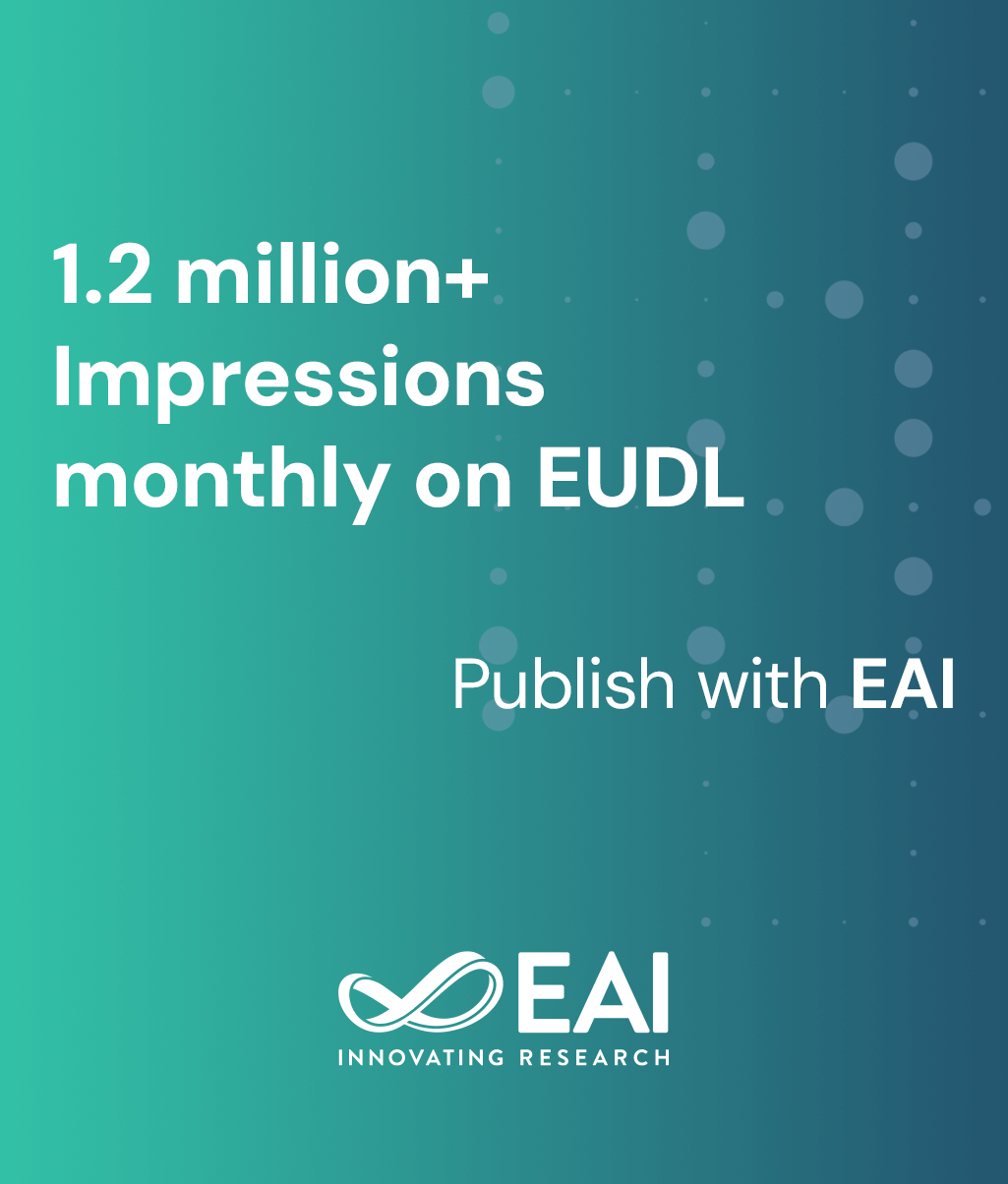
Research Article
Integration of Machine Learning Techniques as Auxiliary Diagnosis of Inherited Metabolic Disorders: Promising Experience with Newborn Screening Data
@INPROCEEDINGS{10.1007/978-3-030-30146-0_23, author={Bo Lin and Jianwei Yin and Qiang Shu and Shuiguang Deng and Ying Li and Pingping Jiang and Rulai Yang and Calton Pu}, title={Integration of Machine Learning Techniques as Auxiliary Diagnosis of Inherited Metabolic Disorders: Promising Experience with Newborn Screening Data}, proceedings={Collaborative Computing: Networking, Applications and Worksharing. 15th EAI International Conference, CollaborateCom 2019, London, UK, August 19-22, 2019, Proceedings}, proceedings_a={COLLABORATECOM}, year={2019}, month={8}, keywords={Newborn screening Machine learning Metabolic patterns Auxiliary diagnosis}, doi={10.1007/978-3-030-30146-0_23} }
- Bo Lin
Jianwei Yin
Qiang Shu
Shuiguang Deng
Ying Li
Pingping Jiang
Rulai Yang
Calton Pu
Year: 2019
Integration of Machine Learning Techniques as Auxiliary Diagnosis of Inherited Metabolic Disorders: Promising Experience with Newborn Screening Data
COLLABORATECOM
Springer
DOI: 10.1007/978-3-030-30146-0_23
Abstract
Tandem mass spectrometry is an advanced biochemical analysis method and has been widely used in screening of inherited metabolic disorders (IMDs). Obtained examination results are filtered by cutoff values and then interpreted based on doctor’s knowledge to get diagnoses. However, cutoff-based approaches have difficulties with the correlations of multiple metabolites. Doctor’s experiences affect the diagnostic decision-making as well. The rapidly increasing availability of newborn screening data (1.5M cases in this study) enables the application of machine learning (ML) techniques to provide more accurate diagnoses of IMDs compared to simple cutoff values. We investigated two tasks in this study, i.e. complicated patterns between metabolites and better auxiliary diagnostic means. Experimental results show that novel metabolic patterns found in the study are effective and meaningful. Integrating ML techniques with these patterns improved predictive performance compared to existing diagnostic methods, suggesting ML techniques are becoming valuable as auxiliary diagnostic tools.